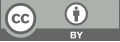
Stock price forecast model for CATL based on BP neural network regression
- 1 Tongji University
* Author to whom correspondence should be addressed.
Abstract
With the introduction of the "Dual Carbon" policy and the increasing environmental awareness among residents, the new energy vehicle industry is experiencing positive growth momentum. New energy vehicles use non-traditional energy sources as their power supply, effectively reducing carbon emissions, enhancing energy efficiency, and contributing to the improvement of China's existing energy landscape, thus supporting environmental protection and the early realization of "carbon peak" and "carbon neutrality" goals. Contemporary Amperex Technology Co., Ltd. (CATL), a prominent and competitive player in China's emerging clean energy industry, focuses on researching, developing, manufacturing, and marketing power battery and energy storage systems specifically designed for new energy vehicles. Moreover, in recent years, machine learning and deep learning have gained wide application in various domains, including stock price prediction and financial investment. This paper constructs a stock price prediction model for CATL based on a BP neural network regression, considering factors related to traditional energy, carbon trading, environmental aspects, and industry-specific factors.
Keywords
Stock Price Forecast, BP Neural Network Regression, CATL
[1]. Xu Z and Lu J 2023 Environmental regulation and new energy industry development: evolutionary logic and optimization path. Inner Mongolia Social Sciences vol 44 pp 124-130
[2]. Wang J and Long S 2022 Research on Unicorn Enterprise Value Assessment Based on EVA - Taking CATL as an Example. Operation and Management. vol 01 pp 102-107
[3]. Zeng H 2022 New energy stock price prediction model based on LightGBM-GRU. Southwest University, MA thesis.
[4]. Yuan W, and Zhou Z 2023 Research on factors affecting stock price fluctuations based on PCA-BP neural network.Trade Exhibition Economics vol 10 pp 99-101
[5]. Zhang N and Chen Y 2021 Research on stock price prediction based on neural network model. Changjiang Information and Communications vol 34 pp 61-63
[6]. Wang S 2008 Research on the application of BP neural network in stock prediction. Central South University, MA thesis.
[7]. Xu X and Yan G 2011 Analysis of stock price trend based on BP neural network. Zhejiang Finance. vol 11 pp57-59+64
[8]. Wang Y and Guo Y 2019 Application of improved XGBoost model in stock forecasting. Computer Engineering and Applications vol 55 pp 202-207
[9]. Yan Z, Qin C and Song G 2021 Random forest model stock price prediction based on Pearson feature selection. Computer Engineering and Applications vol 57.pp 286-296
[10]. Hong J 2017 Research on stock price trend prediction based on GBDT model. Jinan University, MA thesis.
[11]. Liu J, Ma Y and Li X. Research on the impact of the "double points" policy on the technological innovation of new energy vehicle companies. Scientific Research.
Cite this article
Dai,M. (2024). Stock price forecast model for CATL based on BP neural network regression. Applied and Computational Engineering,53,27-38.
Data availability
The datasets used and/or analyzed during the current study will be available from the authors upon reasonable request.
Disclaimer/Publisher's Note
The statements, opinions and data contained in all publications are solely those of the individual author(s) and contributor(s) and not of EWA Publishing and/or the editor(s). EWA Publishing and/or the editor(s) disclaim responsibility for any injury to people or property resulting from any ideas, methods, instructions or products referred to in the content.
About volume
Volume title: Proceedings of the 4th International Conference on Signal Processing and Machine Learning
© 2024 by the author(s). Licensee EWA Publishing, Oxford, UK. This article is an open access article distributed under the terms and
conditions of the Creative Commons Attribution (CC BY) license. Authors who
publish this series agree to the following terms:
1. Authors retain copyright and grant the series right of first publication with the work simultaneously licensed under a Creative Commons
Attribution License that allows others to share the work with an acknowledgment of the work's authorship and initial publication in this
series.
2. Authors are able to enter into separate, additional contractual arrangements for the non-exclusive distribution of the series's published
version of the work (e.g., post it to an institutional repository or publish it in a book), with an acknowledgment of its initial
publication in this series.
3. Authors are permitted and encouraged to post their work online (e.g., in institutional repositories or on their website) prior to and
during the submission process, as it can lead to productive exchanges, as well as earlier and greater citation of published work (See
Open access policy for details).