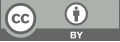
Harnessing AI and machine learning for enhanced credit risk analysis: A comprehensive exploration of computational techniques in the financial realm
- 1 University of Toronto
* Author to whom correspondence should be addressed.
Abstract
Within the confluence of the banking and financial sectors, the integration of machine learning in credit risk analysis signifies a paradigm shift towards data-centric decision-making. Historically, methodologies for credit risk were limited in predictive accuracy and computational efficiency. The advent of expansive language models, exemplified by Ant Group's AntFinGLM, offers a solution. These models, underpinned by deep learning, amalgamate financial texts and transactional data, facilitating the discernment of intricate financial paradigms and market nuances. This paper conducts a rigorous exploration of machine learning methodologies, from Bayesian classifiers to k-means clustering, offering an analytical perspective on their advantages and challenges. As the industry inclines towards innovations like AntFinGLM, the imperatives of professionalism, precision, and data sanctity gain significance. Upholding standards that encompass five dimensions and 28 categories, AntFinGLM epitomises these benchmarks, championing enhanced functionalities while fostering collaborative initiatives with financial entities. Addressing challenges, particularly around data security and professional integrity, becomes crucial. Techniques encompassing intent recognition, fact verification, and robust data protection mechanisms are indispensable. In summation, the endeavours of entities like AntFinGLM underscore the transformative prowess of expansive language models, ushering the financial sector into an epoch characterised by astute, efficient, and safeguarded decision-making paradigms.
Keywords
Machine Learning, Artificial Intelligence, Credit Risk, Financial Realm, AntFinGLM
[1]. Heart of the machine 2023 Ant Group opened a "volume" financial model, "four forces and one" to solve the real proposition of the industry https://www.jiqizhixin.com/articles/2023-09-09-4
[2]. Galindo J & Tamayo P 2000 Credit Risk Assessment Using Statistical and Machine Learning: Basic Methodology and Risk Modeling Applications. Computational Economics. 15(1/2) 107-143.
[3]. Khemakhem S, Ben Said F & Boujelbene Y 2018 Credit risk assessment for unbalanced datasets based on data mining, artificial neural network and support Vector Machines. Journal of Modelling in Management. 13(4) 932-951.
[4]. Abellán J & Castellano JG 2017 A comparative study on base classifiers in ensemble methods for credit scoring. Expert Systems with Applications. 73 1-10.
[5]. Goodell JW, Kumar S, Lim WM & Pattnaik D 2021 Artificial Intelligence and machine learning in finance: Identifying foundations, themes, and research clusters from Bibliometric analysis. Journal of Behavioral and Experimental Finance. 32(3) 100577.
[6]. Krichene A 2017 Using a naive bayesian classifier methodology for loan risk assessment. Journal of Economics, Finance and Administrative Science. 22(42) 3-24.
[7]. Milojević N & Redzepagic S 2021 Prospects of Artificial Intelligence and machine learning application in Banking Risk Management. Journal of Central Banking Theory and Practice. 10(3) 41-57.
[8]. Aziz S & Dowling M 2018 Machine learning and AI for Risk Management. Palgrave Studies in Digital Business & Enabling Technologies 33-50.
[9]. Liu W, Fan H & Xia M 2021 Step-wise multi-grained augmented gradient boosting decision trees for credit scoring. Engineering Applications of Artificial Intelligence. 97 104036.
[10]. Rabbani MR, Abdulla Y, Basahr A, Khan S & Moh’d Ali MA 2020 Embracing of Fintech in Islamic finance in the post Covid Era. 2020 International Conference on Decision Aid Sciences and Application (DASA) 1230-1234.
[11]. Trivedi SK 2020 A study on credit scoring modeling with different feature selection and machine learning approaches. Technology in Society. 63(3) 101413.
Cite this article
Li,X. (2024). Harnessing AI and machine learning for enhanced credit risk analysis: A comprehensive exploration of computational techniques in the financial realm. Applied and Computational Engineering,48,154-160.
Data availability
The datasets used and/or analyzed during the current study will be available from the authors upon reasonable request.
Disclaimer/Publisher's Note
The statements, opinions and data contained in all publications are solely those of the individual author(s) and contributor(s) and not of EWA Publishing and/or the editor(s). EWA Publishing and/or the editor(s) disclaim responsibility for any injury to people or property resulting from any ideas, methods, instructions or products referred to in the content.
About volume
Volume title: Proceedings of the 4th International Conference on Signal Processing and Machine Learning
© 2024 by the author(s). Licensee EWA Publishing, Oxford, UK. This article is an open access article distributed under the terms and
conditions of the Creative Commons Attribution (CC BY) license. Authors who
publish this series agree to the following terms:
1. Authors retain copyright and grant the series right of first publication with the work simultaneously licensed under a Creative Commons
Attribution License that allows others to share the work with an acknowledgment of the work's authorship and initial publication in this
series.
2. Authors are able to enter into separate, additional contractual arrangements for the non-exclusive distribution of the series's published
version of the work (e.g., post it to an institutional repository or publish it in a book), with an acknowledgment of its initial
publication in this series.
3. Authors are permitted and encouraged to post their work online (e.g., in institutional repositories or on their website) prior to and
during the submission process, as it can lead to productive exchanges, as well as earlier and greater citation of published work (See
Open access policy for details).