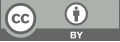
Artificial Intelligence in Legal Systems: Examining Gender Bias and the Role of UK Legal Frameworks in Addressing It
- 1 Benenden School
* Author to whom correspondence should be addressed.
Abstract
This study examines the gender discrimination of Artificial Intelligence (AI) used in the legal system, focusing on risk assessment, facial recognition, and decision-making and decision-support tools. The study delves into the use of AI in the legal system, examining how its reliance on historical data, under/over-representation, and homogeneity of development teams perpetuate existing gender biases. The study then analyses the implications of the United Kingdom General Data Protection Regulation (UK GDPR) and the proposed Data Protection and Digital Information (DPPI) Bill in addressing gender biases in AI. Nevertheless, the study finds the need for a more robust and proactive legal framework that addresses the root causes of these biases in the design and implementation of AI systems. The paper concludes by proposing a framework to effectively address gender bias in AI systems used in the legal system. The framework outlines explicit obligations across policymakers, companies, and end users to ensure the development and deployment of bias-free AI systems. Its role is to provide comprehensive guidelines and oversight mechanisms that promote proactive measures to prevent gender bias. The framework aims to create a more equitable legal environment for everyone.
Keywords
Artificial Intelligence, Gender Discrimination, UK GDPR, Automated Decision-Making, Policy Recommendations
[1]. Gender equality. (2013). https://www.judiciary.uk/wp-content/uploads/JCO/Documents/judicial-college/ETBB_Gender__finalised_.pdf
[2]. Baker Gillis, N. (2021, August 1). Sexism in the Judiciary: The Importance of Bias Definition in NLP and In Our Courts. ACLWeb; Association for Computational Linguistics. https://doi.org/10.18653/v1/2021.gebnlp-1.6
[3]. Barysė, D., & Sarel, R. (2023). Algorithms in the court: does it matter which part of the judicial decision-making is automated? Artificial Intelligence and Law, 32, 117–146. https://doi.org/10.1007/s10506-022-09343-6
[4]. Belenguer, L. (2022). AI bias: exploring discriminatory algorithmic decision-making models and the application of possible machine-centric solutions adapted from the pharmaceutical industry. AI and Ethics, 2(2). https://doi.org/10.1007/s43681-022-00138-8
[5]. Zafar, A. (2024). Balancing the scale: navigating ethical and practical challenges of artificial intelligence (AI) integration in legal practices. Discover Artificial Intelligence, 4(1). https://doi.org/10.1007/s44163-024-00121-8
[6]. UNESCO. (2024). Generative AI: UNESCO study reveals alarming evidence of regressive gender stereotypes. Unesco.org. https://www.unesco.org/en/articles/generative-ai-unesco-study-reveals-alarming-evidence-regressive-gender-stereotypes
[7]. Buolamwini, J., & Gebru, T. (2018). Gender Shades: Intersectional Accuracy Disparities in Commercial Gender Classification *. Proceedings of Machine Learning Research, 81(81), 77–91. https://proceedings.mlr.press/v81/buolamwini18a/buolamwini18a.pdf
[8]. Katyal, S. K. (2020). Private Accountability in an Age of Artificial Intelligence. In W. Barfield (Ed.), The Cambridge Handbook of the Law of Algorithms (pp. 47–106). chapter, Cambridge: Cambridge University Press.
