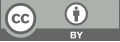
Measuring and analyzing carbon emission performance of Chinese national urban agglomerations: a static-dynamic integrated approach based on NDDF-GML
- 1 School of Economics & Management, Tongji University
* Author to whom correspondence should be addressed.
Abstract
Urban agglomerations play a pivotal role in China's carbon peaking and carbon neutrality goals, yet few studies have provided a unified, long-term assessment of their carbon emission performance. This paper addresses this gap by analyzing panel data (2006–2022) from 16 national-level urban agglomerations. Utilizing a Non-Radial Directional Distance Function (NDDF) to calculate the Carbon Reduction Efficiency Index (CREI) and a Global Malmquist-Luenberger (GML) index to measure Total Factor Carbon Emission Productivity (TFCEP), we reveal considerable disparities across regions. Eastern "optimization-enhancing" agglomerations (e.g., Pearl River Delta, Yangtze River Delta) demonstrate consistently high efficiency, sustained by stable technological advances. In contrast, central and western "growth-enhancing" and "development-nurturing" agglomerations (e.g., the Ningxia region along the Yellow River, Central Shanxi) exhibit lower performance but significant potential for improvement. Dynamic analysis indicates an overall upward trend, largely driven by technology gains in advanced regions and efficiency catch-up in less developed ones, despite challenges such as technological lock-in. Dagum's Gini coefficient shows narrowing gaps under coordinated the carbon peaking and the carbon neutrality goals policies, although institutional barriers still restrict cross-regional technology diffusion. These findings underscore the need for region-specific low-carbon strategies that integrate industrial upgrading and innovation support, thereby promoting balanced and sustainable urban development trajectories.
Keywords
carbon emissions, national urban agglomerations, regional disparities, non-radial directional distance function, GML index
[1]. Wang, Q., Wang, X., Li, R., et al. (2024). Reinvestigating the environmental Kuznets curve (EKC) of carbon emissions and ecological footprint in 147 countries: a matter of trade protectionism. Humanities and Social Sciences Communications, 11(1), 160.
[2]. Cheng, J., Yi, J., Dai, S., et al. (2019). Can low-carbon city construction facilitate green growth? Evidence from China's pilot low-carbon city initiative. Journal of Cleaner Production, 231, 1158-1170.
[3]. Wang, S., Wang, Z., Fang, C. (2021). Evolutionary characteristics and driving factors of carbon emission performance at the city level in China. Science China (Earth Sciences), 65(7), 1292-1307.
[4]. Yu, T., Shu, T., Xu, J. (2019). Spatial pattern, and evolution of China’s urban agglomerations. Frontiers of Urban and Rural Planning, 2(1), 7.
[5]. Ang, B. W. (1998). Is the energy intensity a less useful indicator than the carbon factor in the study of climate change? Energy Policy, 27(15), 943-946.
[6]. Mielnik, O., Goldemberg, J. (1999). Communication The evolution of the “carbonization index” in developing countries. Energy Policy, 27(5), 307-308.
[7]. Sun, J. W. (2005). The decrease of CO2 emission intensity is decarbonization at national and global levels. Energy Policy, 33(8), 975-978.
[8]. Färe, R., Grosskopf, S., Norris, M. (1994). Productivity growth, technical progress, and efficiency change in industrialized countries. American Economic Review, 84(1), 66-83.
[9]. Charnes, A., Cooper, W. W., Rhodes, E. (1978). Measuring the efficiency of decision making units. European Journal of Operational Research, 2(6), 429-444.
[10]. Banker, R. D., Charnes, A., Cooper, W. W. (1984). Some Models for Estimating Technical and Scale Inefficiencies in Data Envelopment Analysis. Management Science, 30(9), 1078-1092.
[11]. Färe, R., Grosskopf, S. (2010). Directional distance functions and slacks-based measures of efficiency. European Journal of Operational Research, 200(1), 320-322.
[12]. Zhou, P., Ang, B. W., Wang, H. (2012). Energy and CO2 emission performance in electricity generation: A non-radial directional distance function approach. European Journal of Operational Research, 221(3), 625-635.
[13]. Zhang, H., Feng, C., Zhou, X. (2020). Going carbon-neutral in China: Does the low-carbon city pilot policy improve carbon emission efficiency? Sustainable Production and Consumption, 33, 312-329.
[14]. Färe, R., Grosskopf, S., Tyteca, D. (1996). An activity analysis model of the environmental performance of firms—application to fossil-fuel-fired electric utilities. Ecological Economics, 18(2), 161-175.
[15]. Färe, R., Grosskopf, S., Pasurka, C. A. (2007). Environmental production functions and environmental directional distance functions. Energy, 32(7), 1055-1066.
