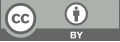
Facial Expression Recognition method based on residual network and attention mechanism
- 1 Nanjing Audit University
* Author to whom correspondence should be addressed.
Abstract
As a primary medium for emotional expression, human facial expressions carry rich informational value. Recent advancements in residual networks and attention mechanisms have broadened their application in expression classification, yet challenges persist in suboptimal key feature extraction and complex model training. To address these issues, this study proposes a novel facial expression recognition method integrating residual networks with attention mechanisms. The framework employs ResNet50 as the backbone network for feature extraction, enhanced by the Convolutional Block Attention Module (CBAM) to autonomously learn and prioritize critical features. Further innovations include reconstructing residual modules within the backbone network to optimize feature extraction and introducing a CAM-adjusted CBAM-ERF mechanism to mitigate neuronal suppression in specific regions, thereby accelerating network convergence and classification efficiency. Experimental results demonstrate the proposed residual network achieves 73.45% and 96.97% accuracy on the FER2013 and CK+ datasets, respectively.
Keywords
attention mechanism, residual network, ResNet50, facial expression recognition
[1]. Dominguez-Catena, I., Paternain, D., & Galar, M. (2024). Metrics for Dataset Demographic Bias: A Case Study on Facial Expression Recognition. IEEE Transactions on Pattern Analysis and Machine Intelligence, 46(8), 5209-5226. https://doi.org/10.1109/TPAMI.2024.3361979
[2]. Jan, N., Gwak, J., & Pamucar, D. (2023). A robust hybrid decision making model for human-computer interaction in the environment of bipolar complex picture fuzzy soft sets. Information Sciences, 645, 119163. https://doi.org/10.1016/j.ins.2023.119163
[3]. Li, Y., Zhang, Z., Chen, B., Lu, G., & Zhang, D. (2023). Deep Margin-Sensitive Representation Learning for Cross-Domain Facial Expression Recognition. IEEE Transactions on Multimedia, 25, 1359-1373. https://doi.org/10.1109/TMM.2022.3141604
[4]. Liu, D., Dai, W., Zhang, H., Jin, X., Cao, J., & Kong, W. (2023). Brain-Machine Coupled Learning Method for Facial Emotion Recognition. IEEE Transactions on Pattern Analysis and Machine Intelligence, 45(9), 10703-10717. https://doi.org/10.1109/TPAMI.2023.3257846
[5]. He, K., Zhang, X., Ren, S., & Sun, J. (2016). Deep residual learning for image recognition. In Proceedings of the IEEE Conference on Computer Vision and Pattern Recognition (pp. 770-778).
[6]. Cai, J., Meng, Z., Khan, A. S., Li, Z., O’Reilly, J., & Tong, Y. (2023). Probabilistic Attribute Tree Structured Convolutional Neural Networks for Facial Expression Recognition in the Wild. IEEE Transactions on Affective Computing, 14(3), 1927-1941. https://doi.org/10.1109/TAFFC.2022.3156920
[7]. Foody, G. M., & Mathur, A. (2004). A relative evaluation of multiclass image classification by support vector machines. IEEE Transactions on Geoscience and Remote Sensing, 42(6), 1335-1343. https://doi.org/10.1109/TGRS.2004.827806
[8]. Mohammadi, M. R., Fatemizadeh, E., & Mahoor, M. H. (2014). PCA-based dictionary building for accurate facial expression recognition via sparse representation. Journal of Visual Communication and Image Representation, 25(5), 1082-1092. https://doi.org/10.1016/j.jvcir.2014.03.011
[9]. Bougourzi, F., Dornaika, F., Mokrani, K., Taleb-Ahmed, A., & Ruichek, Y. (2020). Fusing Transformed Deep and Shallow features (FTDS) for image-based facial expression recognition. Expert Systems with Applications, 156, 113459. https://doi.org/10.1016/j.eswa.2020.113459
[10]. Zhang, F., Liu, Y., & Zhang, X. (2024). Low-dose CT image quality evaluation method based on radiomics and deep residual network with attention mechanism. Expert Systems with Applications, 238, 122268. https://doi.org/10.1016/j.eswa.2023.122268
[11]. Vaswani, A., Shazeer, N., Parmar, N., Uszkoreit, J., Jones, L., Gomez, A. N., Kaiser, Ł., & Polosukhin, I. (2017). Attention is all you need. Advances in Neural Information Processing Systems, 30.
Cite this article
Shang,Q. (2025). Facial Expression Recognition method based on residual network and attention mechanism. Advances in Engineering Innovation,16(4),45-50.
Data availability
The datasets used and/or analyzed during the current study will be available from the authors upon reasonable request.
Disclaimer/Publisher's Note
The statements, opinions and data contained in all publications are solely those of the individual author(s) and contributor(s) and not of EWA Publishing and/or the editor(s). EWA Publishing and/or the editor(s) disclaim responsibility for any injury to people or property resulting from any ideas, methods, instructions or products referred to in the content.
About volume
Journal:Advances in Engineering Innovation
© 2024 by the author(s). Licensee EWA Publishing, Oxford, UK. This article is an open access article distributed under the terms and
conditions of the Creative Commons Attribution (CC BY) license. Authors who
publish this series agree to the following terms:
1. Authors retain copyright and grant the series right of first publication with the work simultaneously licensed under a Creative Commons
Attribution License that allows others to share the work with an acknowledgment of the work's authorship and initial publication in this
series.
2. Authors are able to enter into separate, additional contractual arrangements for the non-exclusive distribution of the series's published
version of the work (e.g., post it to an institutional repository or publish it in a book), with an acknowledgment of its initial
publication in this series.
3. Authors are permitted and encouraged to post their work online (e.g., in institutional repositories or on their website) prior to and
during the submission process, as it can lead to productive exchanges, as well as earlier and greater citation of published work (See
Open access policy for details).