[9]. Radicalisation Awareness Network. (2023). The missing gender-dimension in risk assessment Key outcomes. https://home-affairs.ec.europa.eu/system/files/2024-01/ran_missing_gender-dimension_in_risk_assessment_14112023_en.pdf
[10]. van Eijk, G. (2016). Socioeconomic marginality in sentencing: The built-in bias in risk assessment tools and the reproduction of social inequality. Punishment & Society, 19(4), 463–481. https://doi.org/10.1177/1462474516666282
[11]. Starr, S. B. (2015). The New Profiling. Federal Sentencing Reporter, 27(4), 229–236. https://doi.org/10.1525/fsr.2015.27.4.229
[12]. Primus, R. A. (2003). Equal Protection and Disparate Impact: Round Three. Harvard Law Review, 117(2), 493. https://doi.org/10.2307/3651947
[13]. US Supreme Court . (1976). Craig v. Boren, 429 U.S. 190. Justia Law. https://supreme.justia.com/cases/federal/us/429/190/
[14]. Drösser, C. (2017, December 22). In Order Not to Discriminate, We Might Have to Discriminate. Simons Institute for the Theory of Computing. https://www.droesser.net/en/2017/12/
[15]. Eckhouse, L., Lum, K., Conti-Cook, C., & Ciccolini, J. (2018). Layers of Bias: A Unified Approach for Understanding Problems With Risk Assessment. Criminal Justice and Behavior, 46(2), 185–209. https://doi.org/10.1177/0093854818811379
[16]. Skeem, J. L., Monahan, J., & Lowenkamp, C. T. (2016). Gender, Risk Assessment, and Sanctioning: The Cost of Treating Women Like Men. SSRN Electronic Journal. https://doi.org/10.2139/ssrn.2718460
[17]. Kim, P. (2022, October). Race-Aware Algorithms: Fairness, Nondiscrimination and Affirmative Action. California Law Review. https://www.californialawreview.org/print/race-aware-algorithms-fairness-nondiscrimination-and-affirmative-action
[18]. Directorate-General for Migration and Home Affairs. (2024, May 27). Improving risk assessment: Accounting for gender, May 2024. Migration and Home Affairs. https://home-affairs.ec.europa.eu/whats-new/publications/improving-risk-assessment-accounting-gender-may-2024_en
[19]. Women and Girls in the Justice System | Overview. (2020, August 13). Office of Justice Programs. https://www.ojp.gov/feature/women-and-girls-justice-system/overview#overview
[20]. Katyal, S., & Jung, J. (2021b). The Gender Panopticon: Artificial Intelligence, Gender, and Design Justice. SSRN Electronic Journal. https://doi.org/10.2139/ssrn.3760098
[21]. Waelen, R. A. (2022). The struggle for recognition in the age of facial recognition technology. AI and Ethics, 3(1). https://doi.org/10.1007/s43681-022-00146-8
[22]. Office, U. S. G. A. (2024, March 8). Facial Recognition Technology: Federal Law Enforcement Agency Efforts Related to Civil Rights and Training | U.S. GAO. Www.gao.gov. https://www.gao.gov/products/gao-24-107372
[23]. Lin, S.-H. (2000). An Introduction to Face Recognition Technology. Informing Science: The International Journal of an Emerging Transdiscipline, 3, 001–007. https://doi.org/10.28945/569
[24]. Schuetz, P. (2021). Fly in the Face of Bias: Algorithmic Bias in Law Enforcement’s Facial Recognition Technology and the Need for an Adaptive Legal Framework. Minnesota Journal of Law & Inequality, 39(1), 221–254. https://doi.org/10.24926/25730037.626
[25]. O’Connor, S., & Liu, H. (2023). Gender bias perpetuation and mitigation in AI technologies: challenges and opportunities. AI & SOCIETY, 39(4), 2045–2057. https://doi.org/10.1007/s00146-023-01675-4
[26]. Hill, K. (2023, August 6). Eight Months Pregnant and Arrested After False Facial Recognition Match. The New York Times. https://www.nytimes.com/2023/08/06/business/facial-recognition-false-arrest.html
[27]. Clayton, J. (2024, May 25). “I was misidentified as shoplifter by facial recognition tech.” BBC News; BBC News. https://www.bbc.co.uk/news/technology-69055945
[28]. Charles, M. (2012). Cecilia L. Ridgeway: Framed by Gender: How Gender Inequality Persists in the Modern World. European Sociological Review, 29(2), 408–410. https://doi.org/10.1093/esr/jcs074
[29]. Schwemmer, C., Knight, C., Bello-Pardo, E. D., Oklobdzija, S., Schoonvelde, M., & Lockhart, J. W. (2020). Diagnosing Gender Bias in Image Recognition Systems. Socius: Sociological Research for a Dynamic World, 6(6), 237802312096717. https://doi.org/10.1177/2378023120967171
[30]. What is automated individual decision-making and profiling? (2023, May 19). Ico.org.uk. https://ico.org.uk/for-organisations/uk-gdpr-guidance-and-resources/individual-rights/automated-decision-making-and-profiling/what-is-automated-individual-decision-making-and-profiling/
[31]. Richardson, R. (2021). Defining and Demystifying Automated Decision Systems. Social Science Research Network, 81(3).
