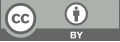
What role does object detection play in autonomous driving?
- 1 Xi'an Jiaotong University Affiliated Middle School
* Author to whom correspondence should be addressed.
Abstract
Deep learning-based object detection algorithm is becoming more and more important in autonomous driving area with an increasing amount and trending these days. This article first provides definitions and introduces an autonomous driving and object detection. Subsequently, a detailed discussion is conducted on object detection, comparing traditional object detection methods with deep learning object detection algorithms. The shortcomings of traditional methods highlighted the advantages of deep learning-based object detection algorithms, laying the groundwork for the use of deep learning object detection algorithms in the following text. Finally, several detection objects and detection scenarios are introduced. The detection objects are divided into different parts, including moving targets, stationary targets, and infrared targets. Moving targets such as pedestrians and vehicles, while stationary targets include traffic signs and lanes. The detection scenarios are classified into ordinary scene detection and complex scene detection. In the discussion section, the commonly used datasets for autonomous driving target detection training are listed first, such as the KITTI dataset, the COCO dataset, and so on. Subsequently, a discussion was conducted on algorithms, mainly focusing on the models and features in one stage and two stages. For different types of algorithms, this article discusses the advantages and disadvantages of the algorithms. When judging the superiority or inferiority of algorithms, there are usually two aspects: detection accuracy and detection speed. FPS is a commonly used indicator for detection speed, and detection accuracy mainly covers five aspects, namely accuracy, precision, recall, AP (average precision), and MAP (mean average precision). Finally, how the improved algorithms are applied and solve the existing problems is discussed.
Keywords
object detection, autonomous driving, deep learning
[1]. Mao, Z., Zhu, J., Wu, X., & Li, J. (2022). Review of YOLO based target detection for autonomous driving. Computer Engineering and Applications, 58(15), 68-77. https://doi.org/10.3778/j.issn.1002-8331.2203-0310
[2]. Li, A., Gong, H., Huang, X., & Cao, J. (2022). Overview of target detection methods for autonomous vehicles. Journal of Shandong Jiaotong University, 30(3), 20-29.
[3]. Song, S., Xia, H., & Li, G. (2023). Research on improved YOLOv5 algorithm and its application in multi-object detection for automatic driving. Computer Engineering and Applications, 59(15), 68-75.
[4]. Li, Y., Hou, L., & Wang, C. (2019). Moving objects detection in automatic driving based on YOLOv3. Computer Engineering and Design, 40(4), 1139-1144.
[5]. Shi, Y., & Jis, S. (2022). CB-CenterNet based autonomous driving occlusion target. Journal of Dalian Jiaotong University, 43(3), 115-120.
[6]. Xu, X., Ma, Y., Qian, X., & Zhang, Y. (2021). Scale-aware Efficient Det: Real-time pedestrian detection algorithm for automated driving. Journal of Image and Graphics, 26(1), 93-100.
[7]. Shi, J., Li, X., Liu, W., & An, X. (2022). Target detection and recognition based on YOLOv4-Efficient. Intelligent Computer and Applications, 12(3), 123-127.
[8]. Chen, Y., Yan, Z., Zhou, C., & Huang, Y. (2023). Vehicle detection in autonomous driving scenarios based on improved YOLOv4. Detecting Technology and Data Processing, 38(1), 59-63, 85.
[9]. Hu, J., Wang, H., & Dai, X. (2023). Real-time detection algorithm for small-target traffic signs based on improved YOLOv5. Computer Engineering and Applications, 59(2), 185-193.
[10]. Wang, C., & Fu, Z. (2018). Traffic sign detection based on YOLOv2 model. Coden Jyiidu, 38(z2), 276-278.
[11]. Zhang, K., & Zhu, M. (2022). Environmental perception algorithm for multi-task autonomous driving based on YOLOv5. Computer Systems Applications, 31(9), 226–232. https://doi.org/10.15888/j.cnki.csa.008698
[12]. Lin, J., Zhang, W., Zhang, K., & Zhang, Y. (2022). Infrared target detection based on YOLOv5. Flight Control & Detection, 5(3), 63-71.
[13]. Wen, H., & Tong, M. (2023). Object detection in automatic driving scenarios based on semi-supervised learning. Microelectronic & Computer, 40(2), 22-36.
[14]. Gu, D., Luo, Y., & Li, W. (2022). Traffic target detection in complex scenes based on improved YOLOv5 algorithm. Journal of Northeastern University (Natural Science), 43(8), 1073-1079.
[15]. Wildcraner. (2021). Detailed explanation KITTI dataset. [Blog post]. https://blog.csdn.net/weixin_46195203/article/details/115870752
[16]. Geiger, A., et al. (2013). Vision meets robotics: The KITTI dataset. International Journal of Robotics Research, 32(11), 1231-1237.
