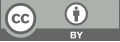
Application of artificial intelligence and machine learning in financial forecasting and trading
- 1 University of California
* Author to whom correspondence should be addressed.
Abstract
Artificial Intelligence (AI) and Machine Learning (ML) have been employed in the field of financial forecasting for many years. Existing scientific research has substantiated the effectiveness of AI/ML in the financial market. Currently, approximately 35% of the total capitalization of the US stock market is influenced by quantitative analysis, primarily consisting of AI/ML methodologies and their variants. This paper reviews classical advanced methodologies of AI/ML in financial forecasting and automated trading. Specifically, it focuses on discussing representative methods from three categories: statistical approach (including ARIMA-GARCH), machine learning approach (including SVM and LSTM), and the logistic approach (including the Fuzzy System). In detail, this paper delves into the fundamental aspects of each method and illustrates their effectiveness through existing results from relevant papers. The structure of this paper begins with the introduction of each method, followed by their applications, and a discussion of their pros and cons. Furthermore, this paper offers an outlook on the hotspots and prospects for the development of this research topic.
Keywords
Artificial Intelligence, Machine Learning, Financial Forecasting
[1]. Boming Huang, Yuxiang Huan, Li Da Xu, Lirong Zheng & Zhuo Zou, Automated trading systems statistical and machine learning methods and hardware implementation: a survey, Enterprise Information Systems, 2019, 13:1, 132-144
[2]. Rundo, Francesco, Francesca Trenta, Agatino Luigi di Stallo, and Sebastiano Battiato. Grid Trading System Robot (GTSbot): A Novel Mathematical Algorithm for Trading FX Market, Applied Sciences 2019, 9, 9: 1796.
[3]. C. N. Babu and B. E. Reddy, Selected Indian stock predictions using a hybrid ARIMA-GARCH model, 2014 International Conference on Advances in Electronics Computers and Communications, Bangalore, 2014, pp. 1-6.
[4]. Farah Hayati Mustapa and Mohd Tahir Ismail, J. Phys.: Conf. Ser. 2019, 1366 012130
[5]. Jair Cervantes, Farid Garcia-Lamont, Lisbeth Rodríguez-Mazahua, Asdrubal Lopez, A comprehensive survey on support vector machine classification: Applications, challenges and trends, Neurocomputing, 2020, 408, 189-215.
[6]. Madge, S. Predicting stock price direction using support Vector Machines. 2015, https://www.cs.princeton.edu/sites/default/files/uploads/saahil_madge.pdf
[7]. A. Fan and M. Palaniswami, Stock selection using support vector machines, International Joint Conference on Neural Networks. Proceedings, 2001,3. 1793-1798.
[8]. L. J. Cao and F. E. H. Tay, Support vector machine with adaptive parameters in financial time series forecasting, IEEE Transactions on Neural Networks, 2003, 14, 6, 1506-1518.
[9]. Adil Moghar, Mhamed Hamiche, Stock Market Prediction Using LSTM Recurrent Neural Network, Procedia Computer Science, 2020, 170, 1168-1173.
[10]. Yan, Y., & Yang, D. A stock trend forecast algorithm based on Deep Neural Networks. Scientific Programming. 2021, https://www.hindawi.com/journals/sp/2021/7510641/
[11]. Cohen, Gil. Algorithmic Trading and Financial Forecasting Using Advanced Artificial Intelligence Methodologies Mathematics 2022, 10, 18: 3302.
[12]. Chandar, S.K. Stock market prediction using subtractive clustering for a neuro fuzzy hybrid approach. Cluster Comput, 2019, 13159–13166.
[13]. Ayodele Ariyo Adebiyi, Aderemi Oluyinka Adewumi, Charles Korede Ayo, Comparison of ARIMA and Artificial Neural Networks Models for Stock Price Prediction, Journal of Applied Mathematics, 2014, Article ID 614342, 7 pages.
Cite this article
Zhu,G. (2024). Application of artificial intelligence and machine learning in financial forecasting and trading. Applied and Computational Engineering,43,1-7.
Data availability
The datasets used and/or analyzed during the current study will be available from the authors upon reasonable request.
Disclaimer/Publisher's Note
The statements, opinions and data contained in all publications are solely those of the individual author(s) and contributor(s) and not of EWA Publishing and/or the editor(s). EWA Publishing and/or the editor(s) disclaim responsibility for any injury to people or property resulting from any ideas, methods, instructions or products referred to in the content.
About volume
Volume title: Proceedings of the 2023 International Conference on Machine Learning and Automation
© 2024 by the author(s). Licensee EWA Publishing, Oxford, UK. This article is an open access article distributed under the terms and
conditions of the Creative Commons Attribution (CC BY) license. Authors who
publish this series agree to the following terms:
1. Authors retain copyright and grant the series right of first publication with the work simultaneously licensed under a Creative Commons
Attribution License that allows others to share the work with an acknowledgment of the work's authorship and initial publication in this
series.
2. Authors are able to enter into separate, additional contractual arrangements for the non-exclusive distribution of the series's published
version of the work (e.g., post it to an institutional repository or publish it in a book), with an acknowledgment of its initial
publication in this series.
3. Authors are permitted and encouraged to post their work online (e.g., in institutional repositories or on their website) prior to and
during the submission process, as it can lead to productive exchanges, as well as earlier and greater citation of published work (See
Open access policy for details).