1. Introduction
Recently, personalized text generation has become increasingly crucial across various applications, from customer service bots to personalized content creation in today’s digital age. Generating personalized text largely enhances user experience by aligning with individual user preferences, styles, and needs. The key value of personalized text generation lies in its ability to create more natural and engaging interactions. By tailoring responses according to individual user data like user-specific features and user identity, large language models can offer more relevant and context-aware content, which can improve user satisfaction and effectiveness in tasks such as recommendation systems [1], sentiment classification, and interactive storytelling. This shift towards more user-centric systems reflects the growing demand for models that can anticipate user needs and preferences. Thus, this has led to a surge in research focused on adapting and enhancing large language models to cater to individual differences.
The Transformer model currently stands as the most prominent framework for text generation in Natural Language Processing (NLP) due to its exceptional ability to handle a wide range of language tasks [2]. However, despite its capabilities, standard Transformer models generally treat everything equally that do not account for individual user characteristics [3]. To address this limitation, recent studies have explored techniques such as fine-tuning on user-specific data and integrating user embeddings directly into the model architecture. For instance, Li, Xinyu et al. [4] leveraged data from user profiles to enhance personalized model performance by integrating personalized user representations. Furthermore, Lee, H. Y. et al. [5] used a social network-based framework to personalize Recurrent Neural Networks (RNNs). They showed that user context can improve personalized language modeling in dialogue systems when through social networks.
In addition, studies have focused on better representing users' diverse interests and behaviors within models. Welch, C. et al. [6] leveraged user similarity to enhance personalization in language models by integrating user behavior across similar profiles. It can also be effective for improving performance with limited data. Further efforts have emphasized optimizing user representations to improve personalization. Wang, B. et al. [7] used personalized embeddings based on user-item interactions like rating matrix to predict user preferences in reviews. Cao, X. et al. [8] also explored injecting user identity into pre-trained models to improve document-level sentiment classification, reinforcing the need for deeper user integration in NLP models. Moreover, King, M., & Cook, P. [9] emphasized the role of personalized models in enhancing text generation tasks by using minimal training data, which is critical for large-scale user bases.
Despite these advancements, challenges remain, particularly in adapting to new users and scaling effectively with large user bases that have limited data. Many current approaches rely on substantial amounts of user data to achieve meaningful personalization, which is not always practical. Therefore, this paper proposes an enhanced Transformer model architecture for personalized text generation. The research focuses on improving text coherence and personalization by incorporating user-specific features, such as post count and average score, alongside user embeddings. Additionally, the model architecture includes bidirectional LSTM layers and a self-attention mechanism to capture more complex relationships in the input data, allowing for better context awareness and adaptability.
To achieve this, the baseline and improved model used in this paper are extended with these additional components, and both models are trained and evaluated on a large Reddit dataset to compare their performance. The goal is to create a model that not only personalizes text generation by incorporating user-specific behavior but also produces more coherent and contextually appropriate outputs. By addressing these challenges, this work aims to improve the robustness of personalized text generation while balancing the complexity and scalability required for handling diverse user bases with limited data availability.
2. Methodology
This section outlines the architecture and implementation details of both the baseline and improved models. The architecture of the model is inspired by Huang, X et al. [10]. They proposed a multitask learning framework that adapts user embeddings by accounting for variations in users' language across different domains, such as movies and products. It can influence the meaning and sentiment of text. Two models used in this study learned from the user embeddings and the multitask learning framework in Huang’s article and was designed to leverage specific features of the dataset to enhance text generation capabilities. The goal is to generate personalized text using user-specific and subreddit-specific features derived from Reddit data. The models were implemented using TensorFlow and Keras libraries, allowing for flexible design and efficient training.
2.1. Baseline Model
The baseline model follows a standard sequence-to-sequence architecture commonly used in text generation tasks. It employs a simple Long Short-Term Memory (LSTM) network to process input sequences since LSTMs are well-suited for this task due to their ability to maintain information over long sequences and capture long-term dependencies, reducing issues like vanishing gradients.
