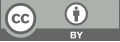
Evolution of YOLO: A Comparative Analysis of YOLOv5, YOLOv8, and YOLOv10
- 1 Computer Science and Technology, Nanjing University, Nanjing City, Jiangsu Province, 210023, China
* Author to whom correspondence should be addressed.
Abstract
This paper presents a systematic comparative analysis of three versions of the YOLO (You Only Look Once) target detection algorithm - YOLOv5, YOLOv8 and YOLOv10. Through experiments on the VOC2012 dataset (converted to COCO format), this paper evaluates the versions in terms of multiple dimensions such as detection performance, inference speed and model complexity. The experimental results show that the detection accuracy and robustness significantly improve with version iteration and the mAP of v8 v10 is improved by 6.69% and 9.12% relative to v5, However, the number of model parameters increases by 68.98% and 48.66, and the FLOPS increases by 94.08% and 91.51%, respectively, which leads to an increased demand for computational resources and a slight decrease in inference speed compared to the old version, especially in practical application scenarios with limited resources.This paper not only demonstrates the continuous progress of the network structure and training strategy, but also explores the balance between performance and efficiency in real-time target detection, which provides references and insights for the future development of related technologies.
Keywords
YOLO, Target detection, Comparative analysis, Performance evaluation, Model complexity
[1]. Girshick, R.B., Donahue, J., Darrell, T., & Malik, J. (2013). Rich Feature Hierarchies for Accurate Object Detection and Semantic Segmentation. 2014 IEEE Conference on Computer Vision and Pattern Recognition, 580-587.
[2]. Redmon, J., Divvala, S.K., Girshick, R.B., & Farhadi, A. (2015). You Only Look Once: Unified, Real-Time Object Detection. 2016 IEEE Conference on Computer Vision and Pattern Recognition (CVPR), 779-788.
[3]. Ren, Shaoqing et al. “Faster R-CNN: Towards Real-Time Object Detection with Region Proposal Networks.” IEEE transactions on pattern analysis and machine intelligence vol. 39,6 (2017): 1137-1149.
[4]. Liu, W. et al. (2016). SSD: Single Shot MultiBox Detector. In: Leibe, B., Matas, J., Sebe, N., Welling, M. (eds) Computer Vision – ECCV 2016. ECCV 2016. Lecture Notes in Computer Science, 14, 21-37
[5]. Vaswani, Ashish et al. “Attention is All you Need.” Neural Information Processing Systems (2017).
[6]. Carion, N., Massa, F., Synnaeve, G., Usunier, N., Kirillov, A., & Zagoruyko, S. (2020). End-to-End Object Detection with Transformers. Springer. p 213–229.
[7]. Glenn Jocher. YOLOv5 by Ultralytics, May 2020.
[8]. Glenn Jocher, Ayush Chaurasia, and Jing Qiu. YOLO by Ultralytics, January 2023.
[9]. Wang, Ao et al. “YOLOv10: Real-Time End-to-End Object Detection.” ArXiv abs/2405.14458 (2024): n. pag
[10]. Mark. Everingham, Luc. Van Gool, Chris Williams, JJohn. Winn, and AAndrew. Zisserman. The PASCAL Visual Object Classes Challenge 2012 (VOC2012) Results.
Cite this article
Zhang,J. (2025). Evolution of YOLO: A Comparative Analysis of YOLOv5, YOLOv8, and YOLOv10. Applied and Computational Engineering,119,173-181.
Data availability
The datasets used and/or analyzed during the current study will be available from the authors upon reasonable request.
Disclaimer/Publisher's Note
The statements, opinions and data contained in all publications are solely those of the individual author(s) and contributor(s) and not of EWA Publishing and/or the editor(s). EWA Publishing and/or the editor(s) disclaim responsibility for any injury to people or property resulting from any ideas, methods, instructions or products referred to in the content.
About volume
Volume title: Proceedings of the 3rd International Conference on Software Engineering and Machine Learning
© 2024 by the author(s). Licensee EWA Publishing, Oxford, UK. This article is an open access article distributed under the terms and
conditions of the Creative Commons Attribution (CC BY) license. Authors who
publish this series agree to the following terms:
1. Authors retain copyright and grant the series right of first publication with the work simultaneously licensed under a Creative Commons
Attribution License that allows others to share the work with an acknowledgment of the work's authorship and initial publication in this
series.
2. Authors are able to enter into separate, additional contractual arrangements for the non-exclusive distribution of the series's published
version of the work (e.g., post it to an institutional repository or publish it in a book), with an acknowledgment of its initial
publication in this series.
3. Authors are permitted and encouraged to post their work online (e.g., in institutional repositories or on their website) prior to and
during the submission process, as it can lead to productive exchanges, as well as earlier and greater citation of published work (See
Open access policy for details).