About AEIAdvances in Engineering Innovation (AEI) is a peer-reviewed, fast-indexing open access journal hosted by Tianjin University Research Centre on Data Intelligence and Cloud-Edge-Client Service Engineering and published by EWA Publishing. AEI is published irregularly, and it is a comprehensive journal focusing on multidisciplinary areas of engineering and at the interface of related subjects, including, but not limited to, Artificial Intelligence, Biomedical Engineering, Electrical and Electronic Engineering, Materials Engineering, Traffic and Transportation Engineering, etc.For the details about the AEI scope, please refer to the Aims and Scope page. For more information about the journal, please refer to the FAQ page or contact info@ewapublishing.org. |
Aims & scope of AEI are: · Artificial Intelligence · Computer Sciences · Aerospace Engineering · Architecture & Civil Engineering · Biomedical Engineering · Electrical and Electronic Engineering · Energy and Power Engineering · Materials Engineering · Mechanical Engineering · Traffic and Transportation Engineering |
Article processing charge
A one-time Article Processing Charge (APC) of 450 USD (US Dollars) applies to papers accepted after peer review. excluding taxes.
Open access policy
This is an open access journal which means that all content is freely available without charge to the user or his/her institution. (CC BY 4.0 license).
Your rights
These licenses afford authors copyright while enabling the public to reuse and adapt the content.
Peer-review process
Our blind and multi-reviewer process ensures that all articles are rigorously evaluated based on their intellectual merit and contribution to the field.
Editors View full editorial board
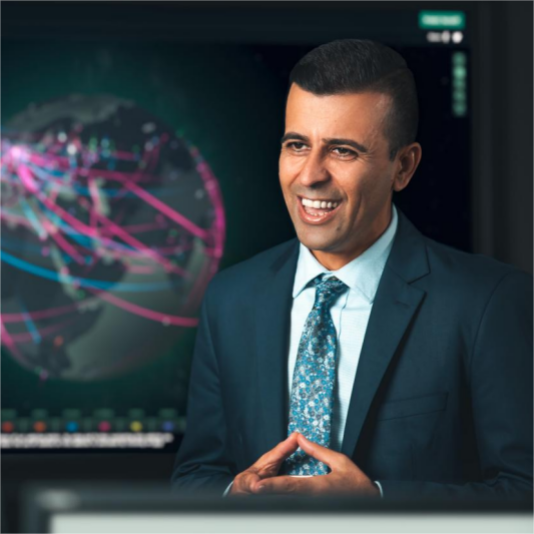
Chicago, US
momar3@iit.edu
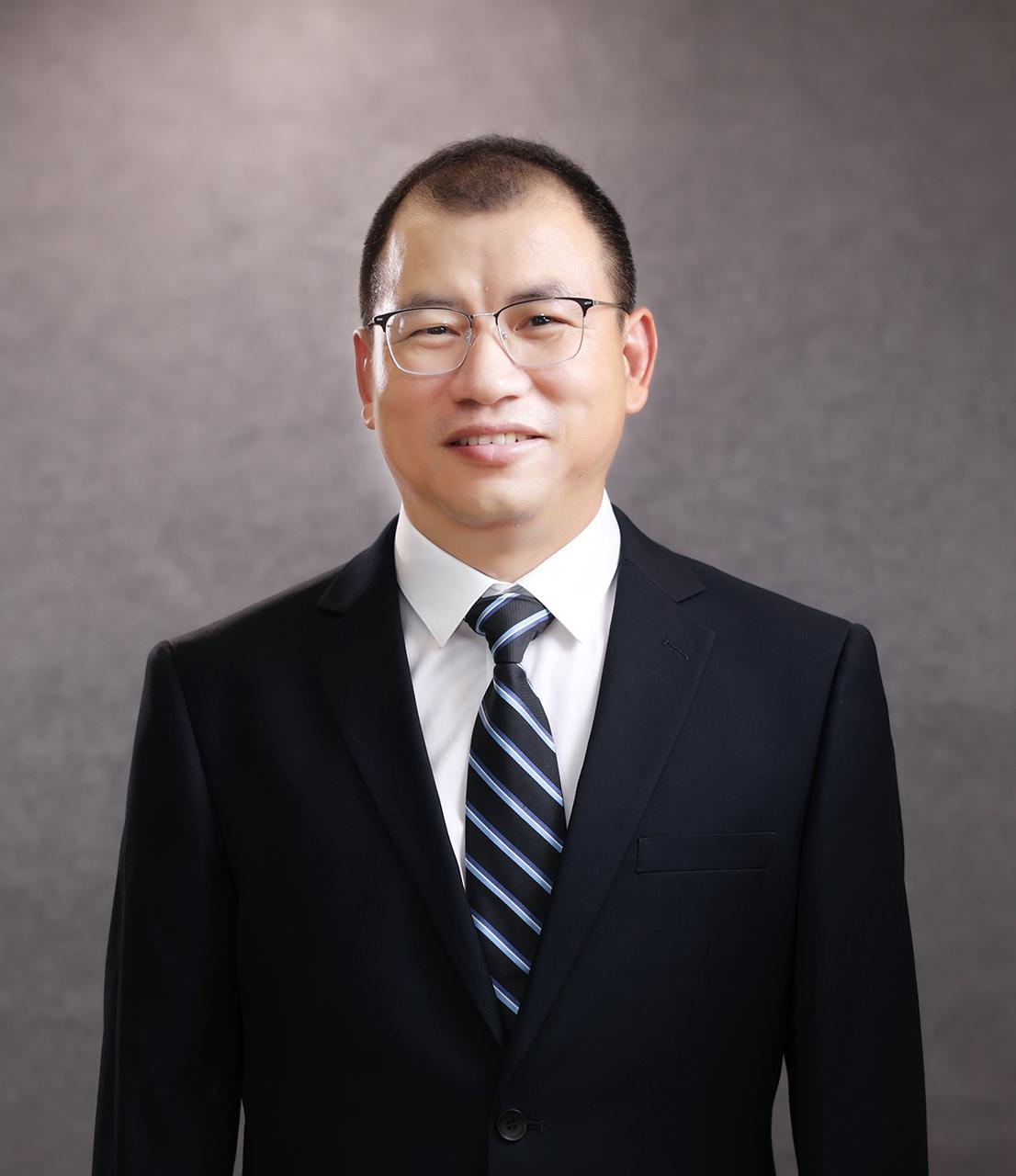
Tianjin, China
rgz@tju.edu.cn
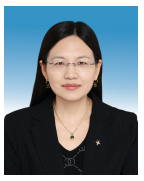
Tianjin, China
zhangli2006@tust.edu.cn
