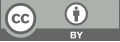
Use Machine Learning to Forecast Economic Recession with Covid-19 Evidence
- 1 Univeristy of Illinois at Urbana Champaign
* Author to whom correspondence should be addressed.
Abstract
This article starts by studying the impact of the COVID-19 pandemic on the United States economy and then delves into the relationship between the pandemic and the economic mobility in U.S. In addition, this article also uses various models to predict the US economy, including vector autoregressive model, support vector machines, eXtreme gradient boosting, light gradient-boosting machine and the long short-term memory network, then comparing the prediction results of the above models to select the relatively optimal model. To test the model, the model is also used to predict Italy's economy and then XGBoost is selected. The limitations of the model will be proposed based on the predicted results. Based on the research, XGBoost model can be applied to forecast economic recession. With further study, we believe such forecast and our understanding of economic processes and economic recession can be improved, and the government can adopt proper policies to alleviate the economic recession.
Keywords
COVID-19, economic forecast, machine learning
[1]. Carter, P., Anderson, M., & Mossialos, E. (2020). Health system, public health, and economic implications of managing COVID-19 from a cardiovascular perspective.
[2]. Guerrieri, V., Lorenzoni, G., Straub, L., & Werning, I. (2022). Macroeconomic implications of COVID-19: Can negative supply shocks cause demand shortages?. American Economic Review, 112(5), 1437-1474.
[3]. Chetty, R., Friedman, J. N., & Stepner, M. (2020). The economic impacts of COVID-19: Evidence from a new public database built using private sector data (No. w27431). National Bureau of Economic Research.
[4]. Chaney, S., & Morath, E. (2020). Record 6.6 million Americans sought unemployment benefits last week. Wall Street Journal,3.
[5]. Baker, S. R., Bloom, N., Davis, S. J., & Terry, S. J. (2020). Covid-induced economic uncertainty (No. w26983). National Bureau of Economic Research.
[6]. Lau, H., Khosrawipour, V., Kocbach, P., Mikolajczyk, A., Schubert, J., Bania, J., & Khosrawipour, T. (2020). The positive impact of lockdown in Wuhan on containing the COVID-19 outbreak in China. Journal of travel medicine, 27(3), taaa037.
[7]. Mendolia, S., Stavrunova, O., & Yerokhin, O. (2021). Determinants of the community mobility during the COVID-19 epidemic: The role of government regulations and information. Journal of Economic Behavior & Organization, 184, 199-231.
[8]. Bonaccorsi, G., Pierri, F., Cinelli, M., Flori, A., Galeazzi, A., Porcelli, F., ... & Pammolli, F. (2020). Economic and social consequences of human mobility restrictions under COVID-19. Proceedings of the National Academy of Sciences, 117(27), 15530-15535.
[9]. Prawoto, N., Priyo Purnomo, E., & Az Zahra, A. (2020). The impacts of Covid-19 pandemic on socio-economic mobility in Indonesia.
[10]. Thorbecke, W. (2020). The impact of the COVID-19 pandemic on the US economy: Evidence from the stock market. Journal of Risk and Financial Management, 13(10), 233.
[11]. Maliszewska, M., Mattoo, A., & Van Der Mensbrugghe, D. (2020). The potential impact of COVID-19 on GDP and trade: A preliminary assessment. World Bank policy research working paper, (9211).
[12]. Alsolami, F. J., ALGhamdi, A. S. A. M., Khan, A. I., Abushark, Y. B., Almalawi, A., Saleem, F., ... & Khan, R. A. (2021). Impact assessment of COVID-19 pandemic through machine learning models. Comput. Mater. Contin, 68, 2895-2912.
[13]. Ludvigson, S. C., Ma, S., & Ng, S. (2020). COVID-19 and the macroeconomic effects of costly disasters (No. w26987). National Bureau of Economic Research.
[14]. Primiceri, G. E., & Tambalotti, A. (2020). Macroeconomic Forecasting in the Time of COVID-19. Manuscript, Northwestern University, 1-23.
[15]. Sims, C. A. (1980). Macroeconomics and reality. Econometrica: journal of the Econometric Society, 1-48.
[16]. Guyon, I., & Elisseeff, A. (2006). An introduction to feature extraction. In Feature extraction: foundations and applications (pp. 1-25). Berlin, Heidelberg: Springer Berlin Heidelberg.
[17]. Boser, B. E., Guyon, I. M., & Vapnik, V. N. (1992, July). A training algorithm for optimal margin classifiers. In Proceedings of the fifth annual workshop on Computational learning theory (pp. 144-152).
[18]. Aizerman, A. (1964). Theoretical foundations of the potential function method in pattern recognition learning. Automation and remote control, 25, 821-837.
[19]. Brownlee, J. (2020). Gradient boosting with scikit-learn, xgboost, lightgbm, and catboost. Machine Learning Mastery.
[20]. Memory, L. S. T. (2010). Long short-term memory. Neural computation, 9(8), 1735-1780.
[21]. Klein, L. R. (Ed.). (1991). Comparative performance of US econometric models. Oxford University Press, USA.
[22]. Fildes, R., & Stekler, H. (2002). The state of macroeconomic forecasting. Journal of macroeconomics, 24(4), 435-468.
Cite this article
Xu,N. (2024). Use Machine Learning to Forecast Economic Recession with Covid-19 Evidence. Journal of Applied Economics and Policy Studies,4,34-43.
Data availability
The datasets used and/or analyzed during the current study will be available from the authors upon reasonable request.
Disclaimer/Publisher's Note
The statements, opinions and data contained in all publications are solely those of the individual author(s) and contributor(s) and not of EWA Publishing and/or the editor(s). EWA Publishing and/or the editor(s) disclaim responsibility for any injury to people or property resulting from any ideas, methods, instructions or products referred to in the content.
About volume
Journal:Journal of Applied Economics and Policy Studies
© 2024 by the author(s). Licensee EWA Publishing, Oxford, UK. This article is an open access article distributed under the terms and
conditions of the Creative Commons Attribution (CC BY) license. Authors who
publish this series agree to the following terms:
1. Authors retain copyright and grant the series right of first publication with the work simultaneously licensed under a Creative Commons
Attribution License that allows others to share the work with an acknowledgment of the work's authorship and initial publication in this
series.
2. Authors are able to enter into separate, additional contractual arrangements for the non-exclusive distribution of the series's published
version of the work (e.g., post it to an institutional repository or publish it in a book), with an acknowledgment of its initial
publication in this series.
3. Authors are permitted and encouraged to post their work online (e.g., in institutional repositories or on their website) prior to and
during the submission process, as it can lead to productive exchanges, as well as earlier and greater citation of published work (See
Open access policy for details).