About ACEThe proceedings series Applied and Computational Engineering (ACE) is an international peer-reviewed open access series that publishes conference proceedings from various methodological and disciplinary perspectives concerning engineering and technology. ACE is published irregularly. The series contributes to the development of computing sectors by providing an open platform for sharing and discussion. The series publishes articles that are research-oriented and welcomes theoretical and applicational studies. Proceedings that are suitable for publication in the ACE cover domains on various perspectives of computing and engineering. |
Aims & scope of ACE are: ·Computing ·Machine Learning ·Electrical Engineering & Signal Processing ·Applied Physics & Mechanical Engineering ·Chemical & Environmental Engineering ·Materials Science and Engineering |
Article processing charge
A one-time Article Processing Charge (APC) of 450 USD (US Dollars) applies to papers accepted after peer review. excluding taxes.
Open access policy
This is an open access journal which means that all content is freely available without charge to the user or his/her institution. (CC BY 4.0 license).
Your rights
These licenses afford authors copyright while enabling the public to reuse and adapt the content.
Peer-review process
Our blind and multi-reviewer process ensures that all articles are rigorously evaluated based on their intellectual merit and contribution to the field.
Editors View full editorial board
United Kingdom
Malaysia
United Kingdom
United Kingdom
yilun.shang@northumbria.ac.uk
Latest articles View all articles

In the context of significant renewable energy integration, power load forecasting is viewed as an essential task in energy management and power system operation and scheduling. In an effort to enhance the accuracy and precision of power load prediction, a predictive technique based on Long Short-Term Memory (LSTM) networks enhanced by the quantum-behaved particle swarm optimization (QPSO) is applied to ultra-short-term power load prediction in this paper. Initially, normalization is used to preprocess power load data before it is divided into training and testing datasets. Subsequently, global optimization of the LSTM’s essential hyperparameters and network architecture is conducted via QPSO, resulting in the development of a QPSO-LSTM forecasting model. Subsequently, the forecasting model is evaluated by employing the coefficient of determination (R²), mean absolute error (MAE), mean absolute percentage error (MAPE), and root mean square error (RMSE) as performance metrics. Finally, comparative experiments are conducted between the proposed model and traditional neural network models. The findings demonstrate that the QPSO-LSTM model offers enhanced forecasting precision and optimal fitting performance.
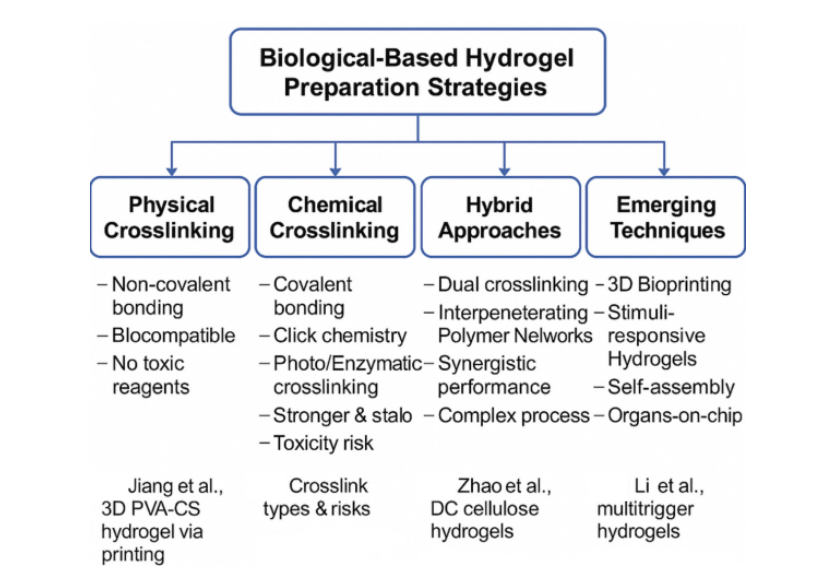
Bio-based hydrogels, derived from natural materials such as chitosan, alginate, gelatin, and collagen, have garnered significant attention for their outstanding biocompatibility and ability to mimic natural tissues. This review examines commonly used preparation methods, including physical, chemical and hybrid cross-linking, along with their primary components, such as polysaccharides and proteins. Owing to their flexibility and responsiveness, these hydrogels are widely used in areas such as soft robotics, cancer therapy and biosensing. However, despite promising advancements, significant challenges persist, particularly regarding their limited strength and stability. Future research should aim to enhance the performance and reliability of these materials to support their integration into complex medical and engineering systems.