The model takes three key inputs: the user ID, subreddit ID, and the post text.
• Input Layers:
User Input: This layer takes a single integer representing the encoded user ID. The user ID is encoded using a LabelEncoder to convert categorical data into numerical form, which the model can process.
Subreddit Input: Similar to the user input, this layer accepts a single integer representing the encoded subreddit ID. This input helps the model learn patterns specific to different subreddit communities.
Post Input: A sequence of integers representing the tokenized and padded post text. The text is preprocessed, tokenized into words, and then converted into sequences of integers. These sequences are padded to a uniform length, 100 words, to ensure consistent input size.
For embedding layers, user IDs and subreddit IDs are each mapped into a 50-dimensional embedding space, thereby allowing the model to learn user-specific and subreddit-specific patterns. Next, the post text is tokenized and converted into sequences of integers, which are then processed by a 100-dimensional text embedding layer. Subsequently, the 128 units LSTM layer processes the embedded text sequence, effectively capturing important patterns and dependencies in the data. Then, the outputs from the LSTM are concatenated with flattened user and subreddit embeddings to integrate user information into the text generation process. Finally, the model uses a dense layer with a SoftMax activation function, which produces probability distributions over the vocabulary for each position in the sequence to predict the next word based on the learned patterns. The model architecture can be found in Figure 1.
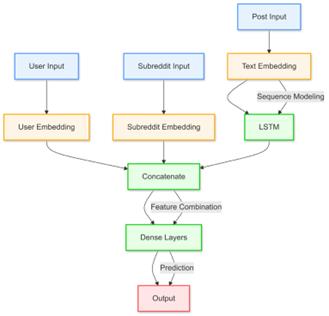
Figure 1. Baseline Model Architecture
This basic architecture serves as the foundation for generating text by focusing on the input sequence's context, but it lacks mechanisms to account for user-specific behavior dynamically or handle the bidirectional flow of information in the text.
2.2. Improved Model
The improved model builds upon the baseline by incorporating advanced architectural changes to better capture user-specific behavior and subreddit context. The core enhancement is the addition of Bidirectional LSTM layers, which process text input sequences in both forward and backward directions, allowing the model to capture dependencies from both past and future contexts. After applying the Bidirectional LSTM layers twice, a self-attention layer is added to ensure the model can focus on the most important parts of the sequence. The framework of this core enhancement is illustrated below in Figure 2.
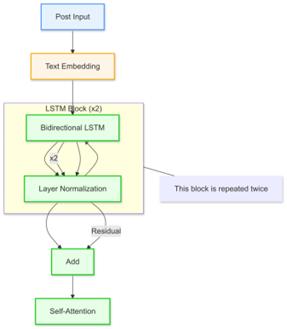
Figure 2. Text Embedding Framework
Figure 2 shows that this architecture includes multiple stacked Bidirectional LSTM layers and residual connections. These added features can help the model retain information over long sequences and improve learning stability. After the Bidirectional LSTM layers, a self-attention mechanism is applied to focus on the most relevant parts of the input sequence. It can further refine the model’s ability to generate coherent and context-aware text.
Besides that, the improved model adds an additional input layer that accepts a vector of two floats representing the user’s, post count and average score. These features provide the model with additional context about the user's activity and typical post performance, enhancing personalization. Then, embeddings for users, subreddits, and text are expanded to 256 dimensions to capture more intricate relationships in the data. User-specific features, post count and average score, are integrated alongside user and subreddit embeddings. Afterwards, these embeddings are flattened and concatenated with the text embedding outputs shown above, and then repeated across the sequence length to ensure that user and subreddit features are available at each time step of text generation. The whole improved model architecture can be found below in Figure 3.
