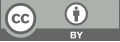
Iterative pseudo-labelling with SoftMax probability in text classification
- 1 School of Computing, National University of Singapore, Singapore, 138600
* Author to whom correspondence should be addressed.
Abstract
Semi-supervised learning is one of the potential research fields in text classification. In this paper, semi-supervised pseudo-label training experiments are conducted using the BERT model that has been pre-trained as a baseline. Only 20% of the original dataset is used for the new training set after segmenting the training set. The raw corpus used for pseudo-label training consists of the remaining 80% of data after labels are removed, while the original test set is still utilized. The results indicate that the key to the semi-supervised pseudo-labelling method is the performance of the original model and reasonable data filtering techniques. Even though the SoftMax value used for data filtering is not precisely equivalent to model prediction accuracy, experimental results show it can somewhat reduce the error propagation problem of the model. This is consistent with earlier research. However, using SoftMax as the threshold for data screening can't bring enough benefits to the model training and make it surpass the training performance of the original data set. As a result, future studies will focus on improving the accuracy of pseudo-labelling with a more suitable data selection method to better the model's performance.
Keywords
Semi-Supervised Learning, Text Classification, Softmax Probability, Deep Learning.
[1]. Kowsari, K., Jafari Meimandi, K., Heidarysafa, M., Mendu, S., Barnes, L., & Brown, D. (2019). Text classification algorithms: A survey. Information, 10(4), 150.
[2]. Xie, Q., Dai, Z., Hovy, E., Luong, T., & Le, Q. (2020). Unsupervised data augmentation for consistency training. Advances in Neural Information Processing Systems, 33, 6256-6268.
[3]. Wei, J., & Zou, K. (2019). Eda: Easy data augmentation techniques for boosting performance on text classification tasks. arXiv preprint arXiv:1901.11196.
[4]. Miyato, T., Dai, A. M., & Goodfellow, I. (2016). Adversarial training methods for semi-supervised text classification. arXiv preprint arXiv:1605.07725.
[5]. Lee, D. H. (2013, June). Pseudo-label: The simple and efficient semi-supervised learning method for deep neural networks. In Workshop on challenges in representation learning, ICML (Vol. 3, No. 2, p. 896).
[6]. Mekala, D., Dong, C., & Shang, J. (2022). LOPS: Learning Order Inspired Pseudo-Label Selection for Weakly Supervised Text Classification. arXiv preprint arXiv:2205.12528.
[7]. Chung, H., & Lee, J. (2022). Iterative Semi-Supervised Learning Using Softmax Probability. Computers, Materials and Continua, 72(3), 5607-5628.
[8]. Zhang, Y., Wang, J., & Zhang, X. (2021). Conciseness is better: recurrent attention lstm model for document-level sentiment analysis. Neurocomputing, 462, 101-112.
[9]. D’Aniello, G., Gaeta, M., & La Rocca, I. (2022). KnowMIS-ABSA: an overview and a reference model for applications of sentiment analysis and aspect-based sentiment analysis. Artificial Intelligence Review, 1-32.
[10]. Devlin, J., Chang, M. W., Lee, K., & Toutanova, K. (2018). Bert: Pre-training of deep bidirectional transformers for language understanding. arXiv preprint arXiv:1810.04805.
Cite this article
Wang,J. (2023). Iterative pseudo-labelling with SoftMax probability in text classification. Applied and Computational Engineering,6,24-29.
Data availability
The datasets used and/or analyzed during the current study will be available from the authors upon reasonable request.
Disclaimer/Publisher's Note
The statements, opinions and data contained in all publications are solely those of the individual author(s) and contributor(s) and not of EWA Publishing and/or the editor(s). EWA Publishing and/or the editor(s) disclaim responsibility for any injury to people or property resulting from any ideas, methods, instructions or products referred to in the content.
About volume
Volume title: Proceedings of the 3rd International Conference on Signal Processing and Machine Learning
© 2024 by the author(s). Licensee EWA Publishing, Oxford, UK. This article is an open access article distributed under the terms and
conditions of the Creative Commons Attribution (CC BY) license. Authors who
publish this series agree to the following terms:
1. Authors retain copyright and grant the series right of first publication with the work simultaneously licensed under a Creative Commons
Attribution License that allows others to share the work with an acknowledgment of the work's authorship and initial publication in this
series.
2. Authors are able to enter into separate, additional contractual arrangements for the non-exclusive distribution of the series's published
version of the work (e.g., post it to an institutional repository or publish it in a book), with an acknowledgment of its initial
publication in this series.
3. Authors are permitted and encouraged to post their work online (e.g., in institutional repositories or on their website) prior to and
during the submission process, as it can lead to productive exchanges, as well as earlier and greater citation of published work (See
Open access policy for details).