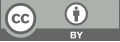
Optimization research on automatic pricing and replenishment decision method for vegetable commodities
- 1 Jilin University of Finance and Economics
* Author to whom correspondence should be addressed.
Abstract
This paper addresses the issues of replenishment and pricing of vegetable commodities in fresh food supermarkets. By analyzing sales data and loss rate data, mathematical models are established to solve the following problems. Spearman correlation analysis and cluster analysis methods are employed. Firstly, data preprocessing is conducted by excluding returned vegetable products from a certain supermarket’s distribution, and then integrating sales transaction details and wholesale price-related data. Secondly, monthly data is used to succinctly present the sales distribution patterns of various categories and individual items of vegetables through line charts. Finally, the models of Spearman correlation analysis and cluster analysis are respectively applied to illustrate the relationship between the sales volume of 6 major categories of vegetables and 251 individual items. Linear regression models, time series analysis, and XG-Boost regression analysis models are used. This paper requires replenishment plans to be made by category, analyzing the relationship between the total sales volume and cost-plus pricing of various vegetable categories. Firstly, a linear regression model is applied to fit historical sales volume and cost-plus pricing, resulting in a linear functional relationship, indicating that only the sales volume of tomatoes is negatively correlated with cost-plus pricing. Based on historical sales data, the daily replenishment total for each vegetable category within the next week is predicted. Predictive models and time series analysis are employed. Meanwhile, for pricing strategies, the XGBoost algorithm is utilized to provide reasonable pricing strategies. Based on the optimal solution, replenishment plans and pricing strategies for the next week are formulated to ensure the maximization of sales revenue objectives.
Keywords
cost-plus pricing, replenishment decision, Spearman correlation coefficient, cluster analysis, linear regression
[1]. Scientific Platform Serving for Statistics Professional. (2021). SPSSPRO (Version 1.0.11) [Online Application Software]. Retrieved from https://www.spsspro.com.
[2]. Xu, W. C. (2012). A Review of Correlation Coefficients Research. Journal of Guangdong University of Technology, 29(3), 12-17.
[3]. Scientific Platform Serving for Statistics Professional. (2021). SPSSPRO (Version 1.0.11) [Online Application Software]. Retrieved from https://www.spsspro.com.
[4]. Saroj, K., & Kavita. (2016). Review: Study on Simple K Mean and Modified K Mean Clustering Technique. International Journal of Computer Science Engineering and Technology, 6(7), 279-281.
[5]. Scientific Platform Serving for Statistics Professional. (2021). SPSSPRO (Version 1.0.11) [Online Application Software]. Retrieved from https://www.spsspro.com.
[6]. Wang, Y. (2005). Application of Time Series Analysis. Beijing: China Renmin University Press.
[7]. Scientific Platform Serving for Statistics Professional. (2021). SPSSPRO (Version 1.0.11) [Online Application Software]. Retrieved from https://www.spsspro.com.
[8]. Chen, T., & Guestrin, C. (2016). XGBoost: A Scalable Tree Boosting System. ACM.
[9]. Scientific Platform Serving for Statistics Professional. (2021). SPSSPRO (Version 1.0.11) [Online Application Software]. Retrieved from https://www.spsspro.com.
[10]. Draper, N. R., & Smith, H. (1998). Applied Regression Analysis. Wiley Series in Probability and Statistics.
Cite this article
Long,C. (2024). Optimization research on automatic pricing and replenishment decision method for vegetable commodities. Advances in Engineering Innovation,7,48-54.
Data availability
The datasets used and/or analyzed during the current study will be available from the authors upon reasonable request.
Disclaimer/Publisher's Note
The statements, opinions and data contained in all publications are solely those of the individual author(s) and contributor(s) and not of EWA Publishing and/or the editor(s). EWA Publishing and/or the editor(s) disclaim responsibility for any injury to people or property resulting from any ideas, methods, instructions or products referred to in the content.
About volume
Journal:Advances in Engineering Innovation
© 2024 by the author(s). Licensee EWA Publishing, Oxford, UK. This article is an open access article distributed under the terms and
conditions of the Creative Commons Attribution (CC BY) license. Authors who
publish this series agree to the following terms:
1. Authors retain copyright and grant the series right of first publication with the work simultaneously licensed under a Creative Commons
Attribution License that allows others to share the work with an acknowledgment of the work's authorship and initial publication in this
series.
2. Authors are able to enter into separate, additional contractual arrangements for the non-exclusive distribution of the series's published
version of the work (e.g., post it to an institutional repository or publish it in a book), with an acknowledgment of its initial
publication in this series.
3. Authors are permitted and encouraged to post their work online (e.g., in institutional repositories or on their website) prior to and
during the submission process, as it can lead to productive exchanges, as well as earlier and greater citation of published work (See
Open access policy for details).