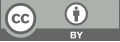
Mind meets machine: how do humans and AI assistants co-create knowledge?
- 1 Beijing University of Posts and Telecommunications, Beijing, China
- 2 Beijing University of Posts and Telecommunications, Beijing, China
- 3 Beijing University of Posts and Telecommunications, Beijing, China
* Author to whom correspondence should be addressed.
Abstract
The emergence of large language models has catalyzed unprecedented interest in artificial intelligence applications. Intelligent assistants, as exemplary implementations of this technology, have demonstrated remarkable capabilities, leading to their increasing adoption as a primary mode of human-machine interaction for task completion. However, the underlying mechanisms governing user interactions with these intelligent assistants warrant further investigation. This study examines the human-machine interaction process through the lens of knowledge co-creation, grounded in sense-making theory. We developed an intelligent assistant platform to collect interaction data from 316 users and conducted grounded theory research, incorporating third-party materials, to construct a comprehensive model of human-machine collaborative knowledge co-creation. The model was subsequently validated through quantitative empirical research. Our findings reveal three key insights: (1) human-machine collaboration serves as a critical mediator in facilitating knowledge co-creation; (2) both individual and machine knowledge capabilities significantly contribute to achieving optimal human-machine synergy; and (3) users' prompt literacy exhibits a significant positive moderating effect on human-machine collaboration. This research makes two primary contributions: it elucidates the fundamental mechanisms underlying human-intelligent assistant interaction from a knowledge co-creation perspective, and it establishes a theoretical foundation for scaling human-machine knowledge co-creation research to organizational and industrial contexts.
Keywords
human-machine collaboration, knowledge co-creation, intelligent assistant, large language model, sense-making
[1]. Jarrahi, M. H., Askay, D., Eshraghi, A., & Smith, P. (2023). Artificial intelligence and knowledge management: A partnership between human and AI. Business Horizons, 66(1), 87–99. https://doi.org/10.1016/j.bushor.2022.03.002
[2]. Sharma, A. (2023). Artificial intelligence for sense making in survival supply chains. International Journal of Production Research, 0(0), 1–24. https://doi.org/10.1080/00207543.2023.2221743
[3]. Feuerriegel, S., Hartmann, J., Janiesch, C., & Zschech, P. (2024). Generative AI. Business & Information Systems Engineering, 66(1), 111–126. https://doi.org/10.1007/s12599-023-00834-7
[4]. Stokel-Walker, C., & Van Noorden, R. (2023). What ChatGPT and generative AI mean for science. Nature, 614(7947), 214–216. https://doi.org/10.1038/d41586-023-00340-6
[5]. Hu, X., Tian, Y., Nagato, K., Nakao, M., & Liu, A. (2023). Opportunities and challenges of ChatGPT for design knowledge management. Procedia CIRP, 119, 21–28. https://doi.org/10.1016/j.procir.2023.05.001
[6]. Chan, C. K. Y., & Lee, K. K. W. (2023). The AI generation gap: Are Gen Z students more interested in adopting generative AI such as ChatGPT in teaching and learning than their Gen X and millennial generation teachers? Smart Learning Environments, 10(1), 60. https://doi.org/10.1186/s40561-023-00269-3
[7]. Feng, C. (Mitsu), Botha, E., & Pitt, L. (2024). From HAL to GenAI: Optimize chatbot impacts with CARE. Business Horizons. Advance online publication. https://doi.org/10.1016/j.bushor.2024.04.012
[8]. Li, L. (2023). Everyday AI sensemaking of freelance knowledge workers. In Companion Publication of the 2023 Conference on Computer Supported Cooperative Work and Social Computing (pp. 452–454). Association for Computing Machinery. https://doi.org/10.1145/3584931.3608924
[9]. Zhang, P., & Soergel, D. (2014). Towards a comprehensive model of the cognitive process and mechanisms of individual sensemaking. Journal of the Association for Information Science and Technology, 65(9), 1733–1756. https://doi.org/10.1002/asi.23125
[10]. Odden, T. O. B., & Russ, R. S. (2019). Defining sensemaking: Bringing clarity to a fragmented theoretical construct. Science Education, 103(1), 187–205. https://doi.org/10.1002/sce.21452
[11]. Norman, D. A., & Bobrow, D. G. (1975). On the role of active memory processes in perception and cognition (Technical Report No. 76-02). Center for Human Information Processing, University of California, San Diego.
[12]. Russell, D. M., Stefik, M. J., Pirolli, P., & Card, S. K. (1993). The cost structure of sensemaking. In Proceedings of the INTERACT ’93 and CHI ’93 Conference on Human Factors in Computing Systems (pp. 269–276). Association for Computing Machinery. https://doi.org/10.1145/169059.169209
[13]. Qian, X., & Fang, Y. (2024). Mitigating algorithm aversion through sensemaking? A revisit to the explanation-seeking process. PACIS 2024 Proceedings.
