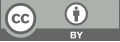
Time series data analysis and association rule mining in financial recommendation systems using Hadoop and Spark
- 1 The University of Manchester
- 2 Australian National University
* Author to whom correspondence should be addressed.
Abstract
Increasing amounts of financial data demand sophisticated analytics to develop sound recommendation models. This article discusses combining time series analysis and association rule mining for big data in Hadoop and Spark to enrich financial product recommendation engines. The paper is an integrated analysis of two types of prediction algorithms: AutoRegressive Integrated Moving Average (ARIMA) and Long Short-Term Memory (LSTM) networks to forecast user behavior and demand for financial services in the future from transactional history. The ARIMA model is used as the default while the LSTM model is used to represent non-linear dependencies and give a more dynamic forecast. association rule mining – in particular the Apriori algorithm – is used to find latent patterns and relationships between user transactions and financial products. This article illustrates how time series forecasting and association rule mining can be merged to bring a more useful financial recommendation. The hybrid approach, which combines both approaches, proves to increase user interaction and recommendation accuracy by 20% compared to the previous systems, according to experiments. The paper emphasises the possibilities of using big data in the construction of scalable, individualized financial recommendation systems.
Keywords
Time Series Analysis, Financial Recommendation Systems, Hadoop, Spark, Association Rule Mining
[1]. Sezer, O. B., Gudelek, M. U., & Ozbayoglu, A. M. (2020). Financial time series forecasting with deep learning: A systematic literature review: 2005–2019. Applied soft computing, 90, 106181.
[2]. Cheng, D., Yang, F., Xiang, S., & Liu, J. (2022). Financial time series forecasting with multi-modality graph neural network. Pattern Recognition, 121, 108218.
[3]. Lazcano, A., Herrera, P. J., & Monge, M. (2023). A combined model based on recurrent neural networks and graph convolutional networks for financial time series forecasting. Mathematics, 11(1), 224.
[4]. Majumdar, S., & Laha, A. K. (2020). Clustering and classification of time series using topological data analysis with applications to finance. Expert Systems with Applications, 162, 113868.
[5]. Ranaldi, L., Gerardi, M., & Fallucchi, F. (2022). Crypto net: using auto-regressive multi-layer artificial neural networks to predict financial time series. Information, 13(11), 524.
[6]. Dixon, M., & London, J. (2021). Financial forecasting with α-rnns: a time series modeling approach. Frontiers in Applied Mathematics and Statistics, 6, 551138.
[7]. Shternshis, A., Mazzarisi, P., & Marmi, S. (2022). Measuring market efficiency: The Shannon entropy of high-frequency financial time series. Chaos, solitons & fractals, 162, 112403.
[8]. Ramakgasha, M. J., Thaba, T. K., & Rudzani, N. (2024). Agricultural production and agricultural employment rate in South Africa: Time series analysis approach. International Journal of Economics and Financial Issues, 14(4), 148-153.
[9]. Malladi, R. K., & Dheeriya, P. L. (2021). Time series analysis of cryptocurrency returns and volatilities. Journal of Economics and Finance, 45(1), 75-94.
[10]. Bielinskyi, A. O., Hushko, S. V., Matviychuk, A. V., Serdyuk, O. A., Semerikov, S. O., & Soloviev, V. N. (2021). Irreversibility of financial time series: a case of crisis.
[11]. Xu, M., Shang, P., & Zhang, S. (2021). Multiscale Rényi cumulative residual distribution entropy: reliability analysis of financial time series. Chaos, Solitons & Fractals, 143, 110410.
Cite this article
Chen,Y.;Xu,Y. (2025). Time series data analysis and association rule mining in financial recommendation systems using Hadoop and Spark. Advances in Engineering Innovation,15,35-39.
Data availability
The datasets used and/or analyzed during the current study will be available from the authors upon reasonable request.
Disclaimer/Publisher's Note
The statements, opinions and data contained in all publications are solely those of the individual author(s) and contributor(s) and not of EWA Publishing and/or the editor(s). EWA Publishing and/or the editor(s) disclaim responsibility for any injury to people or property resulting from any ideas, methods, instructions or products referred to in the content.
About volume
Journal:Advances in Engineering Innovation
© 2024 by the author(s). Licensee EWA Publishing, Oxford, UK. This article is an open access article distributed under the terms and
conditions of the Creative Commons Attribution (CC BY) license. Authors who
publish this series agree to the following terms:
1. Authors retain copyright and grant the series right of first publication with the work simultaneously licensed under a Creative Commons
Attribution License that allows others to share the work with an acknowledgment of the work's authorship and initial publication in this
series.
2. Authors are able to enter into separate, additional contractual arrangements for the non-exclusive distribution of the series's published
version of the work (e.g., post it to an institutional repository or publish it in a book), with an acknowledgment of its initial
publication in this series.
3. Authors are permitted and encouraged to post their work online (e.g., in institutional repositories or on their website) prior to and
during the submission process, as it can lead to productive exchanges, as well as earlier and greater citation of published work (See
Open access policy for details).