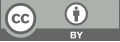
Feature analysis and model comparison of logistic regression and decision tree for customer churn prediction
- 1 Southwestern University of Finance and Economics
* Author to whom correspondence should be addressed.
Abstract
Customer churn has long been a concern for companies because it not only reduces the company's profit in the short term, but is also extremely detrimental to the company's growth in the long term. This paper focuses on the analysis of customer churn in banks by using two machine learning methods, namely logistic regression and decision tree, to predict the churn rate of customers and analyze the decision tree results based on the premise that decision trees are more accurate in prediction and do not have a large prediction bias for a certain group as logistic regression does. The results show that age, estimated salary and the number of products are important factors when predicting and customer groups with some specific characteristics will show a higher departure rate. To address this situation, this paper recommends that bankers continuously optimize their business systems and focus on user groups with high churn rates.
Keywords
machine learning, decision tree, logistic regression, customer churn prediction
[1]. Cuiyan, Z, Manman, Z, Xiaoling, X, et al.: Customer Churn Prediction Model Based on User Behavior Sequences. Journal of Donghua University (English Edition), 39(06), 597-602 (2022).
[2]. Jie, Z, Jianfeng, Y, Lu, Y, Peng, X, and Meng, W.: Application of LSTM Ensemble Method in Customer Churn Prediction. Computer applications and software, 36(11), 45 (2019).
[3]. Wanting, Z, Zhijie, Z, Yang, L, et, al.: Research on DBN Prediction Model of E-Commerce Customer Churn, Computer Engineering and Application, 58(11):84-92, (2022).
[4]. Li, Y.: Predictions model of customer churn in E-commerce based on online sequential optimization extreme learning machine, Journal of Nanjing University of Science And Technology, 43(01), 108-114 (2019).
[5]. Yanjun, C.: E⁃commerce customer churn estimation based on neural network, Modern Electronic Technology, 43(13), 103-105 (2020).
[6]. Ning, X, Fei, G.: Prediction Simulation of Customer Churn in E-Business of Wireless Network, Computer Simulation, 35(09), 475-479 (2018).
[7]. Xin, F, Chen, W, Yuan, L, et, al.: The Customer Churn Prediction Based on Emotional Polarity and BPNN, Journal of China Academy of Electronic Science, 13(03), 340-345 (2018).
[8]. Guoen, X, Wenbin, M, Chanjuan, T, et, al.: Study on the Value Feature and the Emotion Feature to Predict the Web Customer Churn, Chinese Journal of Management, 15(03), 442-449 (2018).
[9]. Zhen, Z.: Analysis of customer churn in the merger of accounting firms, Caikuai Tongxun, 861(01), 120-124 (2021).
[10]. Shu, L, Zhen, J.: A Study on the Economic Consequences of Big Customer Turnover in Accounting Firms, Kuaiji Yanjiu, 422(12), 174-184 (2022).
Cite this article
Wen,Z. (2023). Feature analysis and model comparison of logistic regression and decision tree for customer churn prediction. Applied and Computational Engineering,20,55-61.
Data availability
The datasets used and/or analyzed during the current study will be available from the authors upon reasonable request.
Disclaimer/Publisher's Note
The statements, opinions and data contained in all publications are solely those of the individual author(s) and contributor(s) and not of EWA Publishing and/or the editor(s). EWA Publishing and/or the editor(s) disclaim responsibility for any injury to people or property resulting from any ideas, methods, instructions or products referred to in the content.
About volume
Volume title: Proceedings of the 5th International Conference on Computing and Data Science
© 2024 by the author(s). Licensee EWA Publishing, Oxford, UK. This article is an open access article distributed under the terms and
conditions of the Creative Commons Attribution (CC BY) license. Authors who
publish this series agree to the following terms:
1. Authors retain copyright and grant the series right of first publication with the work simultaneously licensed under a Creative Commons
Attribution License that allows others to share the work with an acknowledgment of the work's authorship and initial publication in this
series.
2. Authors are able to enter into separate, additional contractual arrangements for the non-exclusive distribution of the series's published
version of the work (e.g., post it to an institutional repository or publish it in a book), with an acknowledgment of its initial
publication in this series.
3. Authors are permitted and encouraged to post their work online (e.g., in institutional repositories or on their website) prior to and
during the submission process, as it can lead to productive exchanges, as well as earlier and greater citation of published work (See
Open access policy for details).