The model also employs a deep feedforward network with dense layers, each followed by Layer Normalization and Dropout (rate of 0.3) to prevent overfitting and stabilize training. By incorporating residual connections between the dense layers, the model ensures that learned patterns are preserved as it becomes deeper. The final output layer uses a softmax activation to predict the next word in the sequence, and a custom loss function is employed to mask out padding tokens, ensuring that only meaningful parts of the sequence contribute to the loss calculation.
This improved architecture is designed to better understand the relationships between users, subreddits, and text, enabling more personalized and contextually relevant text generation. Through the use of Bidirectional LSTMs, self-attention, and residual connections, the model captures more nuanced patterns in the data, leading to higher-quality outputs.
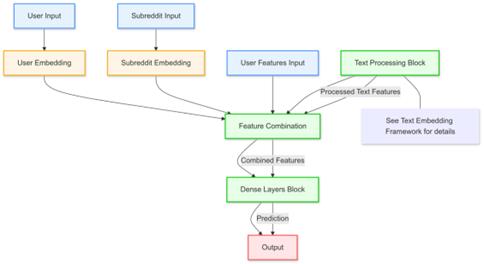
Figure 3. Improved Model Architecture
2.3. Training Process
Both the baseline and improved models were trained using similar strategies, with well-defined hyperparameters and training strategies. The primary goal of training was to optimize both models to effectively learn patterns from the input data while minimizing overfitting.
For both models, the Adam optimizer was used with a learning rate of 0.001. Adam's ability to adapt the learning rate based on gradient moments made it an ideal choice for faster convergence and more stable training. The loss function used for both models was sparse categorical cross entropy, which is suitable for multi-class classification tasks like text generation, where the model predicts the next word from a large vocabulary.
In the baseline model, the dataset was split into training and validation sets, and a batch size of 320 was used to balance between training speed and memory efficiency since the dataset contains 20,000 data. Data generators were implemented to feed the model with user IDs, subreddit IDs, and post sequences. The model was trained for 20 epochs, ensuring enough iterations to capture the underlying patterns. A standard accuracy metric was used to track performance.
For the improved model, the training process was more complex due to the inclusion of additional user features and a larger architecture. The dataset was again split into training and validation sets, but with a reduced batch size of 32 to accommodate the increased computational requirements. A custom data generator was implemented to include user features (post count and average score) alongside user IDs, subreddit IDs, and post sequences. The model was still trained for up to 20 epochs. Additionally, a masked loss function was used to handle padding tokens during training, ensuring that only meaningful tokens contributed to the loss calculation. The improved model employed a custom accuracy metric (masked_accuracy) to account for the masking of padded tokens.
2.4. Callbacks
To prevent overfitting and optimize the training of the improved model, two key callbacks were employed:
• EarlyStopping: This callback monitored the validation loss and halted training if no improvement was observed after 5 epochs. This approach ensured that the model stopped training once it had adequately learned from the data, avoiding unnecessary overfitting.
• ReduceLROnPlateau: This callback reduced the learning rate by a factor of 0.5 when the validation loss plateaued for 3 consecutive epochs. By lowering the learning rate when needed, the model could make finer adjustments to help itself converge more finely during training. It can lead to improved performance without drastic updates that could disrupt the learning process.
The training process for the improved model was more computationally intensive due to the use of higher-dimensional embeddings, bidirectional LSTM layers, and self-attention mechanisms. These additions required more memory and longer training times, but they can capture richer representations and generate more personalized text.
2.5. Text Generation
The text generation process begins by initializing the sequence with an initial input, which could be a specific prompt or a randomly chosen starting word (e.g., "<start>"). This initial sequence provides the model with a context to start generating text, guiding the first prediction based on the context provided. Once initialized, the model predicts the probability distribution for the next word in the sequence. This prediction leverages the learned patterns from the training data and incorporates the context of the provided user and subreddit information to generate relevant text.
