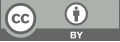
Exploring methods to make AI decisions more transparent and understandable for humans
- 1 Saint Leo University
* Author to whom correspondence should be addressed.
Abstract
As Artificial Intelligence (AI) systems increasingly weave into the fabric of diverse sectors, their intricate and often opaque decision-making processes pose challenges to users and stakeholders alike. The 'black box' nature of AI, especially deep learning models, highlights a pressing need for transparency and interpretability. This paper delves into the significance of making AI decisions transparent and provides a comprehensive exploration of methods aimed at demystifying AI processes. Through the lens of Explainable AI (XAI) and advanced visualization tools, we underscore the importance of bridging the chasm between sophisticated AI operations and human-centric understanding. By fostering transparency, it is anticipated that AI systems can not only enhance efficacy but also fortify trust, ensuring that decisions are both informed and explicable.
Keywords
artificial intelligence, explainable AI (XAI), transparency, decision-making processes, visualization tools
[1]. Russell, S. J., & Norvig, P. (2010). Artificial intelligence: a modern approach. Malaysia; Pearson Education Limited.
[2]. Castelvecchi, D. (2016). Can we open the black box of AI? Nature News, 538(7623), 20.
[3]. Doshi-Velez, F., & Kim, B. (2017). Towards a rigorous science of interpretable machine learning. arXiv preprint arXiv:1702.08608.
[4]. Ribeiro, M. T., Singh, S., & Guestrin, C. (2016). "Why should I trust you?" Explaining the predictions of any classifier. In Proceedings of the 22nd ACM SIGKDD international conference on knowledge discovery and data mining (pp. 1135-1144).
[5]. Goodfellow, I., Bengio, Y., & Courville, A. (2016). Deep learning. MIT press.
[6]. Gunning, D. (2017). Explainable artificial intelligence (xai). Defense Advanced Research Projects Agency (DARPA), nd Web.
[7]. Lundberg, S. M., & Lee, S. I. (2017). A unified approach to interpreting model predictions. In Advances in neural information processing systems (pp. 4765-4774).
[8]. Wattenberg, M., Viégas, F. B., & Borning, A. (2016). How to use t-SNE effectively. Distill.
[9]. Ribeiro, M. T., Singh, S., & Guestrin, C. (2016). "Why should I trust you?" Explaining the predictions of any classifier. Proceedings of the 22nd ACM SIGKDD international conference on knowledge discovery and data mining.
[10]. Wattenberg, M., Viégas, F., & Johnson, I. (2016). How to Use t-SNE Effectively. Distill.
[11]. Yosinski, J., Clune, J., Nguyen, A., Fuchs, T., & Lipson, H. (2015). Understanding neural networks through deep visualization. arXiv preprint arXiv:1506.06579.
[12]. Selvaraju, R. R., Cogswell, M., Das, A., Vedantam, R., Parikh, D., & Batra, D. (2017). Grad-CAM: Visual explanations from deep networks via gradient-based localization. Proceedings of the IEEE international conference on computer vision.
[13]. Lundberg, S. M., & Lee, S. I. (2017). A unified approach to interpreting model predictions. *Adv
Cite this article
MoDastoni,D.A. (2023). Exploring methods to make AI decisions more transparent and understandable for humans. Advances in Engineering Innovation,3,32-36.
Data availability
The datasets used and/or analyzed during the current study will be available from the authors upon reasonable request.
Disclaimer/Publisher's Note
The statements, opinions and data contained in all publications are solely those of the individual author(s) and contributor(s) and not of EWA Publishing and/or the editor(s). EWA Publishing and/or the editor(s) disclaim responsibility for any injury to people or property resulting from any ideas, methods, instructions or products referred to in the content.
About volume
Journal:Advances in Engineering Innovation
© 2024 by the author(s). Licensee EWA Publishing, Oxford, UK. This article is an open access article distributed under the terms and
conditions of the Creative Commons Attribution (CC BY) license. Authors who
publish this series agree to the following terms:
1. Authors retain copyright and grant the series right of first publication with the work simultaneously licensed under a Creative Commons
Attribution License that allows others to share the work with an acknowledgment of the work's authorship and initial publication in this
series.
2. Authors are able to enter into separate, additional contractual arrangements for the non-exclusive distribution of the series's published
version of the work (e.g., post it to an institutional repository or publish it in a book), with an acknowledgment of its initial
publication in this series.
3. Authors are permitted and encouraged to post their work online (e.g., in institutional repositories or on their website) prior to and
during the submission process, as it can lead to productive exchanges, as well as earlier and greater citation of published work (See
Open access policy for details).