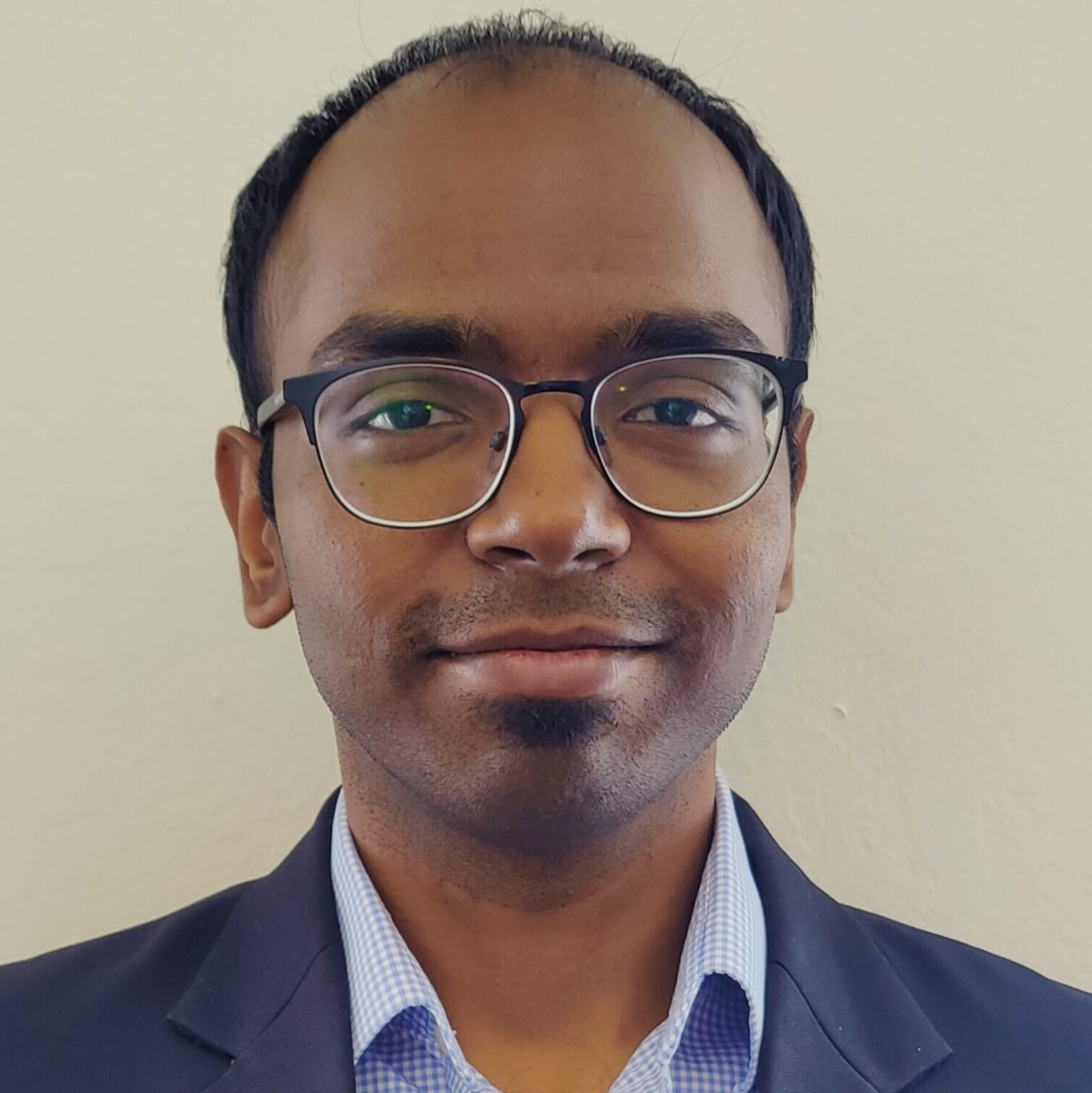
Boston, US
rkpaul@bu.edu
Latest articles View all articles
This study thoroughly investigates how civil engineering management can effectively control project costs. Through analyzing key cost control strategies within civil engineering management, the research reveals the significance of economic principles and rational resource utilization. Using a case study approach, diagnostic analysis of actual engineering projects was conducted, validating the effectiveness of measures such as detailed budgeting, strengthened cost accounting, and enhanced cost management. The findings suggest that implementing scientifically sound civil engineering management can significantly reduce project costs and enhance project efficiency. Additionally, the study recommends reforming traditional management approaches and integrating modern management concepts and methodologies, further optimizing cost control in civil engineering projects. The results offer essential reference value for improving cost management, increasing project benefits, and promoting long-term civil engineering development.
Kunshan, a typical river network city located in the Taihu Plain, has a dense network of rivers, with upstream receiving discharge from rivers and downstream supported by multiple tributaries entering the Yangtze River. Additionally, the city’s internal embanked areas are numerous. Furthermore, the existing information system was constructed prematurely and lacks professional forecasting and simulation technologies, making it difficult to fully grasp the current water security situation and rapidly display the effects of scheduling plans. This study analyzes the “Four-Preparation” integration and proposes improvements. Based on Kunshan’s current situation, the goal is to construct an Integrated Four-Preparation System, establishing a scientific coupling system for forecasting, early warning, rehearsal, and contingency planning. This will strengthen intelligent and smart development applications to achieve scientific disaster reduction.