[14]. Gero, K. I., Swoopes, C., Gu, Z., Kummerfeld, J. K., & Glassman, E. L. (2024). Supporting sensemaking of large language model outputs at scale. Proceedings of the CHI Conference on Human Factors in Computing Systems (pp. 1–21). Association for Computing Machinery. https://doi.org/10.1145/3613904.3642139
[15]. Kanarik, K. J., Osowiecki, W. T., Lu, Y. (Joe), Talukder, D., Roschewsky, N., Park, S. N., Kamon, M., Fried, D. M., & Gottscho, R. A. (2023). Human–machine collaboration for improving semiconductor process development. Nature, 616(7958), 707–711. https://doi.org/10.1038/s41586-023-05773-7
[16]. Pizoń, J., & Gola, A. (2023). Human–machine relationship—Perspective and future roadmap for Industry 5.0 solutions. Machines, 11(2), 203. https://doi.org/10.3390/machines11020203
[17]. Ren, M., Chen, N., & Qiu, H. (2023). Human-machine collaborative decision-making: An evolutionary roadmap based on cognitive intelligence. International Journal of Social Robotics, 15(7), 1101–1114. https://doi.org/10.1007/s12369-023-01020-1
[18]. Sowa, K., Przegalinska, A., & Ciechanowski, L. (2021). Cobots in knowledge work: Human–AI collaboration in managerial professions. Journal of Business Research, 125, 135–142. https://doi.org/10.1016/j.jbusres.2020.11.038
[19]. Sowa, K., & Przegalinska, A. (2020). Digital coworker: Human-AI collaboration in work environment, on the example of virtual assistants for management professions. In A. Przegalinska, F. Grippa, & P. A. Gloor (Eds.), Digital transformation of collaboration (pp. 179–201). Springer. https://doi.org/10.1007/978-3-030-48993-9_13
[20]. Cao, S., Jiang, W., Wang, J., & Yang, B. (2024). From man vs. machine to man + machine: The art and AI of stock analyses. Journal of Financial Economics, 160, 103910. https://doi.org/10.1016/j.jfineco.2024.103910
[21]. Hoc, J.-M. (2000). From human–machine interaction to human–machine cooperation. Ergonomics, 43(7), 833–843. https://doi.org/10.1080/001401300409044
[22]. Lin, H., Han, J., Wu, P., Wang, J., Tu, J., Tang, H., & Zhu, L. (2023). Machine learning and human-machine trust in healthcare: A systematic survey. CAAI Transactions on Intelligence Technology. Advance online publication. https://doi.org/10.1049/cit2.12268
[23]. Xue, C., Zhang, H., & Cao, H. (2024). Multi-agent modelling and analysis of the knowledge learning of a human-machine hybrid intelligent organization with human-machine trust. Systems Science & Control Engineering, 12(1), 2343301. https://doi.org/10.1080/21642583.2024.2343301
[24]. Reich, T., Kaju, A., & Maglio, S. J. (n.d.). How to overcome algorithm aversion: Learning from mistakes. Journal of Consumer Psychology. Advance online publication. https://doi.org/10.1002/jcpy.1313
[25]. Nonaka, I., Takeuchi, H., & Umemoto, K. (1996). A theory of organizational knowledge creation. International Journal of Technology Management, 11(7–8), 833–845.
[26]. Al-Emran, M., Mezhuyev, V., Kamaludin, A., & Shaalan, K. (2018). The impact of knowledge management processes on information systems: A systematic review. International Journal of Information Management, 43, 173–187. https://doi.org/10.1016/j.ijinfomgt.2018.08.001
[27]. Zhang, J. (1997). The nature of external representations in problem solving. Cognitive Science, 21(2), 179–217.
[28]. Richardson, M., & Ball, L. J. (2009). Internal representations, external representations and ergonomics: Towards a theoretical integration. Theoretical Issues in Ergonomics Science, 10(4), 335–376. https://doi.org/10.1080/14639220802368872
[29]. Piaget, J. (1976). Piaget’s theory. In B. Inhelder, H. H. Chipman, & C. Zwingmann (Eds.), Piaget and his school (pp. 11–23). Springer. https://doi.org/10.1007/978-3-642-46323-5_2
[30]. Rumelhart, D. E., & Norman, D. A. (1976). Accretion, tuning and restructuring: Three modes of learning (Report No. 7602). Center for Human Information Processing, University of California, San Diego.
[31]. Vosniadou, S., & Brewer, W. F. (1987). Theories of knowledge restructuring in development. Review of Educational Research, 57(1), 51–67. https://doi.org/10.3102/00346543057001051
[32]. Chi, M. T. (2009). Three types of conceptual change: Belief revision, mental model transformation, and categorical shift. In S. Vosniadou (Ed.), International handbook of research on conceptual change (pp. 89–110). Routledge.
[33]. Pontis, S., & Blandford, A. (2016). Understanding “influence”: An empirical test of the Data-Frame Theory of Sensemaking. Journal of the Association for Information Science and Technology, 67(4), 841–858. https://doi.org/10.1002/asi.23427
[34]. Katila, R., & Ahuja, G. (2017). Something old, something new: A longitudinal study of search behavior and new product introduction. Academy of Management Journal, 45(8), 1183–1194. https://doi.org/10.5465/3069433
[35]. Qu, Y., & Furnas, G. W. (2008). Model-driven formative evaluation of exploratory search: A study under a sensemaking framework. Information Processing & Management, 44(2), 534–555.
