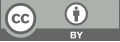
Machine learning-based hotel occupancy prediction
- 1 Wuhan University of Technology
* Author to whom correspondence should be addressed.
Abstract
The online booking system has tremendously facilitated people's ability to travel and make reservations because of the growth of the Internet. However, the unpredictability of travel led to frequent changes in reservations. Frequent order changes can lead to many problems, such as hotels not being able to get order changes in a timely manner and more in-demand customers not being able to stay, which can lead to lower profits and occupancy rates. In addition to this, there are a number of subjective, such as changes in the trip, reasons for work, and reasons for family, and objective, such as weather changes, natural disasters, and Transportation issues, factors that make it more difficult to predict the occupancy rate. In recent years, machine learning has become an increasingly valuable tool for researchers to analyze data. Based on these, this paper summarizes the machine learning algorithms related to hotel occupancy probability prediction and analyzes and compares them. Finally, it gives an outlook on the research of the hotel occupancy rate prediction.
Keywords
Un-subscription, Machine learning, Hotel occupancy prediction
[1]. Gustavo, Nuno. "Marketing management trends in tourism and hospitality industry: facing the 21st century environment." International Journal of Marketing Studies 5.3 (2013): 13.
[2]. Zhu, Fanwei, et al. "Modeling Price Elasticity for Occupancy Prediction in Hotel Dynamic Pricing." Proceedings of the 31st ACM International Conference on Information & Knowledge Management. (2022): 2
[3]. Casalo, Luis V., et al. "Do online hotel rating schemes influence booking behaviors?." International Journal of Hospitality Management 49 (2015): 28-36.
[4]. Albanese, Davide, et al. "mlpy: Machine learning python." arXiv preprint arXiv:1202.6548 (2012): 1-3
[5]. Kumari, Roshan, and Saurabh Kr Srivastava. "Machine learning: A review on binary classification." International Journal of Computer Applications 160.7 (2017): 2-4.
[6]. Zhang, Jialu, et al. "Succinct Explanations With Cascading Decision Trees." arXiv preprint arXiv:2010.06631 (2020): 4-8.
[7]. Churcher, Andrew, et al. "An experimental analysis of attack classification using machine learning in IoT networks." Sensors 21.2 (2021): 446.
[8]. Zhu, Fanwei, et al. "Modeling Price Elasticity for Occupancy Prediction in Hotel Dynamic Pricing." Proceedings of the 31st ACM International Conference on Information & Knowledge Management. (2022): 3-4.
[9]. Antonio, Nuno, Ana de Almeida, and Luis Nunes. "An automated machine learning based decision support system to predict hotel booking cancellations." Data Science Journal 18 (2019): 32-32.
[10]. Chen, Tianqi, and Carlos Guestrin. "Xgboost: A scalable tree boosting system." Proceedings of the 22nd acm sigkdd international conference on knowledge discovery and data mining. (2016): 785-794.
[11]. W. J. Zhang, G. Yang, Y. Lin, C. Ji and M. M. Gupta, "On Definition of Deep Learning," 2018 World Automation Congress (WAC), Stevenson, WA, USA, 2018, pp. 1-5.
Cite this article
Peng,J. (2024). Machine learning-based hotel occupancy prediction. Applied and Computational Engineering,46,151-158.
Data availability
The datasets used and/or analyzed during the current study will be available from the authors upon reasonable request.
Disclaimer/Publisher's Note
The statements, opinions and data contained in all publications are solely those of the individual author(s) and contributor(s) and not of EWA Publishing and/or the editor(s). EWA Publishing and/or the editor(s) disclaim responsibility for any injury to people or property resulting from any ideas, methods, instructions or products referred to in the content.
About volume
Volume title: Proceedings of the 4th International Conference on Signal Processing and Machine Learning
© 2024 by the author(s). Licensee EWA Publishing, Oxford, UK. This article is an open access article distributed under the terms and
conditions of the Creative Commons Attribution (CC BY) license. Authors who
publish this series agree to the following terms:
1. Authors retain copyright and grant the series right of first publication with the work simultaneously licensed under a Creative Commons
Attribution License that allows others to share the work with an acknowledgment of the work's authorship and initial publication in this
series.
2. Authors are able to enter into separate, additional contractual arrangements for the non-exclusive distribution of the series's published
version of the work (e.g., post it to an institutional repository or publish it in a book), with an acknowledgment of its initial
publication in this series.
3. Authors are permitted and encouraged to post their work online (e.g., in institutional repositories or on their website) prior to and
during the submission process, as it can lead to productive exchanges, as well as earlier and greater citation of published work (See
Open access policy for details).