To improve the accuracy of photovoltaic power prediction, a combined prediction model integrating multiple algorithms is proposed. First, key factors are selected, and the Gaussian Mixture Model (GMM) is used to cluster and generate historical days with high correlation to the forecast day. Then, a photovoltaic power prediction model based on Variational Mode Decomposition (VMD) and an improved Grey Wolf Optimizer Kernel Extreme Learning Machine (MSI-GWO-KELM) is constructed. Finally, actual data from a photovoltaic power station in Australia is used as a case study. The results show that the VMD-MSI-GWO-KELM model is suitable for different weather conditions and offers better prediction accuracy than other models.
With the acceleration of urbanization, flood control and drainage management in cities are facing increasing challenges. As a nationally recognized model for effective flood control, Suzhou Industrial Park has experienced frequent extreme weather events in recent years, highlighting the urgent need for informatization in flood control and drainage management. This paper provides a detailed introduction to the design scheme of the flood control and drainage decision-making command information system for Suzhou Industrial Park, covering key aspects such as system requirement analysis, overall architecture, core technologies, and risk management. By integrating multi-source data, including meteorology, hydrology, waterlogging points, and the sewage pipeline network, the system establishes a comprehensive flood control and drainage decision-making platform featuring situational awareness, monitoring and early warning, and command and dispatching functions. The system aims to enhance the flood control and drainage capabilities of the park, ensure urban safety, and provide a reference for the informatization of flood management in other cities.
