1. Introduction
Particularly when it comes to producing text from images, in recent years, Generative Adversarial Networks (GANs) have demonstrated exceptional performance in cross-modal production. Text-to-picture generation has great potential in image generation and automated design, as it allows realistic visuals to be automatically generated from textual descriptions. As GANs continue to evolve, they have made remarkable progress in multimodal domains. However, text-to-image generation based on GANs still faces various issues and challenges. Firstly, the quality of images generated from text still needs improvement. Secondly, Uncertainty in the GAN training process is still a challenge that must be overcome because it results in images that are produced with little variation and detail [1].
Since the emergence of cross-modal applications based on GAN, this field has received widespread attention and has given rise to many groundbreaking studies. Generative Adversarial Text-to-Image Synthesis (GAIT) is the first GAN-based text-to-image generation model [2]. It accepts text descriptions as conditional input in addition to random noise vectors as input. However, its shortcomings mainly lie in the low resolution of generated images, its inability to handle complex scenes, insufficient detail generation, and unstable training. Later, Stacked Generative Adversarial Networks (StackGAN) marked a significant breakthrough in this field by gradually generating high-resolution images through a multi-stage process [3-5]. In addition, Attentional Generative Adversarial Networks (AttnGAN) is another major advancement, as it introduced a multi-level attention mechanism and improved fine-grained image detail by calculating the similarity between text and image features [6-9]. As was already indicated, there are still problems in the text-to-image creation area, such as poor model stability and low resolution of created images. Furthermore, effectively handling complex text descriptions remains a key area of research focus today.
The main objective of this research is to perform a thorough examination of GAN-based Text-to-Image Generation, emphasizing the technology's foundational ideas and evolution. The study begins by introducing the core concepts behind these models, followed by a detailed comparison of the experimental performance of key models in this field. Furthermore, it explores the strengths, limitations, and potential future advancements of these models. This is how the rest of the paper is organized: Section Ⅱ delves into the characteristics and applications of StackGAN and AttnGAN, providing a comparative analysis of their performance. Section Ⅲ discusses the challenges currently faced in this field, along with potential solutions to overcome these obstacles. Lastly, Section Ⅳ concludes with key insights and discusses future prospects for GAN-based Text-to-Image Generation.
2. Methodology
2.1. Dataset description and preprocessing
A portion of the CUB-200-2011 bird image dataset, which has 11,788 images total from 200 different bird species, is used in this section [5, 10]. Each image is accompanied by 10 textual descriptions, which are annotated by people using the content of the photographs; these annotations usually contain details about the color, shape, posture, and other characteristics of the bird. The Caltech-UCSD Birds (CUB) dataset is commonly used for evaluating models such as StackGAN and AttnGAN, especially when generating detailed bird images is required. The samples are shown in the Figure 1 and Figure 2.
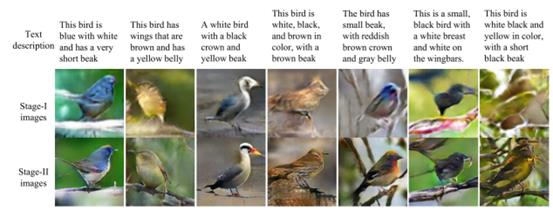
Figure 1. Samples from the CUB test set that were produced by StackGAN from unseen texts. A list of the text descriptions and images produced by the first and second stages of StackGAN are listed in each column.
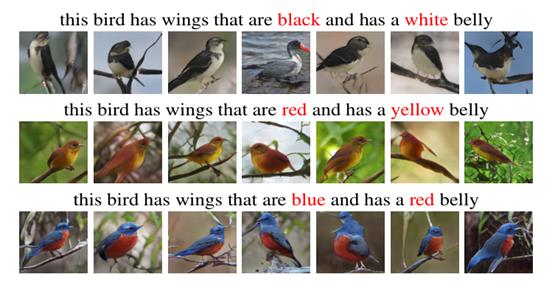
Figure 2. Sample output from AttnGAN model that was trained on CUB with a few of the most frequently attended terms in the text descriptions changed.
2.2. Proposed approach
This review aims to offer a comprehensive overview of GAN, StackGAN, and AttnGAN, explaining the fundamental architectures of these models and emphasizing the mechanisms behind text-to-image generation. It will also examine and compare the key datasets utilized by these models. In addition, the review will evaluate each model's benefits and drawbacks as well. The pipeline is shown in the Figure 3.
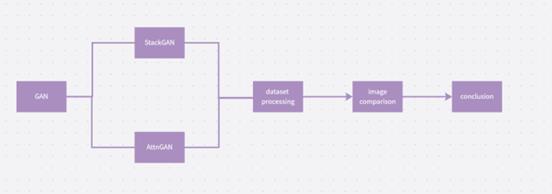
Figure 3. The pipeline of the study.
