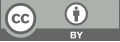
Performance analysis of three recommendation algorithms on Amazon datasets
- 1 Southern University of Science and Technology
* Author to whom correspondence should be addressed.
Abstract
Recommendation systems play a crucial role in enhancing user satisfaction and driving sales for businesses. They are essential in today’s marketplaces, as they are able to suggest products and services that may interest a particular individual based on their past purchases. In this paper, empirical research is conducted on three distinct subsets of the Amazon dataset, namely Sports & Outdoors, Movies & TV, and Video Games, to comprehensively evaluate the performance of three distinct recommendation methods based on deep learning algorithms. These methods include the dot product method, (an updated version of the singular value decomposition algorithm), the neural network method, and the neural collaborative filtering model with natural language processing method. The results of this study reveal that deep learning-based recommendation systems can achieve more accurate results compared to traditional recommendation systems for three types of products. The implementation of these methods on Amazon’s dataset can help improve sales by correctly identifying customers’ interests and suggesting relevant items.
Keywords
Recommendation System, Machine Learning, Neural Network
[1]. Ko, H., Lee, S., Park, Y., & Choi, A. (2022). A survey of recommendation systems: recommendation models, techniques, and application fields. Electronics, 11(1), 141.
[2]. Isinkaye, F. O., Folajimi, Y. O., & Ojokoh, B. A. (2015). Recommendation systems: Principles, methods and evaluation. Egyptian informatics journal, 16(3), 261-273.
[3]. Jin, W., Mao, H., Li, Z., Jiang, H., Luo, C., Wen, H., et al. (2023). Amazon-M2: A Multilingual Multi-locale Shopping Session Dataset for Recommendation and Text Generation. arXiv preprint arXiv:2307.09688.
[4]. Wei, Y., Ma, H., Zhang, R., Li, Z., & Chang, L. (2021). Exploring implicit relationships in social network for recommendation systems. In Pacific-Asia Conference on Knowledge Discovery and Data Mining, 386-397.
[5]. Recommender System Using Amazon Reviews, URL: https://www.kaggle.com/code/saurav9786/recommender-system-using-amazon-reviews. Last Accessed: 2023/09/10
[6]. Koren, Y., Bell, R., & Volinsky, C. (2009). Matrix factorization techniques for recommender systems. Computer, 42(8), 30-37.
[7]. Xue, H. J., Dai, X., Zhang, J., Huang, S., & Chen, J. (2017). Deep matrix factorization models for recommender systems. In IJCAI, 17, 3203-3209.
[8]. Wu, S., Sun, F., Zhang, W., Xie, X., & Cui, B. (2022). Graph neural networks in recommender systems: a survey. ACM Computing Surveys, 55(5), 1-37.
[9]. Paradarami, T. K., Bastian, N. D., & Wightman, J. L. (2017). A hybrid recommender system using artificial neural networks. Expert Systems with Applications, 83, 300-313.
[10]. He, X., Liao, L., Zhang, H., Nie, L., Hu, X., & Chua, T. S. (2017). Neural collaborative filtering. In Proceedings of the 26th international conference on world wide web, 173-182.
Cite this article
Meng,Y. (2024). Performance analysis of three recommendation algorithms on Amazon datasets. Applied and Computational Engineering,51,26-32.
Data availability
The datasets used and/or analyzed during the current study will be available from the authors upon reasonable request.
Disclaimer/Publisher's Note
The statements, opinions and data contained in all publications are solely those of the individual author(s) and contributor(s) and not of EWA Publishing and/or the editor(s). EWA Publishing and/or the editor(s) disclaim responsibility for any injury to people or property resulting from any ideas, methods, instructions or products referred to in the content.
About volume
Volume title: Proceedings of the 4th International Conference on Signal Processing and Machine Learning
© 2024 by the author(s). Licensee EWA Publishing, Oxford, UK. This article is an open access article distributed under the terms and
conditions of the Creative Commons Attribution (CC BY) license. Authors who
publish this series agree to the following terms:
1. Authors retain copyright and grant the series right of first publication with the work simultaneously licensed under a Creative Commons
Attribution License that allows others to share the work with an acknowledgment of the work's authorship and initial publication in this
series.
2. Authors are able to enter into separate, additional contractual arrangements for the non-exclusive distribution of the series's published
version of the work (e.g., post it to an institutional repository or publish it in a book), with an acknowledgment of its initial
publication in this series.
3. Authors are permitted and encouraged to post their work online (e.g., in institutional repositories or on their website) prior to and
during the submission process, as it can lead to productive exchanges, as well as earlier and greater citation of published work (See
Open access policy for details).