[16]. Dong, F., Li, X., Long, R. (2013). Regional carbon emission performance in China according to a stochastic frontier model. Renewable and Sustainable Energy Reviews, 28, 525-530.
[17]. Zhou, P., Delmas, M. A., Kohli, A. (2017). Constructing meaningful environmental indices: A nonparametric frontier approach. Journal of Environmental Economics and Management, 85, 21-34.
[18]. Chambers, R. G., Chung, Y., Färe, R. (1996). Benefit and distance functions. J Econ Theory, 70(2), 407-419.
[19]. Shao, S., Fan, M., Lili, Y. (2021). Economic Restructuring, Green Technical Progress, and Low-Carbon Transition Development in China: An Empirical Investigation Based on the Overall Technology Frontier and Spatial Spillover Effect. Journal of Management World, 38(2), 46-69+4-10.
[20]. Zhang, N., Zhou, P., Choi, Y. (2013). Energy efficiency, CO2 emission performance and technology gaps in fossil fuel electricity generation in Korea: A meta-frontier non-radial directional distance function analysis. Energy Policy, 56, 653-662.
[21]. Chung, Y. H., Färe, R., Grosskopf, S. (1997). Productivity and Undesirable Outputs: A Directional Distance Function Approach. Journal of Environmental Management, 51(3), 229-240.
[22]. Oh, D.-H. (2010). A global Malmquist-Luenberger productivity index. Journal of Productivity Analysis, 34(3), 183-197.
[23]. Zhang, N., Choi, Y. (2013). Total-factor carbon emission performance of fossil fuel power plants in China: A metafrontier non-radial Malmquist index analysis. Energy Economics, 40, 549-559.
[24]. Wang, Z., Feng, C. (2015). Sources of production inefficiency and productivity growth in China: A global data envelopment analysis. Energy Economics, 49, 380-389.
[25]. Du, J., Chen, Y., Huang, Y. (2018). A Modified Malmquist-Luenberger Productivity Index: Assessing Environmental Productivity Performance in China. European Journal of Operational Research, 269(1), 171-187.
[26]. Zhang, N., Kong, F., Choi, Y. (2016). The effect of size-control policy on unified energy and carbon efficiency for Chinese fossil fuel power plants. Energy Policy, 70, 193-200.
[27]. Dagum, C. (1997). A new approach to the decomposition of the Gini income inequality ratio. Empirical Economics, 22(4), 515-531.
[28]. Shan, H. (2008). Reestimating the Capital Stock of China: 1952~2006. Journal of Quantitative & Technological Economics, 25(10), 17-31.
[29]. Wu, Y., Shi, K., Chen, Z. (2023). Developing Improved Time-Series DMSP-OLS-Like Data (1992–2019) in China by Integrating DMSP-OLS and SNPP-VIIRS. IEEE Transactions on Geoscience and Remote Sensing, 60, 1-14.
[30]. Wang, S., Fang, C., Sun, L. (2019). Decarbonizing China’s Urban Agglomerations. Annals of the American Association of Geographers, 109(1), 266-285.
[31]. Commission, E., Centre, J. R., Crippa, M., et al. (2022). GHG emissions of all world countries. Publications Office of the European Union.
Cite this article
Zhuang,R. (2025). Measuring and analyzing carbon emission performance of Chinese national urban agglomerations: a static-dynamic integrated approach based on NDDF-GML. Journal of Applied Economics and Policy Studies,18(2),61-76.
Data availability
The datasets used and/or analyzed during the current study will be available from the authors upon reasonable request.
Disclaimer/Publisher's Note
The statements, opinions and data contained in all publications are solely those of the individual author(s) and contributor(s) and not of EWA Publishing and/or the editor(s). EWA Publishing and/or the editor(s) disclaim responsibility for any injury to people or property resulting from any ideas, methods, instructions or products referred to in the content.
About volume
Journal:Journal of Applied Economics and Policy Studies
© 2024 by the author(s). Licensee EWA Publishing, Oxford, UK. This article is an open access article distributed under the terms and
conditions of the Creative Commons Attribution (CC BY) license. Authors who
publish this series agree to the following terms:
1. Authors retain copyright and grant the series right of first publication with the work simultaneously licensed under a Creative Commons
Attribution License that allows others to share the work with an acknowledgment of the work's authorship and initial publication in this
series.
2. Authors are able to enter into separate, additional contractual arrangements for the non-exclusive distribution of the series's published
version of the work (e.g., post it to an institutional repository or publish it in a book), with an acknowledgment of its initial
publication in this series.
3. Authors are permitted and encouraged to post their work online (e.g., in institutional repositories or on their website) prior to and
during the submission process, as it can lead to productive exchanges, as well as earlier and greater citation of published work (See
Open access policy for details).