After predicting the probability distribution, the model samples the next word from this distribution. Sampling introduces variability and creativity into the generated text by allowing the selection of words based on probability rather than always choosing the most likely word. This approach results in more diverse and interesting outputs. After generating the new word, the input sequence is updated to include this newly generated word, ensuring that the model takes into account the entire context of the generated text when predicting subsequent words. The text generation process repeats these steps, predicting, sampling, and appending words one at a time until an end token is generated or a predefined maximum length is reached.
For the improved model, the text generation process is further personalized by incorporating user features, such as post_count and avg_score, in addition to user and subreddit IDs. These additional features provide the model with deeper insights into user behavior and preferences, potentially leading to more contextually relevant and personalized text outputs. By leveraging these enriched inputs, the improved model can generate text that better aligns with individual user characteristics and content engagement patterns.
3. Experimental results
3.1. Dataset
The choice of datasets was determined based on its size, availability, and the unique features it offered for the training process. This paper opted to train the model using Reddit Posts Dataset to facilitate learning within the model architecture, which included metadata such as post timestamps, author information, subreddit categories, and post content. The key features in this dataset include:
Table 1. Dataset key features
Features |
Meaning |
created_timestamp |
The time when the post was created |
subreddit |
The specific community where the post was made |
title |
The title of the post |
id |
A unique identifier for each post |
author |
The username of the post creator |
score |
The net upvotes received by the post |
num_comments |
The number of comments on the post |
post |
The main content of the post |
To further enhance the model's ability to generate personalized text, the paper extracted additional user-specific features from the dataset in the improved model:
• Post Count (post_count): It represents the total number of posts made by a user, which helps capture the user's activity level on the platform.
• Average Score (avg_score): The mean score of all posts made by a user represents the typical engagement or popularity of users’ content.
By incorporating these user-specific features into the dataset, this paper aimed to provide the model with deeper insights into individual user behaviors and preferences. This enhancement allows the model to generate more personalized and contextually relevant text by understanding each user's typical engagement patterns and activity level.
3.2. Preprocess dataset
The data preprocessing pipeline was designed to prepare the Reddit dataset for model training. It ensures high data quality and enhances the model’s ability to learn from user-specific and subreddit-specific features. The preprocessing process involved multiple steps aimed at cleaning the data and making it suitable for machine learning tasks.
3.3. Data and Text Preprocessing
First, rows with missing values in the 'post' column were removed to maintain data quality and ensure that all text data used in training was complete and valid. Then, a custom preprocess_text function was applied to the text data to standardize and clean it. This function first converted all text to lowercase to ensure uniformity, thus treating words like "Apple" and "apple" as the same token. Next, a regular expression was used to remove any non-word characters, such as punctuation, ensuring that the model focused solely on actual words and phrases. Additionally, stopwords—common words like "and," "the," and "is"—were removed using NLTK’s stopword list because these words are generally less informative for the model. Finally, each word was lemmatized using NLTK's WordNetLemmatizer, which reduced words to their base or dictionary form. This step helped to reduce the diversity of the vocabulary by combining different forms of a word, such as "running," "ran," and "runs," into a single token, "run."
3.4. Tokenization
After text preprocessing, the cleaned text was tokenized into sequences of integers using Keras' Tokenizer. This step converted each word into a unique integer identifier based on its frequency of occurrence in the dataset. Then, this paper chose a vocabulary size of 20,000 to capture the most frequently used. Next step is to add special tokens to the vocabulary: <UNK> for unknown words, <start> for the beginning of sequences, and <end> for the end of sequences. These tokens ensured that unique cases within the text data were handled appropriately during training.
3.5. Sequence Padding
To standardize the input size for the model, all tokenized sequences were padded to a maximum length of 100 words. This padding ensured that each input to the model had a consistent shape, regardless of the original length of the text data, which is essential for batch processing and efficient model training.
3.6. Feature Engineering
For the baseline model, the 'author' and 'subreddit' columns were encoded into numeric values using LabelEncoder. This encoding allows the model to learn basic user and subreddit behaviors.
