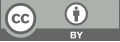
A distributed system log anomaly detection framework based on graph-chain architecture
- 1 National University of Defense Technology
- 2 National University of Defense Technology
- 3 National University of Defense Technology
* Author to whom correspondence should be addressed.
Abstract
Enterprise alliances and government agencies typically deploy distributed systems across subsidiaries and subordinate departments, where each system node collaborates via a network to present a unified interface to users. Addressing the challenges of log data dispersion, heterogeneity, and lack of credibility in distributed systems, this paper proposes a log anomaly detection framework based on a graph-chain architecture. The framework leverages the sequence analysis capabilities of a distilled Transformer model to detect anomalies in system logs at each node. Finally, by integrating blockchain smart contracts, it ensures tamper resistance and traceability. Experimental results demonstrate that the proposed framework achieves an anomaly detection accuracy of 99.6%, surpassing traditional methods.
Keywords
distributed systems, blockchain, anomaly detection, artificial intelligence
[1]. Xu, W., Huang, L., Fox, A., Patterson, D., & Jordan, M. I. (2009). Detecting large-scale system problems by mining console logs. In Proceedings of the ACM SIGOPS 22nd Symposium on Operating Systems Principles (pp. 117–132). Association for Computing Machinery. https://doi.org/10.1145/1629575.1629587
[2]. Li, K.L., Huang, H.K., Tian, S.F., & Xu, W. (2003). Improving one-class SVM for anomaly detection. Proceedings of the 2003 International Conference on Machine Learning and Cybernetics (Vol. 5, pp. 3077-3081). IEEE. https://doi.org/10.1109/ICMLC.2003.1260106
[3]. Wang, Y., Wong, J., & Miner, A. (2004). Anomaly intrusion detection using one class SVM. In Proceedings from the Fifth Annual IEEE SMC Information Assurance Workshop (pp. 358–364).
[4]. Sanh, V., Debut, L., Chaumond, J., & Wolf, T. (2019). DistilBERT, a distilled version of BERT: smaller, faster, cheaper and lighter. arXiv preprint arXiv:1910.01108.
[5]. Xiong, T., Xie, T., Xie, J., & Luo, X. (2021). Oric: A self-adjusting blockchain protocol with high throughput. In 2021 IEEE Intl Conf on Parallel & Distributed Processing with Applications, Big Data & Cloud Computing, Sustainable Computing & Communications, Social Computing & Networking (ISPA/BDCloud/SocialCom/SustainCom) (pp. 1422-1434).
[6]. Du, M., Li, F., Zheng, G., & Srikumar, V. (2017). DeepLog: Anomaly detection and diagnosis from system logs through deep learning. Proceedings of the 2017 ACM SIGSAC Conference on Computer and Communications Security (pp. 1285-1298). Association for Computing Machinery.
[7]. Zhang, X., Xu, Y., Lin, Q., Qiao, B., Zhang, H., Dang, Y., Xie, C., Yang, X., Cheng, Q., Li, Z., Chen, J., He, X., Yao, R., Lou, J.G., Chintalapati, M., Shen, F., & Zhang, D. (2019). Robust log-based anomaly detection on unstable log data. In Proceedings of the 2019 27th ACM joint meeting on European software engineering conference and symposium on the foundations of software engineering (pp. 807-817).
[8]. Le, V. H., & Zhang, H. (2021). Log-based anomaly detection without log parsing. In 2021 36th IEEE/ACM International Conference on Automated Software Engineering (ASE) (pp. 492-504).
Cite this article
Hu,M.;Song,C.;Xie,T. (2025). A distributed system log anomaly detection framework based on graph-chain architecture. Advances in Engineering Innovation,16(3),56-64.
Data availability
The datasets used and/or analyzed during the current study will be available from the authors upon reasonable request.
Disclaimer/Publisher's Note
The statements, opinions and data contained in all publications are solely those of the individual author(s) and contributor(s) and not of EWA Publishing and/or the editor(s). EWA Publishing and/or the editor(s) disclaim responsibility for any injury to people or property resulting from any ideas, methods, instructions or products referred to in the content.
About volume
Journal:Advances in Engineering Innovation
© 2024 by the author(s). Licensee EWA Publishing, Oxford, UK. This article is an open access article distributed under the terms and
conditions of the Creative Commons Attribution (CC BY) license. Authors who
publish this series agree to the following terms:
1. Authors retain copyright and grant the series right of first publication with the work simultaneously licensed under a Creative Commons
Attribution License that allows others to share the work with an acknowledgment of the work's authorship and initial publication in this
series.
2. Authors are able to enter into separate, additional contractual arrangements for the non-exclusive distribution of the series's published
version of the work (e.g., post it to an institutional repository or publish it in a book), with an acknowledgment of its initial
publication in this series.
3. Authors are permitted and encouraged to post their work online (e.g., in institutional repositories or on their website) prior to and
during the submission process, as it can lead to productive exchanges, as well as earlier and greater citation of published work (See
Open access policy for details).