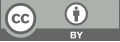
Anomaly Detection in Tax Filing Documents Using Natural Language Processing Techniques
- 1 Applied Statistics, Cornell University, NY, US
- 2 Information Science, University of Michigan, MI, USA
- 3 University of Rochester, Business Analytics, NY, USA
- 4 Business Analytics, UW Madison, WI, USA
* Author to whom correspondence should be addressed.
Abstract
This paper introduces a novel approach to tax fraud detection utilizing natural language processing techniques for identifying anomalies in tax filing documents. The methodology integrates tax-domain specific BERT embeddings with bidirectional LSTM networks to capture contextual relationships within tax documents that traditional numerical analysis might overlook. We present a multi-component ensemble framework that processes both structured and unstructured components of tax filings, extracting semantic relationships between financial entities while maintaining sensitivity to numerical inconsistencies. Using a dataset of 15,000 tax documents with 8.5% labeled anomalies, our approach demonstrates superior performance compared to existing methods, achieving an F1-score of 0.868 and AUC of 0.931—a 7.6% improvement over state-of-the-art techniques. The framework exhibits varying effectiveness across document types, with higher detection accuracy for individual income tax returns (F1-score 0.889) compared to business tax declarations (F1-score 0.818). Performance analysis reveals that semantic relationship features contribute significantly to anomaly detection in business tax documents, while numerical consistency features dominate in individual returns. Despite computational requirements exceeding traditional methods, the enhanced detection capabilities address critical gaps in existing tax fraud detection systems, particularly for sophisticated evasion strategies that manipulate textual elements while maintaining numerical plausibility.
Keywords
Tax Fraud Detection, Natural Language Processing, Anomaly Detection, Feature Extraction
[1]. Sankar, S., Subash, V., Princy, P., Vishalkumar, G., Booma, S., & Solayappan, A. (2024, April). A Novel Method for Detecting Financial Fraud Using Deep Learning in Online Retail. In 2024 Ninth International Conference on Science Technology Engineering and Mathematics (ICONSTEM) (pp. 1-5). IEEE.
[2]. Gresoi, S., Făgărăşan, I., Mocanu, Ş., & Stamatescu, G. (2023, May). Analysis of anomalies in fraud detection for Smart and Non-smart Grids. In 2023 24th International Conference on Control Systems and Computer Science (CSCS) (pp. 490-495). IEEE.
[3]. Mehta, P., Kumar, S., Kumar, R., & Babu, C. S. (2022, December). Enhancement to training of bidirectional gan: An approach to demystify tax fraud. In 2022 IEEE International Conference on Big Data (Big Data) (pp. 3524-3531). IEEE.
[4]. Mehta, P., Mathews, J., Rao, S. K. V., Kumar, K. S., Suryamukhi, K., & Babu, C. S. (2019, March). Identifying malicious dealers in goods and services tax. In 2019 IEEE 4th International Conference on Big Data Analytics (ICBDA) (pp. 312-316). IEEE.
[5]. Kumar, N. N., Sridhar, R., Prasanna, U. U., & Priyanka, G. (2023, April). Tax Management in the Digital Age: A TAB Algorithm-based Approach to Accurate Tax Prediction and Planning. In 2023 International Conference on Inventive Computation Technologies (ICICT) (pp. 908-915). IEEE.
[6]. Sun, J., Zhou, S., Zhan, X., & Wu, J. (2024). Enhancing Supply Chain Efficiency with Time Series Analysis and Deep Learning Techniques.
[7]. Wei, M., Wang, S., Pu, Y., & Wu, J. (2024). Multi-Agent Reinforcement Learning for High-Frequency Trading Strategy Optimization. Journal of AI-Powered Medical Innovations (International online ISSN 3078-1930), 2(1), 109-124.
[8]. Ma, D., Jin, M., Zhou, Z., Wu, J., & Liu, Y. (2024). Deep Learning-Based ADL Assessment and Personalized Care Planning Optimization in Adult Day Health Center. Applied and Computational Engineering, 118, 14-22.