For the improve Model, to further enhance the model's ability to generate personalized content, additional user-specific features were engineered. Two key features were added: Post Count (post_count), representing the total number of posts made by a user, and Average Score (avg_score), which calculated the mean score of all posts made by a user. These features were then standardized using StandardScaler to ensure that they had a mean of zero and a standard deviation of one. This normalization helped improve the model’s convergence during training by making the features comparable and reducing the impact of outliers.
3.7. Results
The performance of both the baseline and improved models was evaluated using training and validation accuracy metrics over multiple epochs. The generated text samples were analyzed to assess the coherence and relevance of the content produced by each model.
Baseline Model Performance: The baseline model was trained using a simple architecture that included only basic user and subreddit embeddings without incorporating any additional user-specific features. The training and validation accuracy and loss of the baseline model are shown in Figure 4.
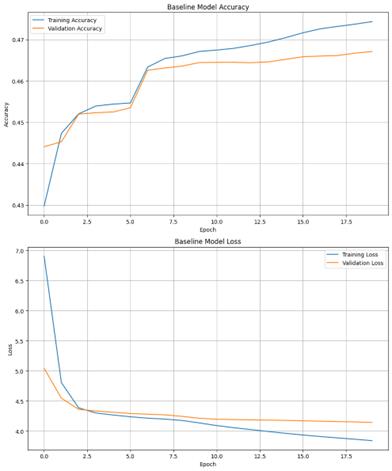
Figure 4. Baseline Model Accuracy and Loss
The training accuracy steadily increased throughout the training epochs, starting from around 0.43 and reaching approximately 0.47 by the end of the training period. The validation accuracy followed a similar trend, improving over time but starting to plateau at around epoch 8. By the end of the training process, the validation accuracy hovered around 0.46, slightly lower than the training accuracy, indicating a potential sign of the model approaching overfitting. The gap between training and validation accuracy, though small, suggests that the model may struggle with generalization beyond a certain point.
On the training loss plot, both the training and validation losses rapidly decreased during the initial epochs, with the training loss dropping from around 7.0 to 4.0 and the validation loss following a similar trend. By epoch 8, the loss curves start to level off, which indicates that the model has learned the majority of patterns in the training data. However, the continuous decline in training loss, compared to the flatter trend of the validation loss, indicates that the model is likely learning to fit the training data too closely, which may not translate well to unseen data.
In terms of text generation, the text generated by the baseline model is as follows: "<start> today I learned good job offer close across many ml industry job suggestion industry may better research ml folk really good research ml thanks feedback thought thanks reading http www linkedin com feed iclr 2022 amp e <UNK> amp <UNK> amp <UNK> amp <UNK> amp e <UNK> amp tn <UNK> amp <UNK> <UNK> amp p <UNK> deep learning ucl new research help subject might realistic anyone experience research industry ml research ml absence opportunity thinking much better ml dl many industry industry research industry research amp industry suggestion may relevant job would much much think many relevant post want switch phd researcher opinion."
This output shows that the model can create sentences that are mostly grammatically correct, but it struggles with making the text flow logically and staying on topic. The generated text has a lot of repetition, with phrases being repeated unnecessarily, which makes it sound confusing and less coherent. Additionally, the text contains several "<UNK>" tokens, which are placeholders for words the model did not recognize or could not generate. This suggests that the model has difficulty understanding the context and producing relevant and meaningful content. Overall, while the model can form sentences, it doesn't effectively connect ideas or maintain a clear focus throughout the text.
Improved Model Performance: The improved model introduced additional user-specific features, such as ‘post_count’ and ‘avg_score’, embedded along with the original user and subreddit embeddings. This enhancement aimed to provide the model with more detailed insights into user behavior and content engagement patterns. The accuracy and loss of the improved model is depicted in Figure 5.
