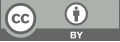
Integrating advanced principal component analysis into naive bayes for enhanced classification performance
- 1 University of Kent
- 2 University of Ottawa
* Author to whom correspondence should be addressed.
Abstract
The Naive Bayes algorithm is one of the most important and popular algorithms in machine learning and data mining, not only because of its simplicity but also because of its superior classification performance. The central assumption of this algorithm is known as the attribute independence assumption. This assumption allows the Naive Bayes algorithm to solve classification problems conveniently, but also limits the performance of this algorithm to a certain extent when the mixed type of variables exist in its input dataset. Recently, we proposed an improved Naive Bayes classification algorithm by combining an improved Principal Component Analysis (PCA) method. The improved PCA first calculates correlation coefficients between coupling variables using the Pearson and Kendall coefficients, where the two types of coefficients are calculated separately for quantitative and qualitative data. After coupling data is transformed into principal components, those correlated variables can be integrated into the improved Naive Bayes algorithm. When the improved Naive Bayes algorithm is applied to a classified task, it is easy to verify that the transformed principal components data are approximately independent, thereby conforming to the Naive Bayes independence assumption to a relatively greater extent. This implies that it is likely for the improved Naive Bayes algorithm to yield a more accurate classification performance, as it is more robust to the presence of noise in classification instances.
Keywords
naive bayes, principal component analysis, classification, Pearson Coefficient, Kendall Coefficient
[1]. Mushtaq, Z., et al. (2023). Effective kernel‐principal component analysis based approach for Wisconsin breast cancer diagnosis. Electronics Letters, 59(2), e212706.
[2]. Yesilkaya, B., et al. (2023). Principal component analysis and manifold learning techniques for the design of brain-computer interfaces based on steady-state visually evoked potentials. Journal of Computational Science, 68, 102000.
[3]. Caplar, R., & Kulisic, P. (1973). Proc. Int. Conf. on Nuclear Physics (Munich), Vol. 1, 517. Amsterdam: North-Holland/American Elsevier.
[4]. Szytula, A., & Leciejewicz, J. (1989). Handbook on the Physics and Chemistry of Rare Earths, Vol. 12 (K. A. Gschneidner Jr & L. Erwin, Eds.), 133. Amsterdam: Elsevier.
[5]. Kuhn, T. (1998). Density matrix theory of coherent ultrafast dynamics. In Theory of Transport Properties of Semiconductor Nanostructures (Electronic Materials Vol. 4, E. Schöll, Ed.), 173–214. London: Chapman and Hall.
[6]. Magdacy Jerjes, A., Zeki Ablahd, N., Yousif Dawod, A., & Fakhrulddin Abdulqader, M. (2023). Detect malicious web pages using naive Bayesian algorithm to detect cyber threats. Wireless Personal Communications, 1-13.
[7]. Zhang, H., Jiang, L., & Webb, G. I. (2023). Rigorous non-disjoint discretization for naive Bayes. Pattern Recognition, 140, 109554.
[8]. Talaei Khoei, T., & Kaabouch, N. (2023). A comparative analysis of supervised and unsupervised models for detecting attacks on intrusion detection systems. Information, 14(2), 103.
[9]. Dhiman, R. (2023). Electroencephalogram channel selection based on Pearson correlation coefficient for motor imagery-brain-computer interface. Measurement: Sensors, 25, 100616.
[10]. Chicco, D., & Jurman, G. (2023). The Matthews correlation coefficient (MCC) should replace the ROC AUC as the standard metric for assessing binary classification. BioData Mining, 16(1), 4.
Cite this article
Luo,L.;Liu,T. (2024). Integrating advanced principal component analysis into naive bayes for enhanced classification performance. Advances in Operation Research and Production Management,3,27-31.
Data availability
The datasets used and/or analyzed during the current study will be available from the authors upon reasonable request.
Disclaimer/Publisher's Note
The statements, opinions and data contained in all publications are solely those of the individual author(s) and contributor(s) and not of EWA Publishing and/or the editor(s). EWA Publishing and/or the editor(s) disclaim responsibility for any injury to people or property resulting from any ideas, methods, instructions or products referred to in the content.
About volume
Journal:Advances in Operation Research and Production Management
© 2024 by the author(s). Licensee EWA Publishing, Oxford, UK. This article is an open access article distributed under the terms and
conditions of the Creative Commons Attribution (CC BY) license. Authors who
publish this series agree to the following terms:
1. Authors retain copyright and grant the series right of first publication with the work simultaneously licensed under a Creative Commons
Attribution License that allows others to share the work with an acknowledgment of the work's authorship and initial publication in this
series.
2. Authors are able to enter into separate, additional contractual arrangements for the non-exclusive distribution of the series's published
version of the work (e.g., post it to an institutional repository or publish it in a book), with an acknowledgment of its initial
publication in this series.
3. Authors are permitted and encouraged to post their work online (e.g., in institutional repositories or on their website) prior to and
during the submission process, as it can lead to productive exchanges, as well as earlier and greater citation of published work (See
Open access policy for details).