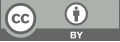
Transfer learning on animal species recognition tasks
- 1 Imperial College London
* Author to whom correspondence should be addressed.
Abstract
In the field of wildlife conservation, it is challenging to distinguish visually similar species, such as leopards, lions and tigers. This poses huge obstacles for automated detection systems. In this study, transfer learning uses YOLOv5 model from Ultralytics repository to enhance the accuracy of the detection. We pre-trained the model on datasets specific to each species and then applied transfer learning across different species pairs (e.g., leopard to lion, lion to tiger). Our results indicate that while models pre-trained on individual species achieved high detection accuracy (mAP@0.5 > 0.95), the effectiveness of transfer learning varied significantly depending on the visual similarity between species. For instance, transferring from leopard to lion demonstrated strong performance (peak mAP@0.5 of 0.91), while transferring from leopard to tiger resulted in lower accuracy (peak mAP@0.5 of 0.80), showing the limitations of transferring learned features between more visually distinct species. These findings indicate that transfer learning largely reduces training time and improves the adaptability of the models when applied to closely related species. However, further improvements are still needed for more distant species pairs and for too small datasets.
Keywords
deep learning, transfer learning, object detection, ecology
[1]. S. Ren, K. He, R. Girshick, and J. Sun, “Faster R-CNN: Towards Real-Time Object Detection with Region Proposal Networks,” IEEE Transactions on Pattern Analysis and Machine Intelligence, vol. 39, no. 6, pp. 1137–1149, Jun. 2017, doi: https://doi.org/10.1109/tpami.2016.2577031.
[2]. D. G. Lowe, “Distinctive Image Features from Scale-Invariant Keypoints,” International Journal of Computer Vision, vol. 60, no. 2, pp. 91–110, Nov. 2004, doi: https://doi.org/10.1023/b:visi.0000029664.99615.94.
[3]. S. J. Pan and Q. Yang, “A Survey on Transfer Learning,” IEEE Transactions on Knowledge and Data Engineering, vol. 22, no. 10, pp. 1345–1359, Oct. 2010, doi: https://doi.org/10.1109/tkde.2009.191.
[4]. A. Esteva et al., “Dermatologist-level Classification of Skin Cancer with Deep Neural Networks,” Nature, vol. 542, no. 7639, pp. 115–118, Jan. 2017, doi: https://doi.org/10.1038/nature21056.
[5]. J. Yosinski, J. Clune, Y. Bengio, and H. Lipson, “How transferable are features in deep neural networks?,” arXiv.org, 2014. https://arxiv.org/abs/1411.1792
[6]. N. M. Haddad et al., “Habitat fragmentation and its lasting impact on Earth’s ecosystems,” Science Advances, vol. 1, no. 2, p. e1500052, Mar. 2015, doi: https://doi.org/10.1126/sciadv.1500052.
[7]. G. Jocher, “ultralytics/yolov5,” GitHub, Aug. 21, 2020. https://github.com/ultralytics/yolov5
[8]. J. Redmon and A. Farhadi, “YOLOv3: An Incremental Improvement,” arXiv.org, 2018. https://arxiv.org/abs/1804.02767
[9]. A. Bochkovskiy, C.-Y. Wang, and H.-Y. M. Liao, “YOLOv4: Optimal Speed and Accuracy of Object Detection,” arXiv, Apr. 2020, Available: https://arxiv.org/abs/2004.10934
[10]. O. Russakovsky et al., “ImageNet Large Scale Visual Recognition Challenge,” International Journal of Computer Vision, vol. 115, no. 3, pp. 211–252, Apr. 2015, doi: https://doi.org/10.1007/s11263-015-0816-y.
[11]. T.-Y. Lin et al., “Microsoft COCO: Common Objects in Context,” Computer Vision – ECCV 2014, vol. 8693, pp. 740–755, 2014, doi: https://doi.org/10.1007/978-3-319-10602-1_48.
Cite this article
Zhu,H. (2024). Transfer learning on animal species recognition tasks. Advances in Engineering Innovation,11,60-63.
Data availability
The datasets used and/or analyzed during the current study will be available from the authors upon reasonable request.
Disclaimer/Publisher's Note
The statements, opinions and data contained in all publications are solely those of the individual author(s) and contributor(s) and not of EWA Publishing and/or the editor(s). EWA Publishing and/or the editor(s) disclaim responsibility for any injury to people or property resulting from any ideas, methods, instructions or products referred to in the content.
About volume
Journal:Advances in Engineering Innovation
© 2024 by the author(s). Licensee EWA Publishing, Oxford, UK. This article is an open access article distributed under the terms and
conditions of the Creative Commons Attribution (CC BY) license. Authors who
publish this series agree to the following terms:
1. Authors retain copyright and grant the series right of first publication with the work simultaneously licensed under a Creative Commons
Attribution License that allows others to share the work with an acknowledgment of the work's authorship and initial publication in this
series.
2. Authors are able to enter into separate, additional contractual arrangements for the non-exclusive distribution of the series's published
version of the work (e.g., post it to an institutional repository or publish it in a book), with an acknowledgment of its initial
publication in this series.
3. Authors are permitted and encouraged to post their work online (e.g., in institutional repositories or on their website) prior to and
during the submission process, as it can lead to productive exchanges, as well as earlier and greater citation of published work (See
Open access policy for details).