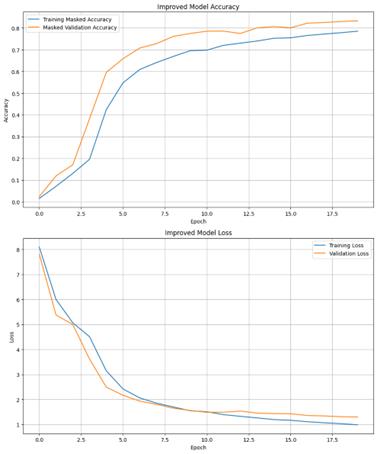
Figure 5. Improved Model Accuracy and Loss
The training accuracy for the improved model showed a rapid increase within the first few epochs, reaching nearly 0.6 by around epoch 4. This sharp rise suggests that the model quickly adapted to the training data, likely due to the richer input features and more complex architecture, including user-specific features and subreddit embeddings. The validation accuracy followed a similar pattern, quickly surpassing the training accuracy and peaking at around 0.83 by epoch 20. After this point, both the training and validation accuracies began to plateau, indicating that the model had effectively learned the patterns in the data. The close alignment between training and validation accuracy throughout training suggests that the model generalized well and avoided significant overfitting.
The training loss curve shows a steady decline, starting from around 8.0 and dropping to approximately 1.0 by the final epoch. The validation loss follows a nearly identical trend, indicating that the model was learning effectively across both the training and validation sets. The fact that both curves converge and show similar rates of decline reinforces the notion that the improved model managed to avoid overfitting, balancing well between training on the given data and generalizing to new inputs.
In terms of text generation, the text generated by the improved model is as follows: "<start> today i learned debug firstly historical compression avail staging modeled tuned mountain tensorboard leveraging scheme mountain rated firstly adder guaranteed mounted mountain landing ssd guaranteed obstacle united eliminate sat downloading historic sat illustrate standardize stitch compression geometry standardize firstly ssd mountain historical allowed eliminate sat orthogonal smoothly availability firstly fft firstly orthogonal."
This output showed that despite the improved accuracy metrics, the generated text output reveals some limitations. The text produced by the model, while more structured, appears incoherent and is filled with non-contextual technical terms. For example, phrases like "debug firstly historical compression avail" and "mountain landing ssd guaranteed obstacle" do not form coherent sentences, suggesting that the model has learned to mimic patterns of language but struggles with meaningful content generation.
Compared to the baseline model, the words generated by the improved model are notably more complex and technical. While the baseline model generated simpler and more repetitive text with filler words, the improved model appears to focus more on generating a variety of complex words in sentences. This difference indicates that the improved model may be overfitting to the individual words and patterns in the training data, prioritizing word-level complexity over contextual or sentence-level coherence. As a result, while the improved model can produce richer and more diverse vocabulary, it struggles to generate coherent and contextually relevant content.
4. Discussion
The results show a clear distinction between the baseline and improved models, particularly in terms of accuracy and the coherence of the generated text. The baseline model employed a straightforward sequence-to-sequence architecture. It used a single LSTM layer, which combined with user and subreddit embeddings. This model demonstrated moderate accuracy in both training and validation, but its generated text lacked coherence and frequently repeated words and phrases. It struggled to maintain logical flow and contextual relevance, often resorting to placeholders (<UNK>) for words it could not generate.
The improved model introduced several architectural enhancements, including Bidirectional LSTM layers, attention mechanisms, and additional user-specific features such as post count and average score. These features helped the model capture more complex patterns in the data and personalize text generation for individual users. The introduction of residual connections and larger embedding spaces allowed the model to leverage richer input data, leading to a notable increase in both training and validation accuracy. It peaks at 0.83 in validation accuracy. Additionally, the use of self-attention also improved the model’s ability to focus on important parts of the input sequence, theoretically enhancing context-awareness.
Despite the higher accuracy and technical improvements, the generated text still presented challenges. The model often overfitted on certain technical terms and generate complex but incoherent words. This suggests that while the model successfully learned to represent complex linguistic patterns and user-specific features, it struggled to balance between word-level complexity and sentence-level coherence. While the words generated by the improved model were more sophisticated than the baseline, the sentences still lacked meaning or logical structure. This could indicate that the model focused too much on learning individual word patterns at the expense of understanding contextual relationships between words.