[36]. Shen, J., & Linn, M. C. (2011). A technology-enhanced unit of modeling static electricity: Integrating scientific explanations and everyday observations. International Journal of Science Education, 33(12), 1597–1623. https://doi.org/10.1080/09500693.2010.514012
[37]. Lyu, C., Yang, J., Zhang, F., Teo, T. S. H., & Mu, T. (2020). How do knowledge characteristics affect firm’s knowledge sharing intention in interfirm cooperation? An empirical study. Journal of Business Research, 115, 48–60. https://doi.org/10.1016/j.jbusres.2020.04.045
[38]. Yagoda, R. E., & Gillan, D. J. (2012). You want me to trust a ROBOT? The development of a human–robot interaction trust scale. International Journal of Social Robotics, 4(3), 235–248. https://doi.org/10.1007/s12369-012-0144-0
[39]. Chi, O. H., Jia, S., Li, Y., & Gursoy, D. (2021). Developing a formative scale to measure consumers’ trust toward interaction with artificially intelligent (AI) social robots in service delivery. Computers in Human Behavior, 118, 106700. https://doi.org/10.1016/j.chb.2021.106700
[40]. Lyles, M. A., & Salk, J. E. (1996). Knowledge acquisition from foreign parents in international joint ventures: An empirical examination in the Hungarian context. Journal of International Business Studies, 27(5), 877–903. https://doi.org/10.1057/palgrave.jibs.8490155
[41]. Basaglia, S., Caporarello, L., Magni, M., & Pennarola, F. (2010). IT knowledge integration capability and team performance: The role of team climate. International Journal of Information Management, 30(6), 542–551. https://doi.org/10.1016/j.ijinfomgt.2010.04.003
[42]. Sarwat, N., & Abbas, M. (2020). Individual knowledge creation ability: Dispositional antecedents and relationship to innovative performance. European Journal of Innovation Management, 24(5), 1763–1781. https://doi.org/10.1108/EJIM-05-2020-0198
[43]. Hoon Song, J., Uhm, D., & Won Yoon, S. (2011). Organizational knowledge creation practice: Comprehensive and systematic processes for scale development. Leadership & Organization Development Journal, 32(3), 243–259. https://doi.org/10.1108/01437731111123906
[44]. Han, Z., & Battaglia, F. (2024). Transforming challenges into opportunities: Leveraging ChatGPT’s limitations for active learning and prompt engineering skill. The Innovation.
[45]. Walter, Y. (2024). Embracing the future of artificial intelligence in the classroom: The relevance of AI literacy, prompt engineering, and critical thinking in modern education. International Journal of Educational Technology in Higher Education, 21(1), 15. https://doi.org/10.1186/s41239-024-00448-3
[46]. Lo, L. S. (2023). The art and science of prompt engineering: A new literacy in the information age. Internet Reference Services Quarterly, 27(4), 203–210. https://doi.org/10.1080/10875301.2023.2227621
[47]. Ortolan, P. (2023). Optimizing prompt engineering for improved generative AI content.
[48]. Johnson, M., Albizri, A., Harfouche, A., & Fosso-Wamba, S. (2022). Integrating human knowledge into artificial intelligence for complex and ill-structured problems: Informed artificial intelligence. International Journal of Information Management, 64, 102479. https://doi.org/10.1016/j.ijinfomgt.2022.102479
Cite this article
Yang,X.;Hao,Y.;Guo,J. (2025). Mind meets machine: how do humans and AI assistants co-create knowledge? . Journal of Applied Economics and Policy Studies,18(3),19-35.
Data availability
The datasets used and/or analyzed during the current study will be available from the authors upon reasonable request.
Disclaimer/Publisher's Note
The statements, opinions and data contained in all publications are solely those of the individual author(s) and contributor(s) and not of EWA Publishing and/or the editor(s). EWA Publishing and/or the editor(s) disclaim responsibility for any injury to people or property resulting from any ideas, methods, instructions or products referred to in the content.
About volume
Journal:Journal of Applied Economics and Policy Studies
© 2024 by the author(s). Licensee EWA Publishing, Oxford, UK. This article is an open access article distributed under the terms and
conditions of the Creative Commons Attribution (CC BY) license. Authors who
publish this series agree to the following terms:
1. Authors retain copyright and grant the series right of first publication with the work simultaneously licensed under a Creative Commons
Attribution License that allows others to share the work with an acknowledgment of the work's authorship and initial publication in this
series.
2. Authors are able to enter into separate, additional contractual arrangements for the non-exclusive distribution of the series's published
version of the work (e.g., post it to an institutional repository or publish it in a book), with an acknowledgment of its initial
publication in this series.
3. Authors are permitted and encouraged to post their work online (e.g., in institutional repositories or on their website) prior to and
during the submission process, as it can lead to productive exchanges, as well as earlier and greater citation of published work (See
Open access policy for details).