The primary research approach involves evaluating and comparing the performance of these models across various datasets, drawing conclusions based on the findings, and proposing potential solutions or future directions for improvement.
2.2.1. Introduction of GAN. In the field of deep learning, GANs are a revolutionary technique, in particular for generative tasks like picture synthesis. The Generator and the Discriminator, two rival neural networks involved in a dynamic adversarial process, are at the center of the fundamental idea of a GAN. The Generator's function is to generate data that fits the distribution of real data, while the Discriminator's job is to distinguish between samples that are generated by the Generator and those that are real. With time, the system can improve the generated outputs thanks to this adversarial architecture, which forces the Generator to produce data that is more and more realistic.
The structure of a GAN is centered on this dual-network architecture. The Generator begins by taking a random noise vector as input, which it transforms into a data sample, such as an image, through a series of neural layers. The goal is for this output to resemble real-world data as closely as possible. In contrast, the Discriminator assesses both the synthetic data from the Generator and the actual data. A probability score indicating whether the input is fabricated or real is what it outputs. The Generator gains the ability to produce data that the Discriminator finds more and more challenging to differentiate from actual samples through iterative training.
The beauty of GANs lies in this continuous feedback loop. As the Discriminator improves at recognizing fake data, the Generator becomes better at creating more convincing outputs, leading to highly sophisticated generative models. This adversarial training paradigm has been instrumental in advancing fields such as image generation, video synthesis, and even text-to-image tasks, demonstrating GANs' profound impact on Artificial Intelligence (AI)-driven content creation.
2.2.2. StackGAN. An expansion of the conventional GAN architecture, StackGAN is made expressly to produce high-resolution images from written descriptions. Unlike conventional GANs, which attempt to generate images in a single step, StackGAN employs a multi-stage generation process that progressively refines the quality and detail of the generated image. This staged approach allows StackGAN to produce images that are not only more realistic but also more aligned with the textual input, making it particularly effective for text-to-image generation tasks.
The primary distinction between StackGAN and a standard GAN lies in its two-stage generation process, where the image quality is enhanced progressively rather than all at once. First, a pre-trained text encoder is utilized in Stage-I to translate a supplied text description into a vector representation that contains the description's semantic information. The generator uses this text embedding plus a random noise vector to create a crude, low-resolution image. Right now, the goal is to ensure that the image's fundamental properties are as realistic as possible. High realism is not the aim at this point; rather, it is to make sure that the image's basic composition, color scheme, and overall structure match the text description. For example, if the text says "a yellow bird with black wings," the generated image at this point will show the approximate shape and color palette of that kind of bird, albeit in a low-resolution, coarse manner.
The initial crude image is further refined in Stage-II. In order to create a higher-resolution, more detailed image, the generator gets both the original text embedding and the low-resolution image. This stage is critical for enhancing finer details, such as texture and object precision, while also correcting any distortions or blurriness from the first stage. The discriminator at this stage has a dual role: it is not only required to determine whether the generated image is legitimate, but also to determine how well the image corresponds with the written description. As a result, StackGAN may produce visuals that match the given text semantically.
Overall, StackGAN's staged process distinguishes it from traditional GANs by providing a more structured and scalable approach to image generation. By breaking the task into two steps, StackGAN can generate high-quality, high-resolution images that are both visually convincing and textually accurate, a significant advancement in the field of generative models.
2.2.3. AttnGAN. A sophisticated deep learning model called AttnGAN is designed to create images from textual descriptions, with an eye on improving the output images' accuracy and detail. Using an attention mechanism, AttnGAN differs from other GAN-based models in that it enables the model to choose focus on particular textual elements when producing associated image segments. This dynamic attention ensures that the generated image is more finely tuned to the semantics of the text, resulting in a closer alignment between the visual output and the descriptive input.
The architecture of AttnGAN builds upon the multi-stage image generation process found in models like StackGAN but incorporates additional refinements. Similar to StackGAN, AttnGAN separates the generating process into several steps, each of which gradually improves the image's quality from a rough, low-resolution form to an output that is extremely detailed and high-resolution. The initial stage generates a basic image that captures the overall structure of the object described in the text, while subsequent stages refine the image by adding layers of detail. To guarantee that the refinement is constant and ongoing, the output from one generator is utilized as the input for the next at each stage.