[17]. Doan, A., Okatan, A., & Etinkaya, A. (2021). The code: Vehicle classification and tracking using convolutional neural network based on Darknet YOLO with COCO dataset. [ResearchGate publication]. https://www.researchgate.net/publication/353958426_Vehicle_Classification_and_Tracking_Using_Convolutional_Neural_Network_Based_on_Darknet_Yolo_with_Coco_Data
[18]. Waiting for Godot. (2022). Introduce COCO dataset. [Blog post]. https://blog.csdn.net/qq_44554428/article/details/122597358
[19]. [19] Ertler, C., et al. (2022). The code: The Mapillary traffic sign dataset for detection and classification on a global scale. [arXiv preprint]. https://arxiv.org/abs/1909.04422
[20]. [20] Zhu, Z., et al. (2016). Traffic-sign detection and classification in the wild. [Conference paper]. https://www.cv-foundation.org/openaccess/content_cvpr_2016/papers/Zhu_Traffic-Sign_Detection_and_CVPR_2016_paper.pdf
[21]. [21] Murakami, M., et al. (2022). Radiative thermal conductivity of single-crystal bridgmanite at the core-mantle boundary with implications for thermal evolution of the Earth. Earth and Planetary Science Letters, 578, 117329. https://doi.org/10.1016/j.epsl.2021.117329
[22]. AI Bacteria. (2023). YOLO series of algorithms in detail: The road from YOLOv1 to YOLOv8. [Blog post]. https://blog.csdn.net/wjinjie/article/details/107509243
[23]. He, X., Luo, Y., & Jiang, L. (2021). Front vehicle detection based on YOLO. Ship Electronic Engineering, 41(1), 137-139.
[24]. Redmon, J., Divvala, S., Girshick, R., & Farhadi, A. (2015). You only look once: Unified, real-time object detection. arXiv preprint. https://arxiv.org/abs/1506.02640
[25]. Zhao, Z. (2022). Analysis of target detection algorithm and its application in autonomous driving scenarios. Automobile Applied Technology, 47(4), 29-33.
[26]. Plain white water. (2022). Object detection->SSD arithmetic. [Blog post]. https://blog.csdn.net/wanchengkai/article/details/12477589
[27]. Liu, W., et al. (2015). SSD: Single shot multibox detector. [arXiv preprint]. https://doi.org/10.48550/arXiv.1512.02325
[28]. Girshick, R., Donahue, J., Darrell, T., & Malik, J. (2013). Rich feature hierarchies for accurate object detection and semantic segmentation. arXiv preprint. https://doi.org/10.48550/arxiv.1311.2524
[29]. He, K., Zhang, X., Ren, S., & Sun, J. (2015). Spatial pyramid pooling in deep convolutional networks for visual recognition. IEEE Transactions on Pattern Analysis and Machine Intelligence, 37(9), 1904-1916.
[30]. Girshick, R. (2015). Fast R-CNN. In Proceedings of the 2015 IEEE International Conference on Computer Vision (ICCV) (pp. 1440–1448). https://doi.org/10.1109/iccv.2015.169
[31]. Ren, S., He, K., Girshick, R., & Sun, J. (2015). Faster R-CNN: Towards real-time object detection with region proposal networks. arXiv preprint. https://doi.org/10.48550/arxiv.1506.01497
[32]. Mao, Z., Zhu, J., Wu, X., & Li, J. (2022). Review of YOLO based target detection for autonomous driving. Computer Engineering and Applications, 58(15), 29-33.
Cite this article
Han,Y. (2024). What role does object detection play in autonomous driving?. Advances in Engineering Innovation,10,76-84.
Data availability
The datasets used and/or analyzed during the current study will be available from the authors upon reasonable request.
Disclaimer/Publisher's Note
The statements, opinions and data contained in all publications are solely those of the individual author(s) and contributor(s) and not of EWA Publishing and/or the editor(s). EWA Publishing and/or the editor(s) disclaim responsibility for any injury to people or property resulting from any ideas, methods, instructions or products referred to in the content.
About volume
Journal:Advances in Engineering Innovation
© 2024 by the author(s). Licensee EWA Publishing, Oxford, UK. This article is an open access article distributed under the terms and
conditions of the Creative Commons Attribution (CC BY) license. Authors who
publish this series agree to the following terms:
1. Authors retain copyright and grant the series right of first publication with the work simultaneously licensed under a Creative Commons
Attribution License that allows others to share the work with an acknowledgment of the work's authorship and initial publication in this
series.
2. Authors are able to enter into separate, additional contractual arrangements for the non-exclusive distribution of the series's published
version of the work (e.g., post it to an institutional repository or publish it in a book), with an acknowledgment of its initial
publication in this series.
3. Authors are permitted and encouraged to post their work online (e.g., in institutional repositories or on their website) prior to and
during the submission process, as it can lead to productive exchanges, as well as earlier and greater citation of published work (See
Open access policy for details).