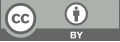
The Impact of Artificial Intelligence Applications on Corporate Labor Productivity
- 1 Beijing University of Posts and Telecommunications, Haidian District, Beijing, China
- 2 Beijing University of Posts and Telecommunications, Haidian District, Beijing, China
* Author to whom correspondence should be addressed.
Abstract
In the context of the rapid global development of artificial intelligence (AI), China is also actively advancing the research and application of related technologies. This paper focuses on Chinese A-share listed companies from 2016 to 2023 and explores the impact of corporate AI applications on labor productivity. A model is constructed in which labor productivity is measured by the natural logarithm of revenue per employee. The application indicator is built using the number of AI-related keywords in annual reports, with control variables set accordingly. Empirical results show that AI applications significantly improve labor productivity, with companies that exhibit good growth, strong cash flow, and large scale performing better in terms of productivity. Robustness checks confirm the validity of these conclusions. The study demonstrates that AI holds immense potential in corporate applications, and companies can build industrial ecosystems to promote its widespread use, enhancing labor productivity and contributing to high-quality economic development.
Keywords
artificial intelligence, labor productivity, labor force structure
[1]. Wang, L., Hu, S., & Dong, Z. (2022). Artificial intelligence technology, task attributes, and occupational substitutability risk: Micro-level empirical evidence. Social Sciences Digest, (08), 91-93.
[2]. Acemoglu, D., Autor, D., Hazell, J., et al. (2022). Artificial intelligence and jobs: Evidence from online vacancies. Journal of Labor Economics, 40(S1), S293-S340.
[3]. Brynjolfsson, E., Mitchell, T., & Rock, D. (2018). What can machines learn and what does it mean for occupations and the economy? In AEA Papers and Proceedings (Vol. 108, pp. 43-47). American Economic Association.
[4]. Zhu, Q., & Liu, H. (2020). Research on the income distribution effects of AI technology transformation: Frontier progress and review. Chinese Population Science, (02), 111-125+128.
[5]. Bessen, J., Goos, M., Salomons, A., et al. (2020). Automation: A guide for policymakers. Economic Studies at Brookings Institution, Washington, DC, USA.
[6]. Yu, L., Wei, X., Sun, Z., et al. (2021). Industrial robots, work tasks, and unconventional skill premiums: Evidence from manufacturing “enterprise-worker” matching survey. Management World, 37(01), 47-59+4. https://doi.org/10.19744/j.cnki.11-1235/f.2021.0004
[7]. Acemoglu, D., & Restrepo, P. (2018). The race between man and machine: Implications of technology for growth, factor shares, and employment. American Economic Review, 108(6), 1488-1542.
[8]. Arntz, M., Gregory, T., & Zierahn, U. (2017). Revisiting the risk of automation. Economics Letters, 159, 157-160.
[9]. Agrawal, A., Gans, J., & Goldfarb, A. (2022). Prediction machines, updated and expanded: The simple economics of artificial intelligence. Harvard Business Press.
[10]. Cai, Y., & Chen, N. (2019). Artificial intelligence, high-quality growth, and high-quality employment in the new technological revolution. Journal of Quantitative Economics and Technical Economics, 36(05), 3-22. https://doi.org/10.13653/j.cnki.jqte.2019.05.001
[11]. Guo, K. (2019). AI development, industrial structural transformation, and changes in labor income share. Management World, 35(07), 60-77+202-203. https://doi.org/10.19744/j.cnki.11-1235/f.2019.0092
[12]. Cheng, H., Chen, W., & Li, T. (2018). Robots in China: Current status, future, and impact — Evidence from the China Enterprise-Worker Matching Survey (CEES). Macro Quality Research, 6(03), 1-21. https://doi.org/10.13948/j.cnki.hgzlyj.2018.9.001
[13]. Yao, J., Zhang, K., Guo, L., et al. (2024). How can AI enhance corporate productivity? — A perspective from labor skill structure adjustment. Management World, 40(02), 101-116+133+117-122. https://doi.org/10.19744/j.cnki.11-1235/f.2024.0018
Cite this article
Feng,J.;Yuan,C. (2025). The Impact of Artificial Intelligence Applications on Corporate Labor Productivity. Journal of Applied Economics and Policy Studies,17,33-37.
Data availability
The datasets used and/or analyzed during the current study will be available from the authors upon reasonable request.
Disclaimer/Publisher's Note
The statements, opinions and data contained in all publications are solely those of the individual author(s) and contributor(s) and not of EWA Publishing and/or the editor(s). EWA Publishing and/or the editor(s) disclaim responsibility for any injury to people or property resulting from any ideas, methods, instructions or products referred to in the content.
About volume
Journal:Journal of Applied Economics and Policy Studies
© 2024 by the author(s). Licensee EWA Publishing, Oxford, UK. This article is an open access article distributed under the terms and
conditions of the Creative Commons Attribution (CC BY) license. Authors who
publish this series agree to the following terms:
1. Authors retain copyright and grant the series right of first publication with the work simultaneously licensed under a Creative Commons
Attribution License that allows others to share the work with an acknowledgment of the work's authorship and initial publication in this
series.
2. Authors are able to enter into separate, additional contractual arrangements for the non-exclusive distribution of the series's published
version of the work (e.g., post it to an institutional repository or publish it in a book), with an acknowledgment of its initial
publication in this series.
3. Authors are permitted and encouraged to post their work online (e.g., in institutional repositories or on their website) prior to and
during the submission process, as it can lead to productive exchanges, as well as earlier and greater citation of published work (See
Open access policy for details).