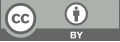
Tracking abandoned objects in surveillance video based on human trajectory
- 1 Xiamen University Tan Kah Kee College
* Author to whom correspondence should be addressed.
Abstract
Intelligent video surveillance is a significant research area in computer vision, with the detection and tracking of abandoned objects in public spaces attracting considerable public attention. However, existing detection technologies still face challenges, including false alarms, missed detections, poor adaptability to environmental conditions, and an inability to monitor in real-time. This paper proposes a method for tracking abandoned objects in surveillance video by analyzing human trajectories. The method addresses three main challenges: detection, tracking, and identification of abandoned objects. First, the paper discusses the inter-frame comparison method and the YOLOv8-based object detection algorithm for identifying abandoned objects and determining their categories. Additionally, it explores person re-identification technology and its application in identifying the owner of an abandoned object. Finally, the movement trajectory of the object’s owner is analyzed to determine the intent of the person who placed it.
Keywords
abandoned objects, object detection, trajectory tracking, person re-identification
[1]. Wei, W. , Yang, W. , Zuo, E. , Qian, Y. , & Wang, L. . (2022). Person re-identification based on deep learning - an overview. Journal of visual communication & image representation(Jan.), 82.
[2]. Ye, M. , Shen, J. , Lin, G. , Xiang, T. , & Hoi, S. C. H. . (2021). Deep learning for person re-identification: a survey and outlook. IEEE Transactions on Pattern Analysis and Machine Intelligence, PP(99), 1-1.
[3]. Ming, Z. , Zhu, M. , Wang, X. , Zhu, J. , Cheng, J. , & Gao, C. , et al. (2022). Deep learning-based person re-identification methods: a survey and outlook of recent works. Image and vision computing(Mar.), 119.
[4]. Zhou, K. , Yang, Y. , Cavallaro, A. , & Xiang, T. . (2019). Omni-scale feature learning for person re-identification.
[5]. Hou Rui, & Tang Zhenmin. (2023). A multi-step method for road remnants detection based on deep learning. Computer & Digital Engineering, 51(8), 1756-1760.
[6]. Anonymous. (2023). A Precise Identification Method for Small Targets of Legacy Based on Yolov5. CN117315233A.
[7]. Yu Fei, Xu Bin, Wang Ronghao, Han Hequan. (2023). Rotating chain plate detection algorithm based on improved yolov8. Manufacturing automation, 45(9), 212-216.
[8]. Su Jia, Jia Ze, Qin Yichang & Zhang Jianyan. (2024). Improved YOLOv8 algorithm for industrial surface defect detection. Computer Engineering and Applications (14), 187-196.
Cite this article
Yang,X. (2024). Tracking abandoned objects in surveillance video based on human trajectory. Advances in Engineering Innovation,11,64-72.
Data availability
The datasets used and/or analyzed during the current study will be available from the authors upon reasonable request.
Disclaimer/Publisher's Note
The statements, opinions and data contained in all publications are solely those of the individual author(s) and contributor(s) and not of EWA Publishing and/or the editor(s). EWA Publishing and/or the editor(s) disclaim responsibility for any injury to people or property resulting from any ideas, methods, instructions or products referred to in the content.
About volume
Journal:Advances in Engineering Innovation
© 2024 by the author(s). Licensee EWA Publishing, Oxford, UK. This article is an open access article distributed under the terms and
conditions of the Creative Commons Attribution (CC BY) license. Authors who
publish this series agree to the following terms:
1. Authors retain copyright and grant the series right of first publication with the work simultaneously licensed under a Creative Commons
Attribution License that allows others to share the work with an acknowledgment of the work's authorship and initial publication in this
series.
2. Authors are able to enter into separate, additional contractual arrangements for the non-exclusive distribution of the series's published
version of the work (e.g., post it to an institutional repository or publish it in a book), with an acknowledgment of its initial
publication in this series.
3. Authors are permitted and encouraged to post their work online (e.g., in institutional repositories or on their website) prior to and
during the submission process, as it can lead to productive exchanges, as well as earlier and greater citation of published work (See
Open access policy for details).