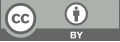
Mitigating Bias in Large Language Models: A Multi-Task Training Approach Using BERT
- 1 Department of Electrical and computer engineering, University of California, Santa Barbara, California, USA
* Author to whom correspondence should be addressed.
Abstract
Large language models (LLMs), such as ChatGPT, have become essential tools due to their advanced natural language processing capabilities. However, these models, trained on extensive internet text, can inadvertently learn and propagate unwanted biases, impacting their outputs. This study addresses this issue by analyzing and mitigating such biases through a multi-task and multi-stage training approach. Utilizing the Winograd Bias (Winobias) dataset, the research fine-tunes the Bidirectional Encoder Representations from Transformers (BERT) model to reduce biased outputs. The approach includes an initial mask task to establish a general understanding and a subsequent cloze task to specifically target and mitigate biases. Results demonstrate a significant reduction in bias, with the original model showing approximately 90% certainty in biased outputs, whereas the de-biased model reduced this certainty to 55%. This study effectively showcases a method for bias reduction by modifying only a few parameters, emphasizing a practical approach to enhancing fairness and balance in LLMs used across various applications.
Keywords
Winobias, Large Language Models, Multi-Stage Training, BERT.
[1]. Bender E M Gebru T McMillan-Major A et al. (2021) On the dangers of stochastic parrots: Can language models be too big?. Proceedings of ACM conference on fairness, accountability, and transparency, 610-623
[2]. Gallegos I O Rossi R A Barrow J et al. (2024) Bias and fairness in large language models: A survey. Computational Linguistics, 1-79
[3]. Zhao J Wang T Yatskar M et al. (2018) Gender bias in coreference resolution: Evaluation and debiasing methods. arXiv preprint 1804.06876
[4]. Kusner M J Loftus J Russell C et al. (2017) Counterfactual fairness. Advances in neural information processing systems, 30
[5]. Zafar M B Valera I Rogriguez M G et al. (2017) Fairness constraints: Mechanisms for fair classification. Artificial intelligence and statistics, 962-970
[6]. Cheng L Kim N Liu H. (2022) Debiasing word embeddings with nonlinear geometry. arXiv preprint 2208.13899
[7]. Kamruzzaman M Kim G L. (2024). Prompting techniques for reducing social bias in llms through system 1 and system 2 cognitive processes. arXiv preprint 2404.17218
[8]. Xu L Xie H Qin S Z J et al. (2023). Parameter-efficient fine-tuning methods for pretrained language models: A critical review and assessment. arXiv preprint 2312.12148
[9]. Houlsby N Giurgiu A Jastrzebski S et al. (2019). Parameter-efficient transfer learning for NLP. International conference on machine learning, 2790-2799
[10]. Devlin J. (2018). Bert: Pre-training of deep bidirectional transformers for language understanding. arXiv preprint 1810.04805
Cite this article
Chen,S. (2024). Mitigating Bias in Large Language Models: A Multi-Task Training Approach Using BERT. Applied and Computational Engineering,105,30-37.
Data availability
The datasets used and/or analyzed during the current study will be available from the authors upon reasonable request.
Disclaimer/Publisher's Note
The statements, opinions and data contained in all publications are solely those of the individual author(s) and contributor(s) and not of EWA Publishing and/or the editor(s). EWA Publishing and/or the editor(s) disclaim responsibility for any injury to people or property resulting from any ideas, methods, instructions or products referred to in the content.
About volume
Volume title: Proceedings of CONF-MLA 2024 Workshop: Neural Computing and Applications
© 2024 by the author(s). Licensee EWA Publishing, Oxford, UK. This article is an open access article distributed under the terms and
conditions of the Creative Commons Attribution (CC BY) license. Authors who
publish this series agree to the following terms:
1. Authors retain copyright and grant the series right of first publication with the work simultaneously licensed under a Creative Commons
Attribution License that allows others to share the work with an acknowledgment of the work's authorship and initial publication in this
series.
2. Authors are able to enter into separate, additional contractual arrangements for the non-exclusive distribution of the series's published
version of the work (e.g., post it to an institutional repository or publish it in a book), with an acknowledgment of its initial
publication in this series.
3. Authors are permitted and encouraged to post their work online (e.g., in institutional repositories or on their website) prior to and
during the submission process, as it can lead to productive exchanges, as well as earlier and greater citation of published work (See
Open access policy for details).