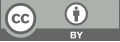
Dense-connected Stacked Hourglass Networks for Human Pose Estimation
- 1 McGill University
- 2 Georgia Institution of Technology
* Author to whom correspondence should be addressed.
Abstract
The main idea of this project is to try to improve the accuracy of human pose estimation in previous models. The new model proposed is based on the Stacked Hourglass Network with new structures added. The new structures ensured that the preservation of features of the original data by adding connections across the network, which we refer to as a “Dense-connected Stacked Hourglass” network, and we expected the new structure and the feature preserved could be helpful in the later stages because the Stacked Hourglass network pools down to very low resolution, during which important information may be lost. The data sets used in the project are MPII Human Pose and FLIC (Frames Labelled in Cinema). The final results show that the proposed architecture is able to improve the estimation accuracy to certain extend in identifying head, wrist and hip, while further studies on the architecture and improvements are still required.
Keywords
Stacked Hourglass, Human Pose Estimation, Machine Learning
[1]. Li, Z., Liu, F., Yang, W., Peng, S. and Zhou, J., 2021. A survey of convolutional neural networks: analysis, applications, and prospects. IEEE transactions on neural networks and learning systems.
[2]. Andriluka, M., Pishchulin, L., Gehler, P. and Schiele, B., 2014. 2d human pose estimation: New benchmark and state of the art analysis. In Proceedings of the IEEE Conference on computer Vision and Pattern Recognition (pp. 3686-3693).
[3]. Newell, A., Yang, K., Deng, J.: Stacked hourglass networks for human pose estimation. arXiv preprint arXiv:1603.06937 (2016)
[4]. Z. Cao, T. Simon, S. -E. Wei and Y. Sheikh, “Realtime Multi-person 2D Pose Estimation Using Part Affinity Fields,” 2017 IEEE Conference on Computer Vision and Pattern Recognition (CVPR), 2017, pp. 1302-1310, doi: 10.1109/CVPR.2017.143.
[5]. Toshev, A., Szegedy, C.: Deeppose: Human pose estimation via deep neural networks. In: Computer Vision and Pattern Recognition (CVPR), 2014 IEEE Conference on, IEEE (2014) 1653–1660
[6]. A Wei, S.E., Ramakrishna, V., Kanade, T., Sheikh, Y.: Convolutional pose machines. Computer Vision and Pattern Recognition (CVPR), 2016 IEEE Conference on (2016)
[7]. G. Huang, Z. Liu, L. Van Der Maaten and K. Q. Weinberger, “Densely Connected Convolutional Networks,” 2017 IEEE Conference on Computer Vision and Pattern Recognition (CVPR), 2017, pp. 2261-2269, doi: 10.1109/CVPR.2017.243.
[8]. Imambi, S., Prakash, K.B. and Kanagachidambaresan, G.R., 2021. PyTorch. Programming with TensorFlow: Solution for Edge Computing Applications, pp.87-104.
Cite this article
Liu,H.;Ding,C. (2024). Dense-connected Stacked Hourglass Networks for Human Pose Estimation. Applied and Computational Engineering,38,86-89.
Data availability
The datasets used and/or analyzed during the current study will be available from the authors upon reasonable request.
Disclaimer/Publisher's Note
The statements, opinions and data contained in all publications are solely those of the individual author(s) and contributor(s) and not of EWA Publishing and/or the editor(s). EWA Publishing and/or the editor(s) disclaim responsibility for any injury to people or property resulting from any ideas, methods, instructions or products referred to in the content.
About volume
Volume title: Proceedings of the 2023 International Conference on Machine Learning and Automation
© 2024 by the author(s). Licensee EWA Publishing, Oxford, UK. This article is an open access article distributed under the terms and
conditions of the Creative Commons Attribution (CC BY) license. Authors who
publish this series agree to the following terms:
1. Authors retain copyright and grant the series right of first publication with the work simultaneously licensed under a Creative Commons
Attribution License that allows others to share the work with an acknowledgment of the work's authorship and initial publication in this
series.
2. Authors are able to enter into separate, additional contractual arrangements for the non-exclusive distribution of the series's published
version of the work (e.g., post it to an institutional repository or publish it in a book), with an acknowledgment of its initial
publication in this series.
3. Authors are permitted and encouraged to post their work online (e.g., in institutional repositories or on their website) prior to and
during the submission process, as it can lead to productive exchanges, as well as earlier and greater citation of published work (See
Open access policy for details).