Several future directions can be pursued to address these challenges. One key area of improvement is the introduction of stronger regularization techniques. Methods such as dropout, L2 regularization, or batch normalization could be employed to help reduce overfitting and encourage the model to learn more generalized patterns.
Another direction involves adjusting the feature representations used in the model. Adding more additional user-specific and subreddit-specific features may further enhance personalization. For instance, incorporating dynamic data, such as user engagement trends over time or subreddit activity patterns, could provide deeper insights into user behaviors. Additionally, user-specific features with contextual embeddings could be combined to improve the alignment between the user’s historical interactions and the generated content.
Refining the model architecture is also another consideration for future work. Advanced models such as transformer-based models like GPT or BERT are highly effective in text generation tasks and could be explored to improve the current model’s performance. These architectures are well-suited for handling complex input data and can generate more coherent text but still maintain the richness and complexity of the language.
5. Conclusion
Throughout this study, several techniques and architectural improvements were implemented to enhance the performance of the baseline model, resulting to more accurate predictions and richer vocabulary generation. The model started from a baseline sequence-to-sequence architecture with a single LSTM layer to a more sophisticated model, which incorporated Bidirectional LSTMs, attention mechanisms, and user-specific features. The improved model showed a significant increase in accuracy and demonstrated an ability to learn complex linguistic patterns and user-specific features. However, challenges in maintaining sentence-level coherence and avoiding overfitting remain. Future work will focus on refining the model with stronger regularization techniques, enriched feature representations, and advanced architectures such as transformers. By addressing these areas, the model aims to generate more contextually appropriate and coherent text tailored to individual users.
References
[1]. Li, L., Zhang, Y., & Chen, L. (2021). Personalized transformer for explainable recommendation. arXiv preprint arXiv:2105.11601.
[2]. Tan, Z., Zeng, Q., Tian, Y., Liu, Z., Yin, B., & Jiang, M. (2024). Democratizing large language models via personalized parameter-efficient fine-tuning. arXiv preprint arXiv:2402.04401.
[3]. Liu, Q., Qin, J., Ye, W., Mou, H., He, Y., & Wang, K. (2024). Adaptive Prompt Routing for Arbitrary Text Style Transfer with Pre-trained Language Models. Proceedings of the AAAI Conference on Artificial Intelligence, 38(17), 18689-18697.
[4]. Li, X., Lipton, Z. C., & Leqi, L. (2024). Personalized language modeling from personalized human feedback. arXiv preprint arXiv:2402.05133.
[5]. Lee, H. Y., Tseng, B. H., Wen, T. H., & Tsao, Y. (2016). Personalizing recurrent-neural-network-based language model by social network. IEEE/ACM Transactions on Audio, Speech, and Language Processing, 25(3), 519-530.
[6]. Welch, C., Gu, C., Kummerfeld, J. K., Pérez-Rosas, V., & Mihalcea, R. (2022, May). Leveraging similar users for personalized language modeling with limited data. In Proceedings of the 60th Annual Meeting of the Association for Computational Linguistics (Volume 1: Long Papers) (pp. 1742-1752).
[7]. Wang, B., Chen, B., Ma, L., & Zhou, G. (2018). User-personalized review rating prediction method based on review text content and user-item rating matrix. Information, 10(1), 1.
[8]. Cao, X., Yu, J., & Zhuang, Y. (2022). Injecting user identity into pretrained language models for document-level sentiment classification. IEEE Access, 10, 30157-30167.
[9]. King, M., & Cook, P. (2020, May). Evaluating approaches to personalizing language models. In Proceedings of the Twelfth Language Resources and Evaluation Conference (pp. 2461-2469).