[32]. Nadeem, A., Marjanovic, O., & Abedin, B. (2022). Gender bias in AI-based decision-making systems: a systematic literature review. Australasian Journal of Information Systems, 26(26). https://doi.org/10.3127/ajis.v26i0.3835
[33]. Veale, M., & Binns, R. (2017). Fairer machine learning in the real world: Mitigating discrimination without collecting sensitive data. Big Data & Society, 4(2), 205395171774353. https://doi.org/10.1177/2053951717743530
[34]. Johnson, K. N. (2019, November 14). Automating the Risk of Bias. Ssrn.com. https://ssrn.com/abstract=3486723
[35]. Ntoutsi, E., Fafalios, P., Gadiraju, U., Iosifidis, V., Nejdl, W., Vidal, M., Ruggieri, S., Turini, F., Papadopoulos, S., Krasanakis, E., Kompatsiaris, I., Kinder‐Kurlanda, K., Wagner, C., Karimi, F., Fernandez, M., Alani, H., Berendt, B., Kruegel, T., Heinze, C., & Broelemann, K. (2020). Bias in Data‐driven Artificial Intelligence systems—An Introductory Survey. WIREs Data Mining and Knowledge Discovery, 10(3). https://doi.org/10.1002/widm.1356
[36]. Mimi Onuoha . (2021, November 9). Notes on Algorithmic Violence. GitHub. https://github.com/MimiOnuoha/On-Algorithmic-Violence
[37]. Dastin, J. (2018, October 11). Insight - Amazon scraps secret AI recruiting tool that showed bias against women. Reuters. https://www.reuters.com/article/world/insight-amazon-scraps-secret-ai-recruiting-tool-that-showed-bias-against-women-idUSKCN1MK0AG/
[38]. Lambrecht, A., & Tucker, C. (2019). Algorithmic Bias? An Empirical Study of Apparent Gender-Based Discrimination in the Display of STEM Career Ads. Management Science, 65(7), 2966–2981.
[39]. Brooks, W. (2022). Artificial Bias: The Ethical Concerns of AI-Driven Dispute Resolution in Family Matters. Journal of Dispute Resolution, 2022(2). https://scholarship.law.missouri.edu/jdr/vol2022/iss2/9
[40]. Altman, M., Wood, A., & Vayena, E. (2018). A Harm-Reduction Framework for Algorithmic Fairness. IEEE Security & Privacy, 16(3), 34–45. https://doi.org/10.1109/msp.2018.2701149
[41]. GOV.UK. (2010). Equality Act 2010. Legislation.gov.uk; Gov.uk. https://www.legislation.gov.uk/ukpga/2010/15/contents
[42]. Human Rights Act 1998. (1998). Legislation.gov.uk. https://www.legislation.gov.uk/ukpga/1998/42/contents
[43]. ICO. (2023, May 19). What is the impact of Article 22 of the UK GDPR on fairness? Ico.org.uk. https://ico.org.uk/for-organisations/uk-gdpr-guidance-and-resources/artificial-intelligence/guidance-on-ai-and-data-protection/how-do-we-ensure-fairness-in-ai/what-is-the-impact-of-article-22-of-the-uk-gdpr-on-fairness/
[44]. ICO. (2023, May 19). What about fairness, bias and discrimination? Ico.org.uk. https://ico.org.uk/for-organisations/uk-gdpr-guidance-and-resources/artificial-intelligence/guidance-on-ai-and-data-protection/how-do-we-ensure-fairness-in-ai/what-about-fairness-bias-and-discrimination/
[45]. Erdos, D. (2022). A Bill for a Change? Analysing the UK Government’s Statutory Proposals on the Content of Data Protection and Electronic Privacy. SSRN Electronic Journal, 13. https://doi.org/10.2139/ssrn.4212420
[46]. How the new Data Bill waters down protections. (2023, November 28). Public Law Project. https://publiclawproject.org.uk/resources/how-the-new-data-bill-waters-down-protections/
