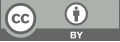
Research on the state prediction model of crowdfunding projects based on machine learning algorithms
- 1 Shanghai University Information Management and Information Systems
* Author to whom correspondence should be addressed.
Abstract
Crowdfunding is a concept that emerged due to difficulties in raising funds for community business projects, social activities, small and micro enterprises, and startups. However, the success or failure of crowdfunding projects is often full of uncertainty. Therefore, predicting whether a crowdfunding project can succeed has become a question worthy of in-depth research. This article takes the crowdfunding website platform Kickstarter as an example and uses machine learning methods to construct an effective crowdfunding project status prediction model. This study aims to compare the performance of different machine learning algorithms in predicting crowdfunding results, while identifying the main factors that affect crowdfunding results and their relative importance. The dataset used in this study includes data from all projects published on Kickstarter between January 2017 and January 2018. This study used six classic classification algorithms to predict the status of crowdfunding activities, and calculated the accuracy of each classification model. The results showed that the accuracy of all six models was close to 1, indicating that they could effectively predict the success or failure of crowdfunding projects. However, the Gaussian Naive Bayes model had slightly lower accuracy than the other five models. Furthermore, the research results indicate that in successful crowdfunding projects, factors such as crowdfunding goals, funds raised, and the number of supporters are more crucial influencing indicators than others.
Keywords
crowdfunding platform, classification prediction, logistic regression, decision tree
[1]. Tang, Z., & Xue, X. (2024). Research on user experience evaluation of public welfare crowdfunding platform based on perceived affordance theory. Journal of Library and Information Science, 9(2), 34-41, 50.
[2]. Lin, F., & Wen, X. (2023). Cultural and creative crowdfunding platform: types, potential and limitations. Industrial Innovation Research(15), 117-120.
[3]. Xu, L., & Yang, Z. (2023). Research on publishing integration ecology from the perspective of user innovation. Publishing Wide Angle(12), 62-67.
[4]. Wei, J., & Zhou, Z. (2024). Crowdfunding performance prediction model based on investor behavior analysis. Computer Application Research(2).
[5]. Xu, H. (2021). Research on the prediction model of online public welfare crowdfunding platform projects based on machine learning algorithm. Unpublished doctoral dissertation, Xidian University, Shaanxi, China.
[6]. Yang, J. (2023). Can artificial intelligence improve the investment efficiency of equity crowdfunding?—An empirical study based on the perspective of investor information perception. Times Finance(12), 57-60.
[7]. Shu, W., & Wang, M. (2024). Analysis of influencing factors of Internet crowdfunding results. Finance and Economics.
[8]. Xue, B. (2022). Research on influencing factors and prediction of online medical crowdfunding project financing results. Unpublished doctoral dissertation, Jiangxi University of Finance and Economics, Jiangxi, China.
Cite this article
YuanTianci,H. (2025). Research on the state prediction model of crowdfunding projects based on machine learning algorithms. Advances in Engineering Innovation,16(3),49-55.
Data availability
The datasets used and/or analyzed during the current study will be available from the authors upon reasonable request.
Disclaimer/Publisher's Note
The statements, opinions and data contained in all publications are solely those of the individual author(s) and contributor(s) and not of EWA Publishing and/or the editor(s). EWA Publishing and/or the editor(s) disclaim responsibility for any injury to people or property resulting from any ideas, methods, instructions or products referred to in the content.
About volume
Volume title:
© 2024 by the author(s). Licensee EWA Publishing, Oxford, UK. This article is an open access article distributed under the terms and
conditions of the Creative Commons Attribution (CC BY) license. Authors who
publish this series agree to the following terms:
1. Authors retain copyright and grant the series right of first publication with the work simultaneously licensed under a Creative Commons
Attribution License that allows others to share the work with an acknowledgment of the work's authorship and initial publication in this
series.
2. Authors are able to enter into separate, additional contractual arrangements for the non-exclusive distribution of the series's published
version of the work (e.g., post it to an institutional repository or publish it in a book), with an acknowledgment of its initial
publication in this series.
3. Authors are permitted and encouraged to post their work online (e.g., in institutional repositories or on their website) prior to and
during the submission process, as it can lead to productive exchanges, as well as earlier and greater citation of published work (See
Open access policy for details).