[10]. Huang, X., Paul, M. J., Burke, R., Dernoncourt, F., & Dredze, M. (2021). User factor adaptation for user embedding via multitask learning. arXiv preprint arXiv:2102.11103.
Cite this article
Shu,C. (2024). Enhanced Personalized Text Generation Using User Embeddings and Attention Mechanisms. Applied and Computational Engineering,107,61-72.
Data availability
The datasets used and/or analyzed during the current study will be available from the authors upon reasonable request.
Disclaimer/Publisher's Note
The statements, opinions and data contained in all publications are solely those of the individual author(s) and contributor(s) and not of EWA Publishing and/or the editor(s). EWA Publishing and/or the editor(s) disclaim responsibility for any injury to people or property resulting from any ideas, methods, instructions or products referred to in the content.
About volume
Volume title: Proceedings of the 2nd International Conference on Machine Learning and Automation
© 2024 by the author(s). Licensee EWA Publishing, Oxford, UK. This article is an open access article distributed under the terms and
conditions of the Creative Commons Attribution (CC BY) license. Authors who
publish this series agree to the following terms:
1. Authors retain copyright and grant the series right of first publication with the work simultaneously licensed under a Creative Commons
Attribution License that allows others to share the work with an acknowledgment of the work's authorship and initial publication in this
series.
2. Authors are able to enter into separate, additional contractual arrangements for the non-exclusive distribution of the series's published
version of the work (e.g., post it to an institutional repository or publish it in a book), with an acknowledgment of its initial
publication in this series.
3. Authors are permitted and encouraged to post their work online (e.g., in institutional repositories or on their website) prior to and
during the submission process, as it can lead to productive exchanges, as well as earlier and greater citation of published work (See
Open access policy for details).
References
[1]. Li, L., Zhang, Y., & Chen, L. (2021). Personalized transformer for explainable recommendation. arXiv preprint arXiv:2105.11601.
[2]. Tan, Z., Zeng, Q., Tian, Y., Liu, Z., Yin, B., & Jiang, M. (2024). Democratizing large language models via personalized parameter-efficient fine-tuning. arXiv preprint arXiv:2402.04401.
[3]. Liu, Q., Qin, J., Ye, W., Mou, H., He, Y., & Wang, K. (2024). Adaptive Prompt Routing for Arbitrary Text Style Transfer with Pre-trained Language Models. Proceedings of the AAAI Conference on Artificial Intelligence, 38(17), 18689-18697.
[4]. Li, X., Lipton, Z. C., & Leqi, L. (2024). Personalized language modeling from personalized human feedback. arXiv preprint arXiv:2402.05133.
[5]. Lee, H. Y., Tseng, B. H., Wen, T. H., & Tsao, Y. (2016). Personalizing recurrent-neural-network-based language model by social network. IEEE/ACM Transactions on Audio, Speech, and Language Processing, 25(3), 519-530.
[6]. Welch, C., Gu, C., Kummerfeld, J. K., Pérez-Rosas, V., & Mihalcea, R. (2022, May). Leveraging similar users for personalized language modeling with limited data. In Proceedings of the 60th Annual Meeting of the Association for Computational Linguistics (Volume 1: Long Papers) (pp. 1742-1752).
[7]. Wang, B., Chen, B., Ma, L., & Zhou, G. (2018). User-personalized review rating prediction method based on review text content and user-item rating matrix. Information, 10(1), 1.
[8]. Cao, X., Yu, J., & Zhuang, Y. (2022). Injecting user identity into pretrained language models for document-level sentiment classification. IEEE Access, 10, 30157-30167.
[9]. King, M., & Cook, P. (2020, May). Evaluating approaches to personalizing language models. In Proceedings of the Twelfth Language Resources and Evaluation Conference (pp. 2461-2469).
[10]. Huang, X., Paul, M. J., Burke, R., Dernoncourt, F., & Dredze, M. (2021). User factor adaptation for user embedding via multitask learning. arXiv preprint arXiv:2102.11103.