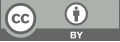
Exploring visual techniques for indoor intrusion detection using detectron2 and Faster RCNN
- 1 Dalian University of Technology
* Author to whom correspondence should be addressed.
Abstract
As societal evolution marches forward, there's an escalating emphasis on indoor security concerns. Within the safety realm, indoor intrusion detection emerges as a pivotal challenge, given its direct implications on safeguarding human lives and assets. Leveraging visual methods for indoor intrusion detection holds promising potential, particularly due to its straightforward deployment advantages. This study zeroes in on surveillance video footage, a prevalent medium in the security domain, as its experimental muse. Post an initial preprocessing phase utilizing a video difference map, the research introduces a pedestrian detection algorithm hinged on the synergy of detectron2 and Faster R-CNN. Insights gleaned reveal that this combined algorithm, when augmented with the video difference map preprocessing, exhibits commendable accuracy, robustness, and real-time efficacy on surveillance footage, especially in scenarios bereft of significant target occlusion. Moreover, this algorithm showcases an adeptness at discerning diminutively sized targets, demonstrating resilience against varying light magnitudes and maintaining impeccable accuracy amidst intricate lighting conditions. By harnessing this methodology, the enhancement of indoor environment safety monitoring becomes feasible, thereby bolstering the provision of dependable protection for individuals.
Keywords
indoor intrusion detection, vision, pedestrian detection, deep learning, surveillance video
[1]. Farheen C, Harald K. Detectron2 for Lesion Detection in Diabetic Retinopathy[J]. Algorithms, 2023,16(3).
[2]. Bobomirzaevich A A, Siful M B I,Rashid N, et al. An Improved Forest Fire Detection Method Based on the Detectron2 Model and a Deep Learning Approach[J]. Sensors, 2023, 23(3).
[3]. He Y,Xiaotang W, Yuhan L, et al. A new face detection method based on Faster RCNN [J]. Journal of Physics: Conference Series,2021,1754(1).
[4]. Hua W, Shifa J, Yang G. Improved Object Detection Algorithm Based on Faster RCNN [J]. Journal of Physics: Conference Series,2022,2395(1).
[5]. Hu L,Wei C, Yang X, et al. Special faster-RCNN for multi-objects detection[P]. International Workshop on Pattern Recognition,2018.
[6]. Leyuan Z, Bihui L,Chuanhui L. SHIP target image recognition based on FAST detector and faster-RCNN[P]. Naval Aeronautical and Astronautical Univ. (China),2021.
[7]. Zhang X,Qiu Z, Jiao L, et al. A method for centroid extraction based on Faster-RCNN [P]. International Symposium on Multispectral Image Processing and Pattern Recognition, 2020.
[8]. Lin Z, Guo Z, Yang J. Research on Texture Defect Detection Based on Faster-RCNN and Feature Fusion [P]. Machine Learning and Computing,2019.
[9]. Wei-Jie X,Shuaiqi L, Fei-Hong Y, et al. Disturbance recognition for -OTDR based on Faster-RCNN [P]. Southern Univ. of Science and Technology of China (China), 2022.
[10]. Bao J, Wei S,Lv J, et al. Optimized Faster-RCNN in Real-time Facial Expression Classification [C]//Advanced Science and Industry Research Center. Proceedings of 2019 2nd International Conference on Communication,Network and Artificial Intelligence(CNAI 2019). IOP Publishing, 2019:1012-1019.
Cite this article
Dong,C. (2024). Exploring visual techniques for indoor intrusion detection using detectron2 and Faster RCNN. Applied and Computational Engineering,36,230-236.
Data availability
The datasets used and/or analyzed during the current study will be available from the authors upon reasonable request.
Disclaimer/Publisher's Note
The statements, opinions and data contained in all publications are solely those of the individual author(s) and contributor(s) and not of EWA Publishing and/or the editor(s). EWA Publishing and/or the editor(s) disclaim responsibility for any injury to people or property resulting from any ideas, methods, instructions or products referred to in the content.
About volume
Volume title: Proceedings of the 2023 International Conference on Machine Learning and Automation
© 2024 by the author(s). Licensee EWA Publishing, Oxford, UK. This article is an open access article distributed under the terms and
conditions of the Creative Commons Attribution (CC BY) license. Authors who
publish this series agree to the following terms:
1. Authors retain copyright and grant the series right of first publication with the work simultaneously licensed under a Creative Commons
Attribution License that allows others to share the work with an acknowledgment of the work's authorship and initial publication in this
series.
2. Authors are able to enter into separate, additional contractual arrangements for the non-exclusive distribution of the series's published
version of the work (e.g., post it to an institutional repository or publish it in a book), with an acknowledgment of its initial
publication in this series.
3. Authors are permitted and encouraged to post their work online (e.g., in institutional repositories or on their website) prior to and
during the submission process, as it can lead to productive exchanges, as well as earlier and greater citation of published work (See
Open access policy for details).