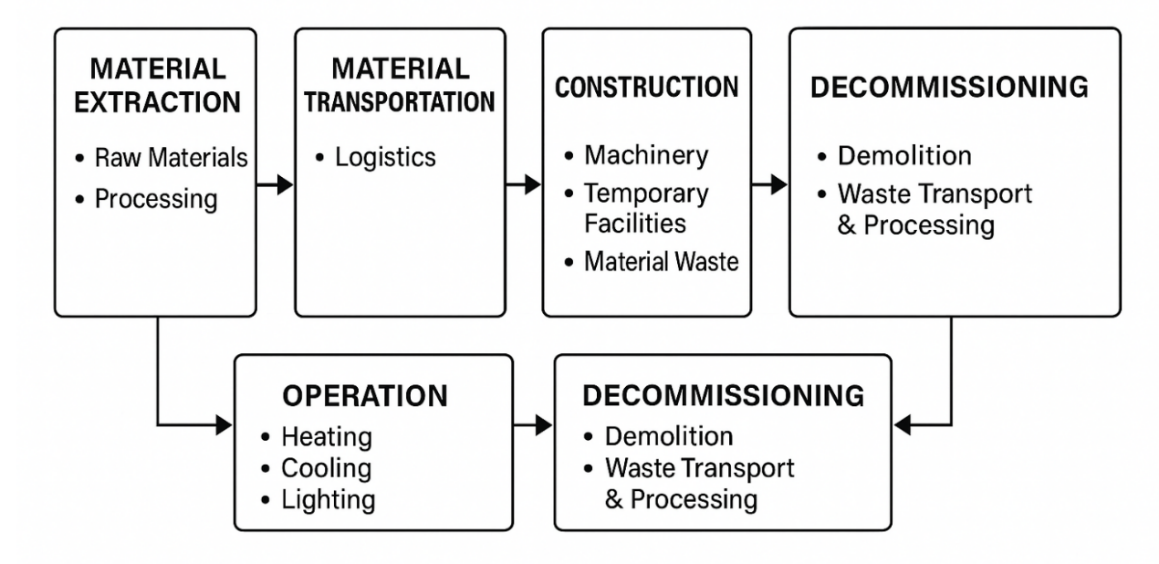
To enable systematic quantification and effective control of carbon emissions in the construction industry, this paper proposes a life cycle-based carbon emission model. Grounded in LCA principles, the model spans four stages: material production, construction, operation, and demolition. It integrates phased accounting with unified aggregation to ensure a closed-loop calculation process. Parameters are derived from the “Building Carbon Emission Calculation Standard” and the China Life Cycle Database (CLCD). Empirical validation on public buildings in Shanghai demonstrates the model’s stability and its ability to identify high-emission stages and optimization opportunities. The model proves applicable to carbon verification, green building evaluation, and full-process carbon management, showing strong practical value and scalability.
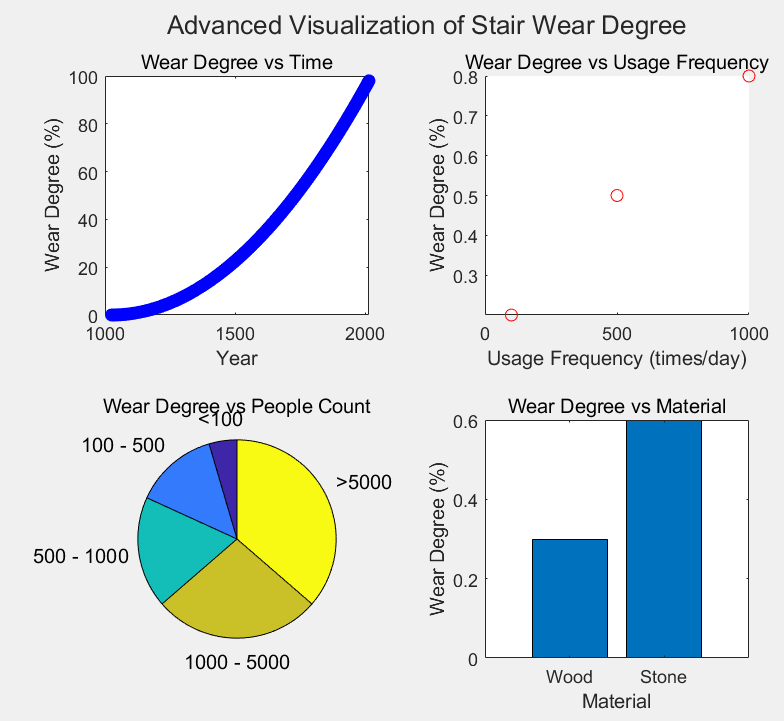
This paper focuses on the research of ancient building staircases, developing multiple models for wear analysis and traffic flow prediction. Firstly, relevant data on wear and traffic flow are collected, including material properties, surface conditions, and the number of staircase users. Model I is based on material mechanics and surface wear theory, constructing W = f (F, D, M) to examine the degree of wear; Model II combines LWR traffic flow and queuing theory, constructing Q = g(ρ, v, lq, tq) to analyze traffic flow; Model III is a PSO model that improves the first two, using the mean square error between predicted and actual data as the fitness function. The results show that the staircase wear model has good explanatory power, the traffic flow model has a high accuracy rate during peak and off-peak hours, and the mean square error of the combined model after PSO optimization is reduced by 30%, with improved fitting performance, providing an important reference for the maintenance, protection, and usage planning of ancient building staircases.