AttnGAN's attention mechanism is one of its main innovations. Through this approach, the model can generate distinct sections of the image by dynamically targeting different parts of the text. For example, the model can focus more on the portion of the description that describes the shape, colors, and details of the bird's body when creating the bird's body. When moving on to generate the wings, it shifts its focus to the words describing the wings, such as "feathered" or "wide-spread." This enables the model to generate more semantically meaningful images, as the attention mechanism helps ensure that each part of the image is aligned with the corresponding part of the text. As a result, AttnGAN excels at generating images that capture both fine-grained details and global coherence.
Moreover, AttnGAN's ability to focus on relevant words during the generation process gives it an edge in generating complex and highly descriptive images. By aligning specific textual elements with corresponding visual components, AttnGAN can produce images that are not only more detailed but also more faithful to the original description. This attention-driven approach marks a significant improvement over previous GAN models, making AttnGAN particularly effective in applications where precise alignment between text and image is critical, such as generating artwork from descriptions or creating visual content based on detailed instructions.
3. Result and Discussion
3.1. Result analysis
The Inception Score (IS) is calculated by evaluating both the diversity of the generated images and their quality. A higher score indicates that the generated images are not only of better quality but also exhibit greater diversity. In the aforementioned bird dataset, StackGAN’s Inception Score is around 3.7, while AttnGAN’s Inception Score can reach 4.36, indicating that AttnGAN has an advantage in both image diversity and quality.
FID (Fréchet Inception Distance) is a tool used to assess the quality of generated images by comparing their distribution to that of real photos; the closer the generated images are to the real images, the lower their score. Experiments (show in Table 1) show that AttnGAN achieves an FID of approximately 23.98 on the CUB dataset, while StackGAN’s FID is typically around 50. This suggests that AttnGAN is superior in terms of generating images that closely resemble real images. These results may be due to StackGAN potentially overlooking some detailed descriptions when generating images. In contrast, AttnGAN, by introducing a cross-modal attention mechanism between text and images, excels at generating fine details that align with textual descriptions. This enables AttnGAN to better retain local details when generating images from text. Based on the table data, Furthermore, compared to StackGAN, it is clear that AttnGAN produces text-generated visuals that are more realistic, varied, and detailed.
Table 1. Accuracy of each method in diabetes’ prediction.
model |
Inception Score (IS) |
Fréchet Inception Distance (FID) |
StackGAN |
3.70 ± .04 |
~50 |
AttnGAN |
4.36 ± .03 |
23.98 |
3.2. Discussion
Traditional GANs serve as the foundational architecture, with the Generator and Discriminator functioning in an adversarial framework. Although GANs are excellent for producing realistic images from noise, there is a discrepancy between the visual output and semantic correctness because these models lack explicit methods that ensure that the generated images closely match the descriptions in the input text.
StackGAN introduces a multi-stage generation process, which improves upon the original GAN by progressively refining image quality across stages. This enables StackGAN to produce higher-resolution, more detailed images, which is especially useful in complex generation tasks where clarity and visual detail are paramount. StackGAN improves the realism of the images, but it still has trouble guaranteeing precise alignment between textual input and image output, particularly when it comes to extracting fine-grained information from the text. By adding an attention component, AttnGAN expands upon the StackGAN framework by enabling the model to concentrate on particular textual elements when producing related sections of the image. This invention fixes one of the main issues with previous models by considerably enhancing the semantic congruence between the generated image and the text. However, despite its improvements, AttnGAN still faces challenges in generating highly diverse images, and like its predecessors, it struggles with producing images that are consistently realistic and semantically aligned in complex scenarios.
Improving cross-modal alignment even further is crucial if author is to make generated visuals accurately represent the textual descriptions and seem realistic. Future research could explore advanced attention mechanisms, such as multi-level cross-modal attention or multimodal alignment techniques, to enhance this consistency. Such innovations would allow for more precise control over the generated image and could potentially lead to models that better capture the intricate relationships between text and visual content.
4. Conclusion
This study focused on comparing the performance of StackGAN and AttnGAN in the context of text-to-image generation, with a particular emphasis on image quality, detail alignment, and realism. Using the CUB-200-2011 bird image dataset, various metrics were employed to evaluate both models extensively. The experimental results demonstrated that AttnGAN consistently outperforms StackGAN, particularly in aligning generated image details with the input text and producing more realistic and coherent images. Even if AttnGAN provides notable gains in visual fidelity and semantic consistency, both the quality and diversity of the generated images can still be improved. Addressing these challenges will be essential for the continued development of this field. Future research may focus on refining attention mechanisms, improving cross-modal alignment, and developing more accurate evaluation metrics tailored to the specific needs of text-to-image generation. It is anticipated that as these developments take place, the text-to-image generating area will develop, providing more advanced and adaptable models for a variety of applications.
