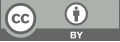
Exploration of hyperparameter efficiency for image style transfer
- 1 Dalian Maritime University
* Author to whom correspondence should be addressed.
Abstract
Image style transfer is a popular computer vision technique that aims to merge the content of one image with the style of another to generate a unique, original image with a different aesthetic feel. Numerous models have been developed for various applications in this field, including portrait painting, art creation, and medical image processing, where additional information or annotations could be added to medical images, making them easier to read and understand. This study focuses on optimizing parameters within the pre-trained Visual Geometry Group (VGG19) network architecture, building on Google Brain’s 2017 work on Arbitrary style transfer in one model. The goal is to improve the quality and realism of the generated images by exploring different parameter combinations and fine-tuning weights and learning rates. This work carefully selects a range of styles and content to compare their effects during the optimization process. Finally, this fine-tuning process strikes a balance between content loss and style loss, which results in high-quality and more realistic images.
Keywords
Image Style Transfer, Image Generation, Deep Learning
[1]. Jing, Y., Yang, Y., Feng, Z., Ye, J., Yu, Y., & Song, M. (2019). Neural style transfer: A review. IEEE transactions on visualization and computer graphics, 26(11), 3365-3385.
[2]. Gatys, L. A., Ecker, A. S., & Bethge, M. (2016). Image style transfer using convolutional neural networks. In Proceedings of the IEEE conference on computer vision and pattern recognition, 2414-2423.
[3]. Liu, L., Xi, Z., Ji, R., & Ma, W. (2019). Advanced deep learning techniques for image style transfer: a survey. Signal Processing: Image Communication, 78, 465-470.
[4]. Gatys, L. A., Ecker, A. S., & Bethge, M. (2015). A neural algorithm of artistic style. arXiv preprint arXiv:1508.06576.
[5]. Simonyan, K., & Zisserman, A. (2014). Very deep convolutional networks for large-scale image recognition. arXiv preprint arXiv:1409.1556.
[6]. Holden, D., Habibie, I., Kusajima, I., & Komura, T. (2017). Fast neural style transfer for motion data. IEEE computer graphics and applications, 37(4), 42-49.
[7]. Mateen, M., Wen, J., Nasrullah, Song, S., & Huang, Z. (2018). Fundus image classification using VGG-19 architecture with PCA and SVD. Symmetry, 11(1), 1.
[8]. Li, J., Wang, Q., Chen, H., An, J., & Li, S. (2020). A review on neural style transfer. In Journal of Physics: Conference Series, 1651(1), 012156.
[9]. Deng, J., Dong, W., Socher, R., Li, L. J., Li, K., & Fei-Fei, L. (2009). Imagenet: A large-scale hierarchical image database. In 2009 IEEE conference on computer vision and pattern recognition, 248-255.
[10]. Simon, M., Rodner, E., & Denzler, J. (2016). Imagenet pre-trained models with batch normalization. arXiv preprint arXiv:1612.01452.
Cite this article
Meng,Q. (2024). Exploration of hyperparameter efficiency for image style transfer. Applied and Computational Engineering,50,89-96.
Data availability
The datasets used and/or analyzed during the current study will be available from the authors upon reasonable request.
Disclaimer/Publisher's Note
The statements, opinions and data contained in all publications are solely those of the individual author(s) and contributor(s) and not of EWA Publishing and/or the editor(s). EWA Publishing and/or the editor(s) disclaim responsibility for any injury to people or property resulting from any ideas, methods, instructions or products referred to in the content.
About volume
Volume title: Proceedings of the 4th International Conference on Signal Processing and Machine Learning
© 2024 by the author(s). Licensee EWA Publishing, Oxford, UK. This article is an open access article distributed under the terms and
conditions of the Creative Commons Attribution (CC BY) license. Authors who
publish this series agree to the following terms:
1. Authors retain copyright and grant the series right of first publication with the work simultaneously licensed under a Creative Commons
Attribution License that allows others to share the work with an acknowledgment of the work's authorship and initial publication in this
series.
2. Authors are able to enter into separate, additional contractual arrangements for the non-exclusive distribution of the series's published
version of the work (e.g., post it to an institutional repository or publish it in a book), with an acknowledgment of its initial
publication in this series.
3. Authors are permitted and encouraged to post their work online (e.g., in institutional repositories or on their website) prior to and
during the submission process, as it can lead to productive exchanges, as well as earlier and greater citation of published work (See
Open access policy for details).