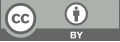
F-PointNet multi-modal 3D object detection based on RGB images and LiDAR point cloud
- 1 University of Bristol
* Author to whom correspondence should be addressed.
Abstract
This project explores the integration of image and point cloud data for 3D object detection using the F-PointNet model, aiming to enhance accuracy and reliability in autonomous driving applications. F-PointNet leverages multimodal data from RGB cameras and LiDAR to improve environmental perception and object localisation under varied operational conditions. Employing a rigorous methodology, the model incorporates preprocessing and network components such as frustum rotation and T-net adjustments to refine the detection process. Experiments were conducted on the KITTI dataset, which included applying both random and designated perturbations, and assessing their impact on the model’s performance. Results show that random perturbations generally outperform designated ones, especially in complex scenarios, by enhancing the model’s adaptability and capability for generalisation. This study highlights the critical role of methodological innovations and data perturbation strategies in advancing 3D object detection technologies, suggesting that further research is needed to optimise these approaches for broader applications. Furthermore, this research contributes to the development of autonomous systems, emphasising the importance of robust and accurate 3D object detection in enhancing the safety and reliability of autonomous vehicles.
Keywords
3D Object Detection, F-PointNet, Multimodal Data Fusion
[1]. Karangwa, J., Liu, J., & Zeng, Z. (2023). Vehicle Detection for Autonomous Driving: A Review of Algorithms and Datasets. IEEE Trans. Intell. Transp. Syst., vol. 24, no. 11, pp. 11568-11594.
[2]. Du, X., Ang, M. H., Karaman, S., & Rus, D. (2018). A General Pipeline for 3D Detection of Vehicles. Proc. IEEE Int. Conf. Robot. Autom., Brisbane, QLD, Australia, pp. 3194-3200, doi: 10.1109/ICRA.2018.8461232.
[3]. Oksuz, K., Cam, B. C., Kalkan, S., & Akbas, E. (2021). Imbalance Problems in Object Detection: A Review. IEEE Trans. Pattern Anal. Mach. Intell., vol. 43, no. 10, pp. 3388-3415, doi: 10.1109/TPAMI.2020.2981890.
[4]. Guo, X., Ji, Z., Feng, Q., Wang, H., Yang, Y., & Li, Z. (2023). URS: A Light-Weight Segmentation Model for Train Wheelset Monitoring. IEEE Trans. Intell. Transp. Syst., vol. 24, no. 7, pp. 7707-7716.
[5]. SAE International (2018). Taxonomy and definitions for terms related to driving automation systems for on-road motor vehicles. SAE Int., vol. 4970, no. 724, pp. 1-5.
[6]. Li, Z., Du, Y., Zhu, M., Zhou, S., & Zhang, L. (2022). A survey of 3D object detection algorithms for intelligent vehicles development. Artif. Life Robot., vol. 27, pp. 1-8.
[7]. Xu, B., & Chen, Z. (2018). Multi-level fusion based 3d object detection from monocular images. Proc. IEEE Conf. Comp. Vis. Pattern Recognit., pp. 2345-2353.
[8]. Wang, Y., Guizilini, V. C., Zhang, T., Wang, Y., Zhao, H., & Solomon, J. (2022). Detr3d: 3d object detection from multi-view images via 3d-to-2d queries. Conf. Robot Learn., pp. 180-191. PMLR.
[9]. Zhou, Y., & Tuzel, O. (2018). Voxelnet: End-to-end learning for point cloud based 3d object detection. Proc. IEEE Conf. Comput. Vis. Pattern Recognit., pp. 4490-4499.
[10]. Zhao, K. et al. (2022). 3D Vehicle Detection Using Multi-Level Fusion From Point Clouds and Images. IEEE Trans. Intell. Transp. Syst., vol. 23, no. 9, pp. 15146-15154, doi: 10.1109/TITS.2021.3137392.
[11]. Qi, C. R. et al. (2017). Pointnet: Deep learning on point sets for 3d classification and segmentation. Proc. IEEE Conf. Comput. Vis. Pattern Recognit.
[12]. Qi, C. R. et al. (2017). Pointnet++: Deep hierarchical feature learning on point sets in a metric space. Advances in Neural Inf. Process. Syst., vol. 30.
[13]. Li, Y. et al. (2018). Pointcnn: Convolution on x-transformed points. Advances in Neural Inf. Process. Syst., vol. 31.
[14]. Fan, L., Xiong, X., Wang, F., Wang, N., & Zhang, Z. (2021). Rangedet: In defense of range view for lidar-based 3d object detection. Proc. IEEE/CVF Int. Conf. Comp. Vis., pp. 2918-2927.