References
[1]. Goodfellow I Pouget-Abadie J Mirza M et al. (2014). Generative adversarial net. Advances in neural information processing systems, 27
[2]. Reed S Akata Z Yan X et al. (2016). Generative adversarial text to image synthesis. International conference on machine learning, 1060-1069
[3]. Denton E L Chintala S Fergus R. (2015). Deep generative image models using laplacian pyramid of adversarial networks. Advances in neural information processing systems, 28
[4]. Radford A. (2015). Unsupervised representation learning with deep convolutional generative adversarial networks. arXiv preprint 1511.06434
[5]. Zhang H Xu T Li H et al. (2017). Stackgan: Text to photo-realistic image synthesis with stacked generative adversarial networks. Proceedings of the IEEE international conference on computer vision, 5907-5915
[6]. Reed S Akata Z Yan X et al. (2016). Generative adversarial text to image synthesis. International conference on machine learning, 1060-1069
[7]. Reed S E Akata Z Mohan S et al. (2016). Learning what and where to draw. Advances in neural information processing systems, 29
[8]. Fang H Gupta S Iandola F et al. (2015). From captions to visual concepts and back. Proceedings of the IEEE conference on computer vision and pattern recognition, 1473-1482
[9]. Xu K Ba J Kiros R et al. (2015). Show, attend and tell: Neural image caption generation with visual attention. International conference on machine learning, 2048-2057
[10]. Xu T Zhang P Huang Q et al. (2018). Attngan: Fine-grained text to image generation with attentional generative adversarial networks. Proceedings of the IEEE conference on computer vision and pattern recognition, 1316-1324
Cite this article
Wang,R. (2024). A Comparative Analysis of StackGAN and AttnGAN in Text-to-Image Generation. Applied and Computational Engineering,105,9-15.
Data availability
The datasets used and/or analyzed during the current study will be available from the authors upon reasonable request.
Disclaimer/Publisher's Note
The statements, opinions and data contained in all publications are solely those of the individual author(s) and contributor(s) and not of EWA Publishing and/or the editor(s). EWA Publishing and/or the editor(s) disclaim responsibility for any injury to people or property resulting from any ideas, methods, instructions or products referred to in the content.
About volume
Volume title: Proceedings of CONF-MLA 2024 Workshop: Neural Computing and Applications
© 2024 by the author(s). Licensee EWA Publishing, Oxford, UK. This article is an open access article distributed under the terms and
conditions of the Creative Commons Attribution (CC BY) license. Authors who
publish this series agree to the following terms:
1. Authors retain copyright and grant the series right of first publication with the work simultaneously licensed under a Creative Commons
Attribution License that allows others to share the work with an acknowledgment of the work's authorship and initial publication in this
series.
2. Authors are able to enter into separate, additional contractual arrangements for the non-exclusive distribution of the series's published
version of the work (e.g., post it to an institutional repository or publish it in a book), with an acknowledgment of its initial
publication in this series.
3. Authors are permitted and encouraged to post their work online (e.g., in institutional repositories or on their website) prior to and
during the submission process, as it can lead to productive exchanges, as well as earlier and greater citation of published work (See
Open access policy for details).
References
[1]. Goodfellow I Pouget-Abadie J Mirza M et al. (2014). Generative adversarial net. Advances in neural information processing systems, 27
[2]. Reed S Akata Z Yan X et al. (2016). Generative adversarial text to image synthesis. International conference on machine learning, 1060-1069
[3]. Denton E L Chintala S Fergus R. (2015). Deep generative image models using laplacian pyramid of adversarial networks. Advances in neural information processing systems, 28
[4]. Radford A. (2015). Unsupervised representation learning with deep convolutional generative adversarial networks. arXiv preprint 1511.06434
[5]. Zhang H Xu T Li H et al. (2017). Stackgan: Text to photo-realistic image synthesis with stacked generative adversarial networks. Proceedings of the IEEE international conference on computer vision, 5907-5915
[6]. Reed S Akata Z Yan X et al. (2016). Generative adversarial text to image synthesis. International conference on machine learning, 1060-1069
[7]. Reed S E Akata Z Mohan S et al. (2016). Learning what and where to draw. Advances in neural information processing systems, 29
[8]. Fang H Gupta S Iandola F et al. (2015). From captions to visual concepts and back. Proceedings of the IEEE conference on computer vision and pattern recognition, 1473-1482
[9]. Xu K Ba J Kiros R et al. (2015). Show, attend and tell: Neural image caption generation with visual attention. International conference on machine learning, 2048-2057
[10]. Xu T Zhang P Huang Q et al. (2018). Attngan: Fine-grained text to image generation with attentional generative adversarial networks. Proceedings of the IEEE conference on computer vision and pattern recognition, 1316-1324