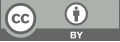
Data mining in AI: Evolution, applications, and future directions
- 1 The University of Queensland
* Author to whom correspondence should be addressed.
Abstract
This paper provides a comprehensive analysis of the evolution and impact of data mining in the field of artificial intelligence (AI), with a particular focus on its application within social and information networks. It traces the origins of AI back to the 1956 Dartmouth Conference, highlighting the subsequent advancements in technologies such as machine learning and data mining that have fueled AI's growth. The paper explores the multifaceted applications of data mining in various sectors including healthcare, transportation, and industrial manufacturing, and delves into the challenges and innovations in recommendation systems, matrix factorization, and intelligent control of autonomous vehicles in intelligent transportation systems. The study emphasizes the significance of distributed algorithms and big data processing frameworks in enhancing the efficiency and applicability of data mining techniques.
Keywords
Artificial Intelligence, Data Mining, Machine Learning, Distributed Algorithms, MapReduce Framework.
[1]. Ageed, Zainab Salih, et al. "Comprehensive survey of big data mining approaches in cloud systems." Qubahan Academic Journal 1.2 (2021): 29-38.
[2]. Stephany, Fabian, et al. "The CoRisk-Index: A data-mining approach to identify industry-specific risk assessments related to COVID-19 in real-time." arXiv preprint arXiv:2003.12432 (2020).
[3]. Van Nguyen, Truong, et al. "Predicting customer demand for remanufactured products: A data-mining approach." European Journal of Operational Research 281.3 (2020): 543-558.
[4]. Greener, Joe G., et al. "A guide to machine learning for biologists." Nature Reviews Molecular Cell Biology 23.1 (2022): 40-55.
[5]. Jo, Taeho. "Machine learning foundations." Supervised, Unsupervised, and Advanced Learning. Cham: Springer International Publishing (2021).
[6]. Mahesh, Batta. "Machine learning algorithms-a review." International Journal of Science and Research (IJSR).[Internet] 9.1 (2020): 381-386.
[7]. Janiesch, Christian, Patrick Zschech, and Kai Heinrich. "Machine learning and deep learning." Electronic Markets 31.3 (2021): 685-695.
[8]. Murphy, Kevin P. Probabilistic machine learning: an introduction. MIT press, 2022.
Cite this article
Wu,Z. (2024). Data mining in AI: Evolution, applications, and future directions. Applied and Computational Engineering,104,1-6.
Data availability
The datasets used and/or analyzed during the current study will be available from the authors upon reasonable request.
Disclaimer/Publisher's Note
The statements, opinions and data contained in all publications are solely those of the individual author(s) and contributor(s) and not of EWA Publishing and/or the editor(s). EWA Publishing and/or the editor(s) disclaim responsibility for any injury to people or property resulting from any ideas, methods, instructions or products referred to in the content.
About volume
Volume title: Proceedings of the 2nd International Conference on Machine Learning and Automation
© 2024 by the author(s). Licensee EWA Publishing, Oxford, UK. This article is an open access article distributed under the terms and
conditions of the Creative Commons Attribution (CC BY) license. Authors who
publish this series agree to the following terms:
1. Authors retain copyright and grant the series right of first publication with the work simultaneously licensed under a Creative Commons
Attribution License that allows others to share the work with an acknowledgment of the work's authorship and initial publication in this
series.
2. Authors are able to enter into separate, additional contractual arrangements for the non-exclusive distribution of the series's published
version of the work (e.g., post it to an institutional repository or publish it in a book), with an acknowledgment of its initial
publication in this series.
3. Authors are permitted and encouraged to post their work online (e.g., in institutional repositories or on their website) prior to and
during the submission process, as it can lead to productive exchanges, as well as earlier and greater citation of published work (See
Open access policy for details).