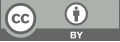
Emotion Classification through Song Lyrics in Multi-Languages with Bert
- 1 McLean High School, McLean, 22101, United States
- 2 Jinling High School Hexi campus, Nanjing, 210019, China
* Author to whom correspondence should be addressed.
Abstract
This research explores emotion classification in song lyrics using BERT models for multi-language datasets, focusing on English and Chinese lyrics. The study emphasizes the application of music therapy techniques by utilizing song lyrics to assist clients in expressing and processing emotions. Sentiment analysis is conducted through a combination of CNN, LSTM, and GRU models, with a GRU + CNN hybrid model demonstrating enhanced performance in multilingual contexts. A comprehensive preprocessing process, including translation processing and tokenization, enables the effective analysis of both English and Chinese lyrics. Experimental results indicate that the GRU + CNN model outperforms traditional models, particularly in cross-lingual sentiment analysis, achieving significant improvements in accuracy and emotional classification.
Keywords
Emotion classification, BERT, music therapy, multilingual sentiment analysis, deep learning, song lyrics
[1]. Cleveland Clinic. “Music Therapy: What Is It, Types & Treatment.” Cleveland Clinic, Cleveland Clinic, 18 July 2023, my.clevelandclinic.org/health/treatments/8817-music-therapy.
[2]. Tiemann, Julie. “Music Therapy Intervention Series: Lyric Discussion.” Metro Music Makers, 25 Nov. 2019, www.metromusicmakers.com/2019/11/music-therapy-intervention-series-lyric-discussion/.
[3]. Poria, S., Gelbukh, A., Hussain, A., Bandyopadhyay, S., & Howard, N. (2013). Music Genre Classification: A Semi-supervised Approach. Mexican Conference on Pattern Recognition.
[4]. Elbir, Ahmet, and Nizamettin Aydin. 2020. “Music Genre Classification and Music Recommendation by Using Deep Learning.” Electronics Letters, March. https://doi.org/10.1049/el.2019.4202.
[5]. Ma, Xundong. 2023. “Music Genre Classification Based on Machine Learning Methods.” Highlights in Science, Engineering and Technology 34 (February): 168–75. https://doi.org/10.54097/hset.v34i.5443.
[6]. Ritika Dhyani. 2023. “Song Classification Using Machine Learning.” International Journal for Research in Applied Science and Engineering Technology 11 (4): 3760–64. https://doi.org/10.22214/ijraset.2023.50890.
[7]. Jamdar, Adit, Jessica Abraham, Karishma Khanna, and Rahul Dubey. 2015. “Emotion Analysis of Songs Based on Lyrical and Audio Features.” International Journal of Artificial Intelligence & Applications 6 (3): 35–50. https://doi.org/10.5121/ijaia.2015.6304.
[8]. Panda, Renato, Bruno Rocha, and Rui Pedro Paiva. 2015. “Music Emotion Recognition with Standard and Melodic Audio Features.” Applied Artificial Intelligence 29 (4): 313–34. https://doi.org/10.1080/08839514.2015.1016389.
[9]. Na, Wang, and Fang Yong. 2022. “Music Recognition and Classification Algorithm Considering Audio Emotion.” Scientific Programming 2022 (January): e3138851. https://doi.org/10.1155/2022/3138851.
[10]. Modran, Horia Alexandru, Tinashe Chamunorwa, Doru Ursuțiu, Cornel Samoilă, and Horia Hedeșiu. 2023. “Using Deep Learning to Recognize Therapeutic Effects of Music Based on Emotions.” Sensors 23 (2): 986. https://doi.org/10.3390/s23020986.
[11]. Shen, Qiangwei. 2023. “The Influence of Music Teaching Appreciation on the Mental Health of College Students Based on Multimedia Data Analysis.” PeerJ Computer Science 9 (September): e1589–89. https://doi.org/10.7717/peerj-cs.1589.
[12]. Agrawal, Yudhik, Ramaguru Guru Ravi Shanker, and Vinoo Alluri. 2021. “Transformer-Based Approach towards Music Emotion Recognition from Lyrics.” Lecture Notes in Computer Science, 167–75. https://doi.org/10.1007/978-3-030-72240-1_12.
[13]. Devlin, Jacob, Ming-Wei Chang, Kenton Lee, and Kristina Toutanova. 2019. “BERT: Pre-Training of Deep Bidirectional Transformers for Language Understanding.” ArXiv.org. May 24, 2019. https://doi.org/10.48550/arXiv.1810.04805.
[14]. Martinez, Servando Pizarro, Moritz Zimmermann, Miguel Serkan Offermann, and Florian Reither. 2024. “Exploring Genre and Success Classification through Song Lyrics Using DistilBERT: A Fun NLP Venture.” ArXiv (Cornell University), July. https://doi.org/10.48550/arxiv.2407.21068.
[15]. Revathy, V R, Anitha S Pillai, and Fatemah Daneshfar. 2023. “LyEmoBERT: Classification of Lyrics’ Emotion and Recommendation Using a Pre-Trained Model.” Procedia Computer Science 218 (January): 1196–1208. https://doi.org/10.1016/j.procs.2023.01.098.
[16]. Imdiptanu. 2019. “GitHub - Imdiptanu/Lyrics-Emotion-Detection: Single-Label and Multi-Label Classifiers to Detect Emotions in Lyrics Achieved 0.65 and 0.82 F1 Scores Respectively.” GitHub. 2019. https://github.com/imdiptanu/lyrics-emotion-detection/tree/master.
Cite this article
Hong,Y.;Xue,Y. (2025). Emotion Classification through Song Lyrics in Multi-Languages with Bert. Applied and Computational Engineering,108,59-68.
Data availability
The datasets used and/or analyzed during the current study will be available from the authors upon reasonable request.
Disclaimer/Publisher's Note
The statements, opinions and data contained in all publications are solely those of the individual author(s) and contributor(s) and not of EWA Publishing and/or the editor(s). EWA Publishing and/or the editor(s) disclaim responsibility for any injury to people or property resulting from any ideas, methods, instructions or products referred to in the content.
About volume
Volume title: Proceedings of the 5th International Conference on Signal Processing and Machine Learning
© 2024 by the author(s). Licensee EWA Publishing, Oxford, UK. This article is an open access article distributed under the terms and
conditions of the Creative Commons Attribution (CC BY) license. Authors who
publish this series agree to the following terms:
1. Authors retain copyright and grant the series right of first publication with the work simultaneously licensed under a Creative Commons
Attribution License that allows others to share the work with an acknowledgment of the work's authorship and initial publication in this
series.
2. Authors are able to enter into separate, additional contractual arrangements for the non-exclusive distribution of the series's published
version of the work (e.g., post it to an institutional repository or publish it in a book), with an acknowledgment of its initial
publication in this series.
3. Authors are permitted and encouraged to post their work online (e.g., in institutional repositories or on their website) prior to and
during the submission process, as it can lead to productive exchanges, as well as earlier and greater citation of published work (See
Open access policy for details).