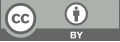
Application of big data analysis in water pollution monitoring
- 1 University of New South Wales
* Author to whom correspondence should be addressed.
Abstract
Contemporarily, the quick development of an abundant amount of big data analysis technologies has brought great convenience to individuals' everyday existence. In terms of environmental protection, especially water pollution monitoring, this technological progress is particularly critical. As the global demand for clean water resources grows and global industrialization intensifies pollution of the water environment, the adoption of advanced data analysis technologies has become critical. Among the vast array of machine learning architectures, three particularly stand out due to their significance and widespread adoption: the artificial neural network (ANN), which serves as a foundational pillar in understanding complex data patterns; the multi-layer perceptron neural network (MLPNN), a sophisticated evolution that allows for deeper computations and learning; and the adaptive neuro-fuzzy inference system (ANFIS), which brilliantly combines neural and fuzzy logic principles for intricate problem solving. These models not only have high accuracy due to their wide application, but they still have their own limitations. This article aims to introduce the methods, basic principles, and application scenarios of these models. In addition, this article also compares the advantages and limitations of these machine learning models, thereby providing some new ideas for future improvements and innovations in model algorithms, application scenarios, and integration.
Keywords
Big data, Machine learning, Water pollution, Monitoring
[1]. Ding X W, Dong X S, Hou B D, Fan G H and Zhang X Y 2021. J. Clean. Prod. vol 309 p 127398.
[2]. Farzin S, Chianeh F N, Anaraki M V and Mahmoudian F 2020. J. Clean. Prod. vol 266 p 122075.
[3]. Wang J, Zhao J, Lei X and Wang H 2018 Environ. Pollut. vol 241 pp 759–774.
[4]. Aeberhard M, Rauch S, Bahram M, Tanzmeister G, Thomas J, Pilat Y, Homm F, Huber W and Kaempchen N 2015 IEEE Trans. Parallel Distrib. Syst. vol 7(1) p 42e57.
[5]. Esteva A, Robicquet A, Ramsundar B, Kuleshov V, DePristo M, Chou K, Cui C,Corrado G, Thrun S and Dean J 2019 Nat. Med. vol 25 (1) p 24e29.
[6]. Zhu S and Heddam S 2020 Water Qual Res J vol 55 pp 106–118
[7]. Elkiran G, Nourani V and Abba S 2019 J Hydrol vol 577 p 123962
[8]. Haribowo R, Dermawan V and Fitrina H 2020 IOP Conf Ser: Earth Environ Sci vol 1 p 012003
[9]. Yetilmezsoy K, Ozkaya B and Cakmakci M 2011 Neural Netw World vol 21 p 193
[10]. Hasan R, Raghav A, Mahmood S and Hasan M A 2011 2011 International Conference on Information Management, Innovation Management and Industrial Engineering pp 491-495.
[11]. Allawi M F, Jaafar O, Hamzah F M, Abdullah S M S and El-shafe A 2018 Environ Sci Pollut Res vol 25 pp 13446–13469
[12]. Zaji A H and Bonakdari H 2019 ISH J Hydraul Eng vol 25 pp 316–324.
[13]. Ceccaroni L, Velickovski F, Blaas M, Wernand M R, Blauw A, Subirats L 2018 Earth Observ Open Sci Innov vol 15 pp 311–320.
[14]. Ighalo J O, Adeniyi A G and Marques G 2021 Model. Earth Syst. Environ. vol 7 pp 669–681.
[15]. Elkiran G, Nourani V, Abba S I and Abdullahi J 2018 Global J. Environ.Sci. Manage. vol 4(4), pp 439–450.
Cite this article
Zhou,C. (2024). Application of big data analysis in water pollution monitoring. Applied and Computational Engineering,53,173-180.
Data availability
The datasets used and/or analyzed during the current study will be available from the authors upon reasonable request.
Disclaimer/Publisher's Note
The statements, opinions and data contained in all publications are solely those of the individual author(s) and contributor(s) and not of EWA Publishing and/or the editor(s). EWA Publishing and/or the editor(s) disclaim responsibility for any injury to people or property resulting from any ideas, methods, instructions or products referred to in the content.
About volume
Volume title: Proceedings of the 4th International Conference on Signal Processing and Machine Learning
© 2024 by the author(s). Licensee EWA Publishing, Oxford, UK. This article is an open access article distributed under the terms and
conditions of the Creative Commons Attribution (CC BY) license. Authors who
publish this series agree to the following terms:
1. Authors retain copyright and grant the series right of first publication with the work simultaneously licensed under a Creative Commons
Attribution License that allows others to share the work with an acknowledgment of the work's authorship and initial publication in this
series.
2. Authors are able to enter into separate, additional contractual arrangements for the non-exclusive distribution of the series's published
version of the work (e.g., post it to an institutional repository or publish it in a book), with an acknowledgment of its initial
publication in this series.
3. Authors are permitted and encouraged to post their work online (e.g., in institutional repositories or on their website) prior to and
during the submission process, as it can lead to productive exchanges, as well as earlier and greater citation of published work (See
Open access policy for details).