Volumes View all volumes
2025
Volume 16April 2025
Find articlesVolume 16March 2025
Find articlesVolume 16March 2025
Find articles2024
Volume 14December 2024
Find articlesVolume 13November 2024
Find articlesVolume 12October 2024
Find articlesAnnouncements View all announcements
Advances in Engineering Innovation
We pledge to our journal community:
We're committed: we put diversity and inclusion at the heart of our activities...
Advances in Engineering Innovation
The statements, opinions and data contained in the journal Advances in Engineering Innovation (AEI) are solely those of the individual authors and contributors...
Indexing
The published articles will be submitted to following databases below:
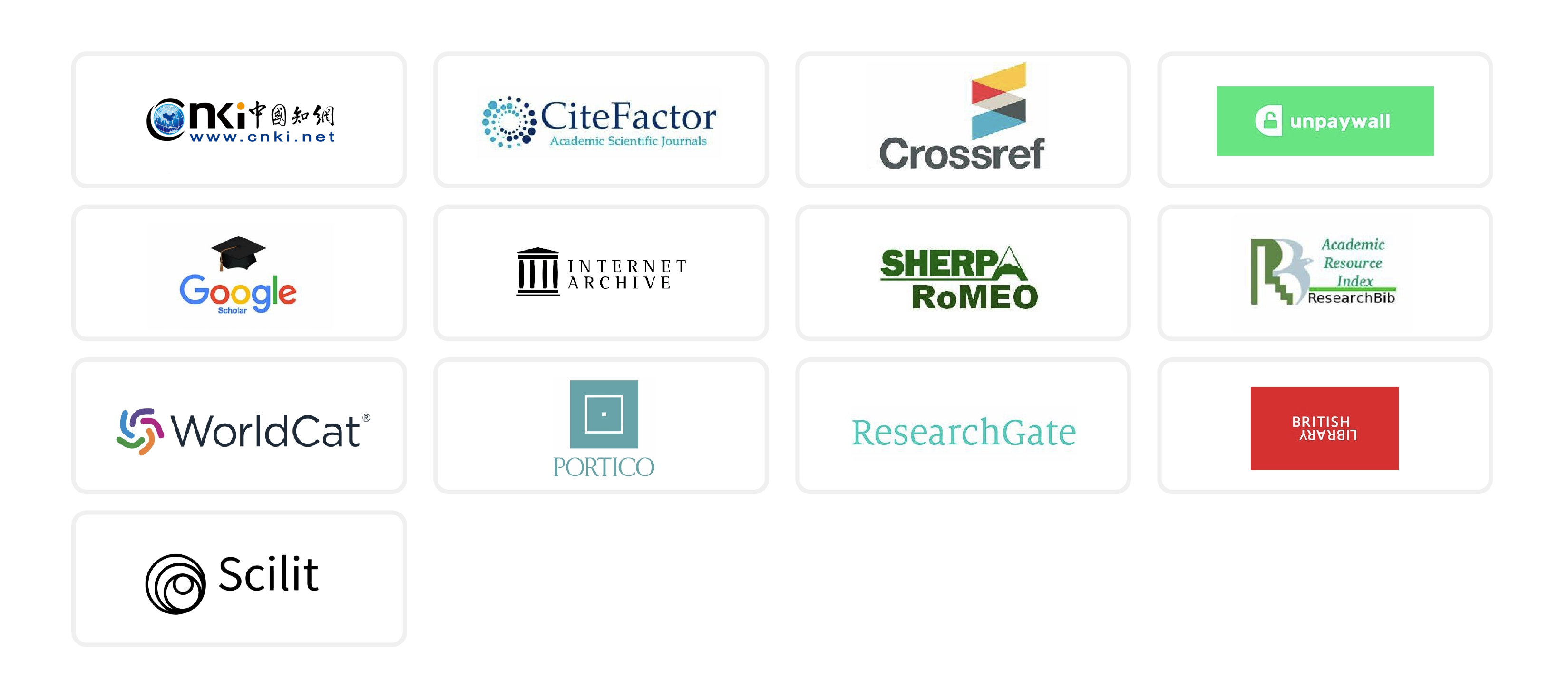