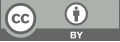
Daily Stock Selection
- 1 Columbia University
- 2 Columbia University
- 3 Columbia University
* Author to whom correspondence should be addressed.
Abstract
This study aims to investigate the predictive power of various financial indicators in forecasting daily stock returns. To achieve this goal, we collected a year’s worth of stock data for the stocks listed in S&P 500 using yfinance, which included market capitalization, sector, PE ratios, revenue, earnings per share, and other variables. Our methodology involved analyzing stock performance predictors using advanced statistical methods and machine learning models, including Random Forest, Gradient Boosting, and Generalized Linear Model Regression. The findings suggest that daily stock returns can be significantly predicted by a few financial factors including PE ratio, PB ratio, and Debt to Equity ratio. To assess the performance of our models, we performed a 12-fold cross validation on the validatable models Gradient Boosting and Random Forest. The result demonstrated that Gradient Boosting provided the most reliable predictions based on mean squared error analysis. This study validates the robustness of using historical stock information in forecasting short-term stock returns, providing insights that could benefit investors in understanding market dynamics.
Keywords
daily stock returns, financial indicators, market dynamics
[1]. Binder, J. J., & Merges, M. J. (2000). Stock market volatility and economic factors. Review of Quantitative Finance and Accounting. Available at SSRN: https://ssrn.com/abstract=265272 or http://dx.doi.org/10.2139/ssrn.265272.
[2]. Bin, O. A., Huang, S., Salameh, A. A., Khurram, H., & Fareed, M. (2022). Stock market forecasting using the random forest and deep neural network models before and during the COVID-19 period. Frontiers in Environmental Science, 10.
[3]. Baker, M., & Wurgler, J. (2007). Investor sentiment in the stock market. Journal of Economic Perspectives, 21(2), 129–152.
[4]. Cvetkov-Iliev, A., Allauzen, A., & Varoquaux, G. (2022). Analytics on nonnormalized data sources: More learning, rather than more cleaning. IEEE Access, 10, 42420–42431.
[5]. Caginalp, G., & Laurent, H. (1998). The predictive power of price patterns. Applied Mathematical Finance, 5, 181–206. Available at SSRN: https://ssrn.com/abstract=932984.
[6]. Consoli, S., Reforgiato Recupero, D., & Saisana, M. (2021). Data Science for Economics and Finance: Methodologies and Applications. Springer.
[7]. Davis, J., Devos, L., Reyners, S., & Schoutens, W. (2020). Gradient boosting for quantitative finance. Journal of Computational Finance, 24(4). Available at SSRN: https://ssrn.com/abstract=3829891.
[8]. Fang, Y., Liu, X.-Y., & Yang, H. (2019). Practical machine learning approach for stock trading strategies using alternative dataset. Available at SSRN: https://ssrn.com/abstract=3501239 or http://dx.doi.org/10.2139/ssrn.3501239.
[9]. KDnuggets. (2022). Random Forest Algorithm: Do We Need Normalization? https://www.kdnuggets.com/2022/07/random-forest-algorithm-need-normalization. [Online; accessed 25-April-2024].
[10]. Krauss, C., & Stübinger, J. (2015). Nonlinear dependence modeling with bivariate copulas: Statistical arbitrage pairs trading on the S&P 100. FAU Discussion Papers in Economics, 15/2015. Friedrich-Alexander University Erlangen-Nuremberg, Institute for Economics.
[11]. Nayanar, N. (2023). Interpreting institutional investment activity as a Markov process: A stock recommender. Intelligent Decision Technologies, 17(3), 1–13.
[12]. Ngo, T. H. D. (2016). Generalized linear models for non-normal data. https://support.sas.com/resources/papers/proceedings16/8380-2016.pdf. Accessed: 2024-04-25.
[13]. Raymond, M. R. (1986). Missing data in evaluation research. Evaluation & the Health Professions, 9(4), 395–420.
[14]. Rebonato, R. (2004). Volatility and Correlation: The Perfect Hedger and the Fox (2nd ed.). John Wiley & Sons.
Cite this article
Liu,Y.;Zhang,X.;Zhang,L. (2024). Daily Stock Selection. Journal of Fintech and Business Analysis,1,77-86.
Data availability
The datasets used and/or analyzed during the current study will be available from the authors upon reasonable request.
Disclaimer/Publisher's Note
The statements, opinions and data contained in all publications are solely those of the individual author(s) and contributor(s) and not of EWA Publishing and/or the editor(s). EWA Publishing and/or the editor(s) disclaim responsibility for any injury to people or property resulting from any ideas, methods, instructions or products referred to in the content.
About volume
Journal:Journal of Fintech and Business Analysis
© 2024 by the author(s). Licensee EWA Publishing, Oxford, UK. This article is an open access article distributed under the terms and
conditions of the Creative Commons Attribution (CC BY) license. Authors who
publish this series agree to the following terms:
1. Authors retain copyright and grant the series right of first publication with the work simultaneously licensed under a Creative Commons
Attribution License that allows others to share the work with an acknowledgment of the work's authorship and initial publication in this
series.
2. Authors are able to enter into separate, additional contractual arrangements for the non-exclusive distribution of the series's published
version of the work (e.g., post it to an institutional repository or publish it in a book), with an acknowledgment of its initial
publication in this series.
3. Authors are permitted and encouraged to post their work online (e.g., in institutional repositories or on their website) prior to and
during the submission process, as it can lead to productive exchanges, as well as earlier and greater citation of published work (See
Open access policy for details).