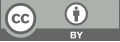
Target localization of abrasion-resistant color fastness samples based on YOLOv8 optimization and enhancement
- 1 Wenzhou University
* Author to whom correspondence should be addressed.
Abstract
To address the challenges in detecting abrasion-resistant color fastness samples – including limited sample instances, non-uniform shapes, and insufficiently distinct texture variations that compromise localization accuracy – this paper optimizes the detection framework through the integration of three key strategies: Global Attention Mechanism (GAM), Dynamic Sampling (DySample), and Adaptively Spatial Feature Fusion (ASFF), thereby enhancing detection accuracy and efficiency. Initially, Mosaic data augmentation is implemented to enrich dataset diversity and improve model robustness. Subsequently, the GAM attention mechanism is embedded into the backbone network to enhance target feature extraction capabilities. DySample replaces conventional upsampling methods in the neck network to achieve more effective feature reconstruction. Finally, the ASFF module is integrated into the Detect module within the head network to enable adaptively spatial weight learning for multi-scale feature map fusion. Compared with baseline algorithms, the improved framework demonstrates performance gains of 1.2% in Precision, 3.0% in Recall, 1.2% in mAP@0.5, and 13.5% in mAP@0.5:0.95. Experimental results validate the effectiveness of the proposed method, which maintains satisfactory performance across additional datasets, demonstrating strong robustness and superior generalization capability.
Keywords
target detection, YOLOv8, abrasion-resistant color fastness sample, convolutional neural network
[1]. Zhang, H., Tang, Y., & Zhou, W. (2022). Introductory Analysis of Colour Fastness to Textiles. Textile Testing and Standards, 8(04), 21-24-32.
[2]. Ruan, X., Lin, F., & Cui, G. (2022). Textile color fastness grading device (CN217277926U) [Utility Model Patent]. China National Intellectual Property Administration.
[3]. An, Y., Xue, W., & Ding, Y., (2022). Grading of color fastness to rubbing of textiles based on image processing. Journal of Textile Research, 43(12), 131-137.
[4]. Liu, Z. Y., Xu, H. Y., Zhu, X. Z., Li, C., Wang, Z. Y., Cao, Y. Q., & Dai, K. J. (2024). Bi-YOLO: An Improved Lightweight Object Detection Algorithm Based on YOLOv8n. Computer Engineering and Science, 46(08), 1444-1454.
[5]. Jiang, W., Wang, W., & Yang, J. (2024). AEM-YOLOv8s: Small target detection in UAV aerial images. Computer Engineering and Applications, 60(17), 191-202.
[6]. Zheng, L., Yi, J., He, P., Tie, J., Zhang, Y., Wu, W., & Long, L. (2024). Improvement of the YOLOv8 Model in the Optimization of the Weed Recognition Algorithm in Cotton Field. Plants, 13(13), 1843-1843.
[7]. Wu, T., Miao, Z., Huang, W., Han, W., Guo, Z., & Li, T. (2024). SGW-YOLOv8n: An Improved YOLOv8n-Based Model for Apple Detection and Segmentation in Complex Orchard Environments. Agriculture, 14(11), 1958-1958.
[8]. Ultralytics. (2023). YOLOv8 [Source code]. GitHub. Retrieved April 10, 2023, from https://github.com/ultralytics/ultralytics
[9]. Hu, J., Shen, L., & Sun, G. (2018). Squeeze-and-excitation networks. Proceedings of the 2018 IEEE/CVF Conference on Computer Vision and Pattern Recognition (pp. 7132-7141). IEEE. https://doi.org/10.1109/CVPR.2018.00745
[10]. Woo, S., Park, J., Lee, J.Y., & Kweon, I. S. (2018). CBAM: Convolutional block attention module. In V. Ferrari, M. Hebert, C. Sminchisescu, & Y. Weiss (Eds.), Computer vision - ECCV 2018: 15th European Conference on Computer Vision, Munich, Germany, September 8-14, 2018, Proceedings, Part VII (pp. 3-19). Springer. https://doi.org/10.1007/978-3-030-01234-2_1
[11]. Liu, S., Huang, D., & Wang, Y. (2019). Learning Spatial Fusion for Single-Shot Object Detection. In IEEE Computer Society Conference on Computer Vision and Pattern Recognition (CVPR). arXiv:1911.09516.
[12]. Liu, W., Lu, H., Fu, H., & Cao, Z. (2023). Learning to upsample by learning to sample. Proceedings of the 2023 IEEE/CVF International Conference on Computer Vision (ICCV) (pp. 6004-6014). IEEE. https://doi.org/10.1109/ICCV51070.2023.00554
[13]. Bochkovskiy, A., Wang, C., & Liao, H. M. (2020). YOLOv4: Optimal Speed and Accuracy of Object Detection. In Computer Science - Computer Vision and Pattern Recognition. arXiv:2004.10934.
[14]. Liu, W., Anguelov, D., Erhan, D., Szegedy, C., Reed, S., Fu, C.Y., & Berg, A. C. (2016). SSD: Single shot multiBox detector. In European Conference on Computer Vision (ECCV) (pp. 21-37).
[15]. Ren, S., He, K., Girshick, R., & Sun, J. (2017). Faster r-cnn: Towards real-time object detection with region proposal networks. IEEE Transactions on Pattern Analysis and Machine Intelligence, 39(6), 1137-1149.
[16]. Du, D., Zhu, P., Wen, L., Bian, X., Lin, H., & Hu, Q. (2019). VisDrone-DET2019: The Vision Meets Drone Object Detection in Image Challenge Results. In 2019 IEEE/CVF International Conference on Computer Vision Workshop (ICCVW) (pp. 213-226).
[17]. Li, Y., Shi, Z., & Hoffmann (2021). Global Attention Mechanism: Retain Information to Enhance Channel-Spatial Interactions. In IEEE/CVF Conference on Computer Vision and Pattern Recognition (CVPR). arXiv:2112.05561.
Cite this article
Cao,Y. (2025). Target localization of abrasion-resistant color fastness samples based on YOLOv8 optimization and enhancement. Advances in Engineering Innovation,16(4),98-105.
Data availability
The datasets used and/or analyzed during the current study will be available from the authors upon reasonable request.
Disclaimer/Publisher's Note
The statements, opinions and data contained in all publications are solely those of the individual author(s) and contributor(s) and not of EWA Publishing and/or the editor(s). EWA Publishing and/or the editor(s) disclaim responsibility for any injury to people or property resulting from any ideas, methods, instructions or products referred to in the content.
About volume
Journal:Advances in Engineering Innovation
© 2024 by the author(s). Licensee EWA Publishing, Oxford, UK. This article is an open access article distributed under the terms and
conditions of the Creative Commons Attribution (CC BY) license. Authors who
publish this series agree to the following terms:
1. Authors retain copyright and grant the series right of first publication with the work simultaneously licensed under a Creative Commons
Attribution License that allows others to share the work with an acknowledgment of the work's authorship and initial publication in this
series.
2. Authors are able to enter into separate, additional contractual arrangements for the non-exclusive distribution of the series's published
version of the work (e.g., post it to an institutional repository or publish it in a book), with an acknowledgment of its initial
publication in this series.
3. Authors are permitted and encouraged to post their work online (e.g., in institutional repositories or on their website) prior to and
during the submission process, as it can lead to productive exchanges, as well as earlier and greater citation of published work (See
Open access policy for details).