[9]. Diao, S., Wan, Y., Huang, D., Huang, S., Sadiq, T., Khan, M. S., ... & Mazhar, T. (2025). Optimizing Bi-LSTM networks for improved lung cancer detection accuracy. PloS one, 20(2), e0316136.
[10]. Ma, X., Bi, W., Li, M., Liang, P., & Wu, J. (2025). An Enhanced LSTM-based Sales Forecasting Model for Functional Beverages in Cross-Cultural Markets. Applied and Computational Engineering, 118, 55-63.
[11]. Bi, W., Trinh, T. K., & Fan, S. (2024). Machine Learning-Based Pattern Recognition for Anti-Money Laundering in Banking Systems. Journal of Advanced Computing Systems, 4(11), 30-41.
[12]. Ma, X., & Fan, S. (2024). Research on Cross-national Customer Churn Prediction Model for Biopharmaceutical Products Based on LSTM-Attention Mechanism. Academia Nexus Journal, 3(3).
[13]. Wang, P., Varvello, M., Ni, C., Yu, R., & Kuzmanovic, A. (2021, May). Web-lego: trading content strictness for faster webpages. In IEEE INFOCOM 2021-IEEE Conference on Computer Communications (pp. 1-10). IEEE.
[14]. Ni, C., Zhang, C., Lu, W., Wang, H., & Wu, J. (2024). Enabling Intelligent Decision Making and Optimization in Enterprises through Data Pipelines.
[15]. Zheng, S., Zhang, Y., & Chen, Y. (2024). Leveraging Financial Sentiment Analysis for Detecting Abnormal Stock Market Volatility: An Evidence-Based Approach from Social Media Data. Academia Nexus Journal, 3(3).
[16]. Ma, D., & Ling, Z. (2024). Optimization of Nursing Staff Allocation in Elderly Care Institutions: A Time Series Data Analysis Approach. Annals of Applied Sciences, 5(1).
[17]. W. Xu, J. Xiao, and J. Chen, “Leveraging large language models to enhance personalized recommendations in e-commerce,” arXiv, arXiv:2410.12829, 2024.
[18]. Xiao, J., Deng, T., & Bi, S. (2024). Comparative Analysis of LSTM, GRU, and Transformer Models for Stock Price Prediction. arXiv preprint arXiv:2411.05790.
Cite this article
Liang,J.;Fan,J.;Feng,Z.;Xin,J. (2025). Anomaly Detection in Tax Filing Documents Using Natural Language Processing Techniques. Applied and Computational Engineering,144,80-89.
Data availability
The datasets used and/or analyzed during the current study will be available from the authors upon reasonable request.
Disclaimer/Publisher's Note
The statements, opinions and data contained in all publications are solely those of the individual author(s) and contributor(s) and not of EWA Publishing and/or the editor(s). EWA Publishing and/or the editor(s) disclaim responsibility for any injury to people or property resulting from any ideas, methods, instructions or products referred to in the content.
About volume
Volume title: Proceedings of the 3rd International Conference on Functional Materials and Civil Engineering
© 2024 by the author(s). Licensee EWA Publishing, Oxford, UK. This article is an open access article distributed under the terms and
conditions of the Creative Commons Attribution (CC BY) license. Authors who
publish this series agree to the following terms:
1. Authors retain copyright and grant the series right of first publication with the work simultaneously licensed under a Creative Commons
Attribution License that allows others to share the work with an acknowledgment of the work's authorship and initial publication in this
series.
2. Authors are able to enter into separate, additional contractual arrangements for the non-exclusive distribution of the series's published
version of the work (e.g., post it to an institutional repository or publish it in a book), with an acknowledgment of its initial
publication in this series.
3. Authors are permitted and encouraged to post their work online (e.g., in institutional repositories or on their website) prior to and
during the submission process, as it can lead to productive exchanges, as well as earlier and greater citation of published work (See
Open access policy for details).