[15]. Beltrán, J., Guindel, C., Moreno, F. M., Cruzado, D., Garcia, F., & De La Escalera, A. (2018). Birdnet: a 3d object detection framework from lidar information. 2018 21st Int. Conf. Intel. Trans. Sys. (ITSC), pp. 3517-3523. IEEE.
[16]. Li, B. (2017). 3d fully convolutional network for vehicle detection in point cloud. 2017 IEEE/RSJ Int. Conf. Intell. Robots Sys. (IROS), pp. 1513-1518. IEEE.
[17]. Chen, X., Wan, J., Li, B., & Xia, T. (2017). Multi-view 3D Object Detection Network for Autonomous Driving. IEEE. https://doi.org/10.1109/cvpr.2017.691
[18]. Qi, C. R. et al. (2020). Imvotenet: Boosting 3d object detection in point clouds with image votes. Proc. IEEE/CVF Conf. Comput. Vis. Pattern Recognit., pp. 4404-4413.
[19]. Qi, C. R. et al. (2018). Frustum pointnets for 3D object detection from rgb-d data. Proc. IEEE Conf. Comput. Vis. Pattern Recognit., pp. 918-927.
[20]. Wang, Z., & Jia, K. (2019). Frustum ConvNet: Sliding frustums to aggregate local point-wise features for amodal 3D object detection. Proc. IEEE/RSJ Int. Conf. Intell. Robot. Syst. (IROS), pp. 1742-1749.
[21]. Chen, C., Fragonara, L. Z., & Tsourdos, A. (2021). RoIFusion: 3D object detection from LiDAR and vision. IEEE Access, 9, 51710-51721.
[22]. Yu, S. L., Westfechtel, T., Hamada, R., Ohno, K., & Tadokoro, S. (2017). Vehicle detection and localization on bird’s eye view elevation images using convolutional neural network. 2017 IEEE Int. Sym. Safety, Sec. Resc. Robot. (SSRR), pp. 102-109. IEEE.
[23]. Yan, Y., Mao, Y., & Li, B. (2018). SECOND: Sparsely embedded convolutional detection. Sensors, vol. 18, no. 10, pp. 3337.
[24]. Zou, Z., Chen, K., Shi, Z., Guo, Y., & Ye, J. (2023). Object detection in 20 years: A survey. Proc. IEEE, 111(3), 257-276.
[25]. Lin, T. Y., Dollár, P., Girshick, R., He, K., Hariharan, B., & Belongie, S. (2017). Feature pyramid networks for object detection. Proc. IEEE Conf. Comput. Vis. Pattern Recognit., pp. 2117-2125.
[26]. Lin, T. Y., Dollár, P., Girshick, R., He, K., Hariharan, B., & Belongie, S. (2017). Feature pyramid networks for object detection. Proc. IEEE Conf. Comput. Vis. Pattern Recognit., pp. 2117-2125.
[27]. Kossaifi, J., Bulat, A., Tzimiropoulos, G., & Pantic, M. (2019). T-net: Parametrizing fully convolutional nets with a single high-order tensor. Proc. of the IEEE/CVF Conf. Comp. Vis. Pattern Recognit., pp. 7822-7831.
Cite this article
Luo,Y. (2024). F-PointNet multi-modal 3D object detection based on RGB images and LiDAR point cloud. Advances in Engineering Innovation,12,77-85.
Data availability
The datasets used and/or analyzed during the current study will be available from the authors upon reasonable request.
Disclaimer/Publisher's Note
The statements, opinions and data contained in all publications are solely those of the individual author(s) and contributor(s) and not of EWA Publishing and/or the editor(s). EWA Publishing and/or the editor(s) disclaim responsibility for any injury to people or property resulting from any ideas, methods, instructions or products referred to in the content.
About volume
Journal:Advances in Engineering Innovation
© 2024 by the author(s). Licensee EWA Publishing, Oxford, UK. This article is an open access article distributed under the terms and
conditions of the Creative Commons Attribution (CC BY) license. Authors who
publish this series agree to the following terms:
1. Authors retain copyright and grant the series right of first publication with the work simultaneously licensed under a Creative Commons
Attribution License that allows others to share the work with an acknowledgment of the work's authorship and initial publication in this
series.
2. Authors are able to enter into separate, additional contractual arrangements for the non-exclusive distribution of the series's published
version of the work (e.g., post it to an institutional repository or publish it in a book), with an acknowledgment of its initial
publication in this series.
3. Authors are permitted and encouraged to post their work online (e.g., in institutional repositories or on their website) prior to and
during the submission process, as it can lead to productive exchanges, as well as earlier and greater citation of published work (See
Open access policy for details).