Volumes View all volumes
Volume 172June 2025
Find articlesProceedings of CONF-FMCE 2025 Symposium: Semantic Communication for Media Compression and Transmission
Conference website: https://2025.conffmce.org/glasgow.html
Conference date: 24 October 2025
ISBN: 978-1-80590-221-8(Print)/978-1-80590-222-5(Online)
Editor: Anil Fernando
Volume 171June 2025
Find articlesProceedings of the 3rd International Conference on Functional Materials and Civil Engineering
Conference website: https://2025.conffmce.org/
Conference date: 24 October 2025
ISBN: 978-1-80590-219-5(Print)/978-1-80590-220-1(Online)
Editor: Anil Fernando
Volume 170June 2025
Find articlesProceedings of the 3rd International Conference on Mechatronics and Smart Systems
Conference website: https://2025.confmss.org/
Conference date: 16 June 2025
ISBN: 978-1-80590-217-1(Print)/978-1-80590-218-8(Online)
Editor: Mian Umer Shafiq
Volume 169June 2025
Find articlesProceedings of CONF-MSS 2025 Symposium: Machine Vision System
Conference website: https://www.confmss.org/chicago.html
Conference date: 5 June 2025
ISBN: 978-1-80590-209-6(Print)/978-1-80590-210-2(Online)
Editor: Marwan Omar, Cheng Wang
Announcements View all announcements
Applied and Computational Engineering
We pledge to our journal community:
We're committed: we put diversity and inclusion at the heart of our activities...
Applied and Computational Engineering
The statements, opinions and data contained in the journal Applied and Computational Engineering (ACE) are solely those of the individual authors and contributors...
Indexing
The published articles will be submitted to following databases below:
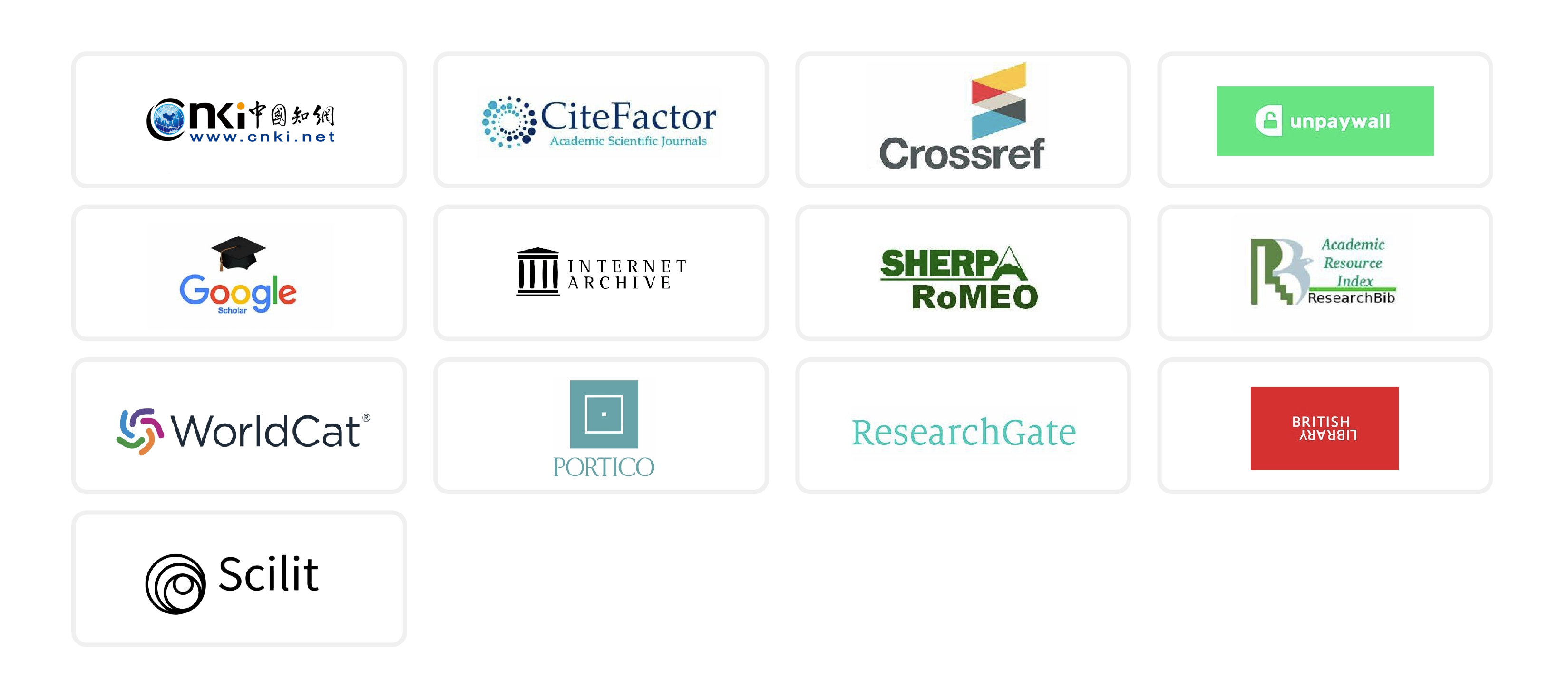