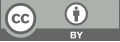
Medal prediction model based on machine learning and Bayes
- 1 College of Mathematics and Statistics, Liaoning University, Shenyang, China
* Author to whom correspondence should be addressed.
Abstract
The Olympic Games, organized by the International Olympic Committee, is the largest summer comprehensive games in the world, and its medal list has attracted much attention. The Olympic Games is a dynamic and complex system, and it is of extensive and far-reaching practical significance to establish a scientific and accurate prediction model for the competition results and to reveal the rules of medals. In this regard, this paper will address the following issues. For Problem 1, we first used Machine learning algorithms and Random Forest models. The goodness-of-fit index was used to judge the advantages and disadvantages of Random Forest, Logistic regression and XGBoost, and secondly, we predicted the number of medals won by each country and the number of medals won by each country in 2028, and with the help of the correlation analysis and the systematic clustering algorithm, we came up with the intrinsic connection between the host country, the amount of project changes and the amount of medal changes. For problem 2, we firstly adopt Bayesian Changepoint Detection monitoring model. We use Bayesian Changepoint Detection monitoring to determine the location of the effect point of "great coaches", then we use the factor of coach's contribution rate to determine the influence of coaches in national programs, and at the end of the question, we have conducted case studies on China, England and Brazil, and verified the reasonableness of the model by combining with the real situation in history. For question 3, we first summarized the model above, provided insights related to the Olympic medal count, and explained how each type of insight informs the Olympics. The host country's home field effect and international economic power were analyzed, and we thus made recommendations to the Olympics on infrastructure development, logistical experience, and so on, in order to provide for the next Olympic Games in Los Angeles, USA.
Keywords
machine learning, Random Forest, hierarchical clustering, Bayesian Changepoint Detection
[1]. Wikipedia contributors. (n.d.). Machine learning. In Wikipedia. Retrieved January 27, 2025, from https://en.wikipedia.org/wiki/Machine_learning
[2]. Sruthi, E. R. (2024). Understanding random forest algorithm with examples. Analytics Vidhya.
[3]. Omarzai, F. (2024). XGBoost regression in depth. Medium. https://medium.com/@fraidoonomarzai99/xgboost-regression-in-depth-cb2b3f623281
[4]. Wikipedia contributors. (n.d.). Hierarchical clustering. In Wikipedia. Retrieved November 11, 2024, from https://en.wikipedia.org/wiki/Hierarchical_clustering
[5]. Adams, R. P., & MacKay, D. J. C. (2007). Bayesian online changepoint detection [arXiv:0710.3742]. arXiv. https://doi.org/10.48550/arXiv.0710.3742
Cite this article
Liu,H. (2025). Medal prediction model based on machine learning and Bayes. Advances in Operation Research and Production Management,4(1),23-40.
Data availability
The datasets used and/or analyzed during the current study will be available from the authors upon reasonable request.
Disclaimer/Publisher's Note
The statements, opinions and data contained in all publications are solely those of the individual author(s) and contributor(s) and not of EWA Publishing and/or the editor(s). EWA Publishing and/or the editor(s) disclaim responsibility for any injury to people or property resulting from any ideas, methods, instructions or products referred to in the content.
About volume
Journal:Advances in Operation Research and Production Management
© 2024 by the author(s). Licensee EWA Publishing, Oxford, UK. This article is an open access article distributed under the terms and
conditions of the Creative Commons Attribution (CC BY) license. Authors who
publish this series agree to the following terms:
1. Authors retain copyright and grant the series right of first publication with the work simultaneously licensed under a Creative Commons
Attribution License that allows others to share the work with an acknowledgment of the work's authorship and initial publication in this
series.
2. Authors are able to enter into separate, additional contractual arrangements for the non-exclusive distribution of the series's published
version of the work (e.g., post it to an institutional repository or publish it in a book), with an acknowledgment of its initial
publication in this series.
3. Authors are permitted and encouraged to post their work online (e.g., in institutional repositories or on their website) prior to and
during the submission process, as it can lead to productive exchanges, as well as earlier and greater citation of published work (See
Open access policy for details).