[47]. McCullagh, K. (2023). Data Protection and Digital Sovereignty Post-Brexit. Bloomsburycollections.com. https://www.bloomsburycollections.com/monograph-detail?docid=b-9781509966516&tocid=b-9781509966516-chapter2
[48]. Raysa Benatti, Severi, F., Avila, S., & Colombini, E. L. (2024). Gender Bias Detection in Court Decisions: A Brazilian Case Study. 2022 ACM Conference on Fairness, Accountability, and Transparency, 67(3), 746–763. https://doi.org/10.1145/3630106.3658937
[49]. Bell, F., Bennett Moses, L., Legg, M., Silove, J., & Zalnieriute, M. (2022, June 14). AI Decision-Making and the Courts: A Guide for Judges, Tribunal Members and Court Administrators. Papers.ssrn.com. https://papers.ssrn.com/sol3/papers.cfm?abstract_id=4162985
[50]. Di Noia, T., Tintarev, N., Fatourou, P., & Schedl, M. (2022). Recommender systems under European AI regulations. Communications of the ACM, 65(4), 69–73. https://doi.org/10.1145/3512728
[51]. Award-winning project on preventing gender bias in AI systems used in judiciaries. (2021). United Nations : Office on Drugs and Crime. https://www.unodc.org/unodc/en/gender/news/award-winning-project-on-preventing-gender-bias-in-ai-systems-used-in-judiciaries.html
[52]. UNESCO. (2020). Artificial intelligence and gender equality: Key findings of UNESCO’s global dialogue. https://unesdoc.unesco.org/ark:/48223/pf0000374174
Cite this article
Huang,M. (2025). Artificial Intelligence in Legal Systems: Examining Gender Bias and the Role of UK Legal Frameworks in Addressing It. Lecture Notes in Education Psychology and Public Media,80,40-49.
Data availability
The datasets used and/or analyzed during the current study will be available from the authors upon reasonable request.
Disclaimer/Publisher's Note
The statements, opinions and data contained in all publications are solely those of the individual author(s) and contributor(s) and not of EWA Publishing and/or the editor(s). EWA Publishing and/or the editor(s) disclaim responsibility for any injury to people or property resulting from any ideas, methods, instructions or products referred to in the content.
About volume
Volume title: Proceedings of the 2nd International Conference on Global Politics and Socio-Humanities
© 2024 by the author(s). Licensee EWA Publishing, Oxford, UK. This article is an open access article distributed under the terms and
conditions of the Creative Commons Attribution (CC BY) license. Authors who
publish this series agree to the following terms:
1. Authors retain copyright and grant the series right of first publication with the work simultaneously licensed under a Creative Commons
Attribution License that allows others to share the work with an acknowledgment of the work's authorship and initial publication in this
series.
2. Authors are able to enter into separate, additional contractual arrangements for the non-exclusive distribution of the series's published
version of the work (e.g., post it to an institutional repository or publish it in a book), with an acknowledgment of its initial
publication in this series.
3. Authors are permitted and encouraged to post their work online (e.g., in institutional repositories or on their website) prior to and
during the submission process, as it can lead to productive exchanges, as well as earlier and greater citation of published work (See
Open access policy for details).