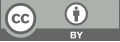
Three-Dimensional Synergies: The Impact of Intelligent Investment, Industrial Environment, and Human Capital on the Employment Structure of High-Tech Manufacturing
- 1 Beijing University of Posts and Telecommunications
- 2 Zhejiang University of Finance & Economics
* Author to whom correspondence should be addressed.
Abstract
This study investigates the impact pathway and mechanisms of multi-factor artificial intelligence investment on the proportion of high-tech manufacturing labor force, drawing upon the theory of skill-biased technological changes (SBTC). Employing a mixed qualitative and quantitative research design, we identify key resource factors influencing changes in the employment structure within the high-tech manufacturing industry. Our analysis employs fuzzy-set qualitative comparative analysis (fsQCA) on a provincial dataset from China, spanning the years 2018 to 2020. Findings reveal the following insights: (1) There exists a configurational effect among antecedent factors influencing changes in the employment structure of the manufacturing industry. Specifically, investments in AI-related industries, industrial robots, industry transformation and upgrading, industrial intelligence, working-age population proportion, and educational attainment of the workforce are not individually necessary conditions. (2) There are three paths that drive the increase of the proportion of high-tech manufacturing labor force, including comprehensive development type, capital-led type, and education-led type. (3) Investments in industrial robots and advancements in industrial intelligence emerge as pivotal factors driving the increase in the proportion of high-tech manufacturing labor force. This research contributes to advancing a multidimensional perspective on biased technological change, laying the groundwork for regional management strategies to invest in AI for fostering the healthy development of high-tech manufacturing.
Keywords
technological progress, artificial intelligence, high-tech manufacturing, employment structure, configurational effects, fsQCA
[1]. Hu, W., Fang, J., Zhang, T., et al. (2023). A new quantitative digital twin maturity model for high-end equipment. Journal of Manufacturing Systems, 66, 248-259.
[2]. Yu, T. T., Rong, A., & Hao, F. L. (2022). Avoiding the middle-income trap: The spatial-temporal effects of human capital on regional economic growth in Northeast China. Growth and Change, 53(2), 536-558.
[3]. Su, Z., Togay, G., & Côté, A. (2021). Artificial intelligence: A destructive and yet creative force in the skilled labour market. Human Resource Development International, 24(3), 341-352.
[4]. Frey, C. B., & Osborne, M. A. (2017). The future of employment: How susceptible are jobs to computerisation? Technological Forecasting and Social Change, 114, 254-280.
[5]. Acemoglu, D., & Restrepo, P. (2020). Robots and jobs: Evidence from US labor markets. Journal of Political Economy, 128(6), 2188-2244.
[6]. Caselli, M. (2014). Trade, skill-biased technical change and wages in Mexican manufacturing. Applied Economics, 46(3), 336-348.
[7]. Lewis, T. (1996). Studying the impact of technology on work and jobs. Journal of Industrial Teacher Education, 3(33), 44-65.
[8]. Autor, D. H., Levy, F., & Murnane, R. J. (2003). The skill content of recent technological change: An empirical exploration. The Quarterly Journal of Economics, 118(4), 1279-1333.
[9]. Acemoglu, D. (2002). Technical change, inequality, and the labor market. Journal of Economic Literature, 40(1), 7-72.
[10]. Frank, M. R., Autor, D., Bessen, J. E., et al. (2019). Toward understanding the impact of artificial intelligence on labor. Proceedings of the National Academy of Sciences, 116(14), 6531-6539.
[11]. Deming, D. J. (2017). The growing importance of social skills in the labor market. The Quarterly Journal of Economics, 132(4), 1593-1640.
[12]. Arntz, M., Gregory, T., & Zierahn, U. (2017). Revisiting the risk of automation. Economics Letters, 159, 157-160.
[13]. David, B. (2017). Computer technology and probable job destructions in Japan: An evaluation. Journal of the Japanese and International Economies, 43, 77-87.
[14]. Acemoglu, D., & Restrepo, P. (2018). The race between man and machine: Implications of technology for growth, factor shares, and employment. The American Economic Review, 108(6), 1488-1542.
[15]. Böhm, M. J., & Siegel, C. (2021). Make yourselves scarce: The effect of demographic change on the relative wages and employment rates of experienced workers. International Economic Review (Philadelphia), 62(4), 1537-1568.
[16]. Mao, R., Xu, J., & Zou, J. (2018). The labor force age structure and employment structure of the modern sector. China Economic Review, 52, 1-15.
[17]. Wu, B., & Yang, W. (2022). Empirical test of the impact of the digital economy on China's employment structure. Finance Research Letters, 49, 103047.
[18]. Autor, D. H. (2015). Why are there still so many jobs? The history and future of workplace automation. Journal of Economic Perspectives, 29(3), 3-30.
[19]. Acemoglu, D., & Restrepo, P. (2019). Automation and new tasks: How technology displaces and reinstates labor. Journal of Economic Perspectives, 33(2), 3-29.
[20]. Ma, H., Gao, Q., Li, X., et al. (2022). AI development and employment skill structure: A case study of China. Economic Analysis and Policy, 73, 242-254.
[21]. Gu, T., Zhang, S., & Cai, R. (2022). Can artificial intelligence boost employment in service industries? Empirical analysis based on China. Applied Artificial Intelligence, 36(1), 1078-1097.
[22]. Fiss, P. C. (2011). Building better causal theories: A fuzzy set approach to typologies in organization research. Academy of Management Journal, 54(2), 393-420.
[23]. Borland, J., & Coelli, M. (2017). Are robots taking our jobs? Australian Economic Review, 50(4), 377-397.
[24]. Schneider, C. Q., & Wagemann, C. (2012). Set-theoretic methods for the social sciences: A guide to qualitative comparative analysis. Cambridge University Press.
[25]. Gomółka, K., & Flisikowski, K. (2020). Changes in the structure of Armenia's labour resources between 1993 and 2020. Economic Annals-XXI, 185(9-10), 155-166.
Cite this article
Guo,J.;Nie,W. (2024). Three-Dimensional Synergies: The Impact of Intelligent Investment, Industrial Environment, and Human Capital on the Employment Structure of High-Tech Manufacturing. Journal of Applied Economics and Policy Studies,6,1-11.
Data availability
The datasets used and/or analyzed during the current study will be available from the authors upon reasonable request.
Disclaimer/Publisher's Note
The statements, opinions and data contained in all publications are solely those of the individual author(s) and contributor(s) and not of EWA Publishing and/or the editor(s). EWA Publishing and/or the editor(s) disclaim responsibility for any injury to people or property resulting from any ideas, methods, instructions or products referred to in the content.
About volume
Journal:Journal of Applied Economics and Policy Studies
© 2024 by the author(s). Licensee EWA Publishing, Oxford, UK. This article is an open access article distributed under the terms and
conditions of the Creative Commons Attribution (CC BY) license. Authors who
publish this series agree to the following terms:
1. Authors retain copyright and grant the series right of first publication with the work simultaneously licensed under a Creative Commons
Attribution License that allows others to share the work with an acknowledgment of the work's authorship and initial publication in this
series.
2. Authors are able to enter into separate, additional contractual arrangements for the non-exclusive distribution of the series's published
version of the work (e.g., post it to an institutional repository or publish it in a book), with an acknowledgment of its initial
publication in this series.
3. Authors are permitted and encouraged to post their work online (e.g., in institutional repositories or on their website) prior to and
during the submission process, as it can lead to productive exchanges, as well as earlier and greater citation of published work (See
Open access policy for details).