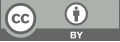
House price prediction using machine learning
- 1 GuangDong University Of Technology
* Author to whom correspondence should be addressed.
Abstract
The role of the real estate industry in economic development and social progress reflects the economic well-being of individuals and regions. With the increase of people's income level, the demand for housing is also increasing. Therefore, making a more accurate house price forecast will help people make the most correct strategy to buy a house when they need it. This study focuses on house price prediction in King County, Washington, a diverse real estate market. Leveraging machine learning models such as linear regression, random forest, neural networks and XGBoost, these supervised learning models are used to delve into house price forecasting. This research includes random forest and XGBoost, are implemented using Scikit-Learn tools. Besides, the Feedforward Neural Network is introduced with the drop out layer in order to reduce the occurrence of model fitting situations. The findings reveal that XGBoost achieves the highest accuracy, making it well-suited for precise price predictions. Additionally, the research identifies grade, sqft_living, and latitude as the three most influential features significantly affecting house prices within the dataset.
Keywords
House price prediction, linear regression, random forest, neural networks, XGBoost
[1]. Maslow, A. H. (1943). A theory of human motivation. Psychological Review, 50 (4), 370-96.
[2]. “King County, Washington”, wikipedia, 31 October 2023, https://en.wikipedia.org/wiki/King_County,_Washington
[3]. House Sales in King County, USA, https://www.kaggle.com/datasets/harlfoxem/housesalesprediction/data
[4]. Visualization-on-a-Map https://www.kaggle.com/code/chrisbronner/regression-r2-0-82-and-map-visualization#3.
[5]. N. N. Ghosalkar and S. N. Dhage, "Real Estate Value Prediction Using Linear Regression," 2018 Fourth International Conference on Computing Communication Control and Automation (ICCUBEA), Pune, India, 2018, pp. 1-5, doi:10.1109/ICCUBEA.2018.8697639
[6]. Breiman L. Random Forests. SpringerLink. https://doi.org/10.1023/A:1010933404324 (accessed September 11, 2019).
[7]. Raschka S, Mirjalili V. Python machine learning: Machine learning and deep learning with Python, scikit-learn, and TensorFlow. 2nd ed. Birmingham: Packt Publishing; 2017.
[8]. Cireşan, D.C.; Meier, U.; Gambardella, L.M.; Schmidhuber, J. Deep, big, simple neural nets for handwritten digit recognition. Neural Comput. 2010, 22, 3207–3220.
[9]. T. Chen, C. Guestrin XGBoost: A scalable tree boosting system, Association for Computing Machinery (2016), pp. 785-794
[10]. W. Dong, Y. Huang, B. Lehane, G. Ma XGBoost algorithm-based prediction of concrete electrical resistivity for structural health monitoring and Automation in Construction, 114 (2020), p. 103155
Cite this article
Li,C. (2024). House price prediction using machine learning. Applied and Computational Engineering,53,225-237.
Data availability
The datasets used and/or analyzed during the current study will be available from the authors upon reasonable request.
Disclaimer/Publisher's Note
The statements, opinions and data contained in all publications are solely those of the individual author(s) and contributor(s) and not of EWA Publishing and/or the editor(s). EWA Publishing and/or the editor(s) disclaim responsibility for any injury to people or property resulting from any ideas, methods, instructions or products referred to in the content.
About volume
Volume title: Proceedings of the 4th International Conference on Signal Processing and Machine Learning
© 2024 by the author(s). Licensee EWA Publishing, Oxford, UK. This article is an open access article distributed under the terms and
conditions of the Creative Commons Attribution (CC BY) license. Authors who
publish this series agree to the following terms:
1. Authors retain copyright and grant the series right of first publication with the work simultaneously licensed under a Creative Commons
Attribution License that allows others to share the work with an acknowledgment of the work's authorship and initial publication in this
series.
2. Authors are able to enter into separate, additional contractual arrangements for the non-exclusive distribution of the series's published
version of the work (e.g., post it to an institutional repository or publish it in a book), with an acknowledgment of its initial
publication in this series.
3. Authors are permitted and encouraged to post their work online (e.g., in institutional repositories or on their website) prior to and
during the submission process, as it can lead to productive exchanges, as well as earlier and greater citation of published work (See
Open access policy for details).