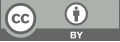
Detecting sarcastic expressions with deep neural networks
- 1 Shenzhen College of International Education, Shenzhen, China
* Author to whom correspondence should be addressed.
Abstract
Following the ever increasing trend in social media such as Twitter, Facebook, and Instagram, automatic analysis of people’s conversations and languages have become a problem of great significance for businesses and governments in attempt to understand and analyze people’s habits, thoughts, and patterns towards different subjects of interests. Within the field of natural language processing, sarcasm detection has always been a difficult challenge for sentiment analysis. Recent years, there has been great interests shown by researchers towards sarcasm detection. Neural networks achieve huge success and advancements surrounding this topic, but reviews for this task are very limited and there’s a lack of comprehensive review of the development of sarcasm detection so far. Thus, this paper aims to summarize and present the various methods directed towards sarcasm detection, the progress it has made, and examination of potential problems and availability for further improvements.
Keywords
sarcasm detection, deep learning
[1]. Sarsam, S. M., Al-Samarraie, H., Alzahrani, A. I., & Wright, B. (2020). Sarcasm detection using machine learning algorithms in Twitter: A systematic review. International Journal of Market Research, 62(5), 578-598.
[2]. Moores, B., & Mago, V. (2022). A Survey on Automated Sarcasm Detection on Twitter. arXiv preprint arXiv:2202.02516.
[3]. Joshi, A., Tripathi, V., Patel, K., Bhattacharyya, P., & Carman, M. (2016). Are word embedding-based features useful for sarcasm detection?. arXiv preprint arXiv:1610.00883.
[4]. Mishra, A., Kanojia, D., Nagar, S., Dey, K., & Bhattacharyya, P. (2017). Harnessing cognitive features for sarcasm detection. arXiv preprint arXiv:1701.05574.
[5]. Zhang, M., Zhang, Y., & Fu, G. (2016, December). Tweet sarcasm detection using deep neural network. In Proceedings of COLING 2016, the 26th International Conference on Computational Linguistics: technical papers (pp. 2449-2460).
[6]. Babanejad, N., Davoudi, H., An, A., & Papagelis, M. (2020, December). Affective and contextual embedding for sarcasm detection. In Proceedings of the 28th international conference on computational linguistics (pp. 225-243).
[7]. Jena, A. K., Sinha, A., & Agarwal, R. (2020, July). C-net: Contextual network for sarcasm detection. In Proceedings of the second workshop on figurative language processing (pp. 61-66).
[8]. Potamias, R. A., Siolas, G., & Stafylopatis, A. G. (2020). A transformer-based approach to irony and sarcasm detection. Neural Computing and Applications, 32(23), 17309-17320.
[9]. Lemmens, J., Burtenshaw, B., Lotfi, E., Markov, I., & Daelemans, W. (2020, July). Sarcasm detection using an ensemble approach. In proceedings of the second workshop on figurative language processing (pp. 264-269).
[10]. Hazarika, D., Poria, S., Gorantla, S., Cambria, E., Zimmermann, R., & Mihalcea, R. (2018). Cascade: Contextual sarcasm detection in online discussion forums. arXiv preprint arXiv:1805.06413.
[11]. M. H. Jafari, S. Samavi, S. M. R. Soroushmehr, H. Mohaghegh, N. Karimi, and K. Najarian, Set of descriptors for skin cancer diagnosis using non-dermoscopic color images,Sept 2016.
Cite this article
Huang,Z. (2023). Detecting sarcastic expressions with deep neural networks. Applied and Computational Engineering,5,62-68.
Data availability
The datasets used and/or analyzed during the current study will be available from the authors upon reasonable request.
Disclaimer/Publisher's Note
The statements, opinions and data contained in all publications are solely those of the individual author(s) and contributor(s) and not of EWA Publishing and/or the editor(s). EWA Publishing and/or the editor(s) disclaim responsibility for any injury to people or property resulting from any ideas, methods, instructions or products referred to in the content.
About volume
Volume title: Proceedings of the 3rd International Conference on Signal Processing and Machine Learning
© 2024 by the author(s). Licensee EWA Publishing, Oxford, UK. This article is an open access article distributed under the terms and
conditions of the Creative Commons Attribution (CC BY) license. Authors who
publish this series agree to the following terms:
1. Authors retain copyright and grant the series right of first publication with the work simultaneously licensed under a Creative Commons
Attribution License that allows others to share the work with an acknowledgment of the work's authorship and initial publication in this
series.
2. Authors are able to enter into separate, additional contractual arrangements for the non-exclusive distribution of the series's published
version of the work (e.g., post it to an institutional repository or publish it in a book), with an acknowledgment of its initial
publication in this series.
3. Authors are permitted and encouraged to post their work online (e.g., in institutional repositories or on their website) prior to and
during the submission process, as it can lead to productive exchanges, as well as earlier and greater citation of published work (See
Open access policy for details).