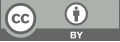
An improved BiGAN model for anomaly detection in finance
- 1 University of Warwick
* Author to whom correspondence should be addressed.
Abstract
Financial systems play a pivotal role in shaping contemporary society, and the detection of financial anomalies holds immense significance in mitigating the adverse repercussions of market uncertainties on the global economy. In this context, this study presents an innovative LSTM-GANs model, specifically crafted to enhance the detection of anomalies in financial stock markets. The model introduces an "Anomaly Score" as a pivotal metric, which is computed through a combination of factors such as Reconstruction Loss, Latent Space Distance, and Discriminator Score. This composite score provides a quantitative assessment of the anomaly level within the financial data. By applying a predefined threshold to this Anomaly Score, the model efficiently identifies and flags anomalies. In a world where financial markets are increasingly complex and prone to unexpected events, the ability to detect and respond to anomalies swiftly is paramount. This novel LSTM-GANs model offers a promising approach to bolster the accuracy and effectiveness of financial anomaly detection, thereby contributing to the stability and resilience of global financial systems.
Keywords
GAN, Anomaly detection, Quantitative finance
[1]. Eskin E 2000 Anomaly detection over noisy data using learned probability distributions
[2]. Blázquez-García A et al 2021 A review on outlier/anomaly detection in time series data. ACM Computing Surveys (CSUR) 54(3) 1-33
[3]. Goodfellow I 2014 Generative adversarial networks Advances in neural information processing systems
[4]. Eckerli F 2021 Generative Adversarial Networks in finance: an overview arXiv preprint arXiv:2106.06364.
[5]. Mattia F D 2019 A Survey on GANs for Anomaly Detection arXiv preprint arXiv:1906.11632.
[6]. Bashar M A 2023 ALGAN: Time Series Anomaly Detection with Adjusted-LSTM GAN. arXiv preprint arXiv:2308.06663
[7]. Zenati H Foo C S Lecouat B Manek G and Chandrasekhar V R 2018 EFFICIENT GAN-BASED ANOMALY DETECTION. ICLR.
[8]. Yu Y et al 2019 A review of recurrent neural networks: LSTM cells and network architectures. Neural computation 31(7) 1235-1270
[9]. Staudemeyer R C and Morris E R 2019 Understanding LSTM--a tutorial into long short-term memory recurrent neural networks arXiv preprint arXiv:1909.09586
[10]. Srivastava A et al 2017 Veegan: Reducing mode collapse in gans using implicit variational learning. Advances in neural information processing systems 30
Cite this article
Chen,G. (2024). An improved BiGAN model for anomaly detection in finance. Applied and Computational Engineering,53,90-95.
Data availability
The datasets used and/or analyzed during the current study will be available from the authors upon reasonable request.
Disclaimer/Publisher's Note
The statements, opinions and data contained in all publications are solely those of the individual author(s) and contributor(s) and not of EWA Publishing and/or the editor(s). EWA Publishing and/or the editor(s) disclaim responsibility for any injury to people or property resulting from any ideas, methods, instructions or products referred to in the content.
About volume
Volume title: Proceedings of the 4th International Conference on Signal Processing and Machine Learning
© 2024 by the author(s). Licensee EWA Publishing, Oxford, UK. This article is an open access article distributed under the terms and
conditions of the Creative Commons Attribution (CC BY) license. Authors who
publish this series agree to the following terms:
1. Authors retain copyright and grant the series right of first publication with the work simultaneously licensed under a Creative Commons
Attribution License that allows others to share the work with an acknowledgment of the work's authorship and initial publication in this
series.
2. Authors are able to enter into separate, additional contractual arrangements for the non-exclusive distribution of the series's published
version of the work (e.g., post it to an institutional repository or publish it in a book), with an acknowledgment of its initial
publication in this series.
3. Authors are permitted and encouraged to post their work online (e.g., in institutional repositories or on their website) prior to and
during the submission process, as it can lead to productive exchanges, as well as earlier and greater citation of published work (See
Open access policy for details).