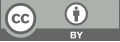
Application of deep learning-based Intrusion Detection System (IDS) in network anomaly traffic detection
- 1 Computer Science, Stevens Institute of Technology, NJ, USA
- 2 Computer Engineering, New York University, New York, USA
- 3 Artificial intelligence, Royal Holloway University of London, Egham, UK
- 4 Computer Science, University of Electronic Science and Technology of China, Cheng Du, China
- 5 Information System & Technology Data Analytics, California State University, CA, USA
* Author to whom correspondence should be addressed.
Abstract
This study discusses the application of deep learning technology in network intrusion detection systems (IDS) and focuses on a new model named CNN-Focal. First, reviewing traditional IDS technology, it analyzes its limitations in dealing with complex network traffic. Then, the design principle of the CNN-Focal model is described in detail, which uses threshold convolution and SoftMax multi-class classification technology to improve abnormal traffic detection’s accuracy and efficiency effectively. The experimental results show that CNN-Focal performs well on the open data set, demonstrating the potential and advantages of its application in the natural network environment and providing a new perspective and method for further research of deep learning in the field of network security in the future.
Keywords
Deep learning, Intrusion detection System (IDS), CNN-Focal Model, Abnormal network traffic
[1]. Sagduyu, Yalin E., Yi Shi, and Tugba Erpek. “IoT network security from the perspective of adversarial deep learning.” 2019 16th Annual IEEE International Conference on Sensing, Communication, and Networking (SECON). IEEE, 2019.
[2]. Alom, M. Z., & Taha, T. M. (2017, June). Network intrusion detection for cyber security using unsupervised deep learning approaches. In 2017 IEEE National Aerospace and Electronics Conference (NAECON) (pp. 63-69). IEEE.
[3]. Kumar, C., Bharati, T. S., & Prakash, S. (2021). Online social network security: a comparative review using machine learning and deep learning. Neural Processing Letters, 53(1), 843-861.
[4]. Gong Y, Zhu M, Huo S, et al. Utilizing Deep Learning for Enhancing Network Resilience in Finance[C]//2024 7th International Conference on Advanced Algorithms and Control Engineering (ICAACE). IEEE, 2024: 987-991.
[5]. Uppal, H. A. M., Javed, M., & Arshad, M. (2014). An overview of the intrusion detection system (IDS) and its commonly used techniques and classifications. International Journal of Computer Science and Telecommunications, 5(2), 20-24.
[6]. Abbas, S. H., Naser, W. A. K., & Kadhim, A. A. (2023). Subject review: Intrusion Detection System (IDS) and Intrusion Prevention System (IPS). Global Journal of Engineering and Technology Advances, 14(2), 155-158.
[7]. Pradhan, M., Nayak, C. K., & Pradhan, S. K. (2020). Intrusion detection systems (IDS) and their types. In Securing the Internet of Things: Concepts, methodologies, tools, and applications (pp. 481-497). IGI Global.
[8]. Tian, J., Li, H., Qi, Y., Wang, X., & Feng, Y. (2024). Intelligent medical detection and diagnosis assisted by deep learning. Applied and Computational Engineering, 64, 121-126.
[9]. Borkar, A., Donode, A., & Kumari, A. (2017, November). A survey on the Intrusion Detection System (IDS) and Internal Intrusion Detection and Protection System (IIDPS). In 2017 International Conference on Inventive Computing and Informatics (ICICI) (pp. 949-953). IEEE.
[10]. Liu, B., Cai, G., Ling, Z., Qian, J., & Zhang, Q. (2024). Precise positioning and prediction system for autonomous driving based on generative artificial intelligence. Applied and Computational Engineering, 64, 42-49.
Cite this article
Zhao,F.;Li,H.;Niu,K.;Shi,J.;Song,R. (2024). Application of deep learning-based Intrusion Detection System (IDS) in network anomaly traffic detection. Applied and Computational Engineering,86,231-237.
Data availability
The datasets used and/or analyzed during the current study will be available from the authors upon reasonable request.
Disclaimer/Publisher's Note
The statements, opinions and data contained in all publications are solely those of the individual author(s) and contributor(s) and not of EWA Publishing and/or the editor(s). EWA Publishing and/or the editor(s) disclaim responsibility for any injury to people or property resulting from any ideas, methods, instructions or products referred to in the content.
About volume
Volume title: Proceedings of the 6th International Conference on Computing and Data Science
© 2024 by the author(s). Licensee EWA Publishing, Oxford, UK. This article is an open access article distributed under the terms and
conditions of the Creative Commons Attribution (CC BY) license. Authors who
publish this series agree to the following terms:
1. Authors retain copyright and grant the series right of first publication with the work simultaneously licensed under a Creative Commons
Attribution License that allows others to share the work with an acknowledgment of the work's authorship and initial publication in this
series.
2. Authors are able to enter into separate, additional contractual arrangements for the non-exclusive distribution of the series's published
version of the work (e.g., post it to an institutional repository or publish it in a book), with an acknowledgment of its initial
publication in this series.
3. Authors are permitted and encouraged to post their work online (e.g., in institutional repositories or on their website) prior to and
during the submission process, as it can lead to productive exchanges, as well as earlier and greater citation of published work (See
Open access policy for details).