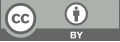
Sentiment analysis of Twitter user text based on the BERT model
- 1 East China University of Technology
* Author to whom correspondence should be addressed.
Abstract
Deep Neural Networks (DNNs) utilizing Recurrent Neural Network (RNN) architectures have found extensive application in text sentiment analysis. A prevailing notion suggests that augmenting the model's capacity can significantly improve accuracy and overall model performance. Building upon this premise, this paper advocates the adoption of a larger BERT model for text sentiment analysis. Bidirectional Encoder Representations from Transformers (BERT) is a sophisticated pre-trained language comprehension model that leverages Transformers as feature extractors. However, as the amount of model data increases, exceeding the memory limitations of a single GPU, algorithm optimization becomes crucial. Therefore, this paper employs two methods, namely data parallelism and GPipe parallelism, to accelerate and optimize the BERT model. Compared to a single GPU, training speed almost linearly increases with the addition of more GPUs. In addition, this research investigates the accuracy of the most advanced language model, chatgpt, by reannotating the dataset. During training, it was observed that the accuracy of the chatgpt-annotated dataset significantly declined in both RNN and BERT models. This indicates that chatgpt still exhibits some errors in sentiment text analysis.
Keywords
BERT, Sentiment analysis, Data optimization
[1]. Saon G Tüske Z Bolanos D et al 2021 Advancing RNN transducer technology for speech recognition. ICASSP 2021-2021 IEEE International Conference on Acoustics Speech and Signal Processing (ICASSP) IEEE: 5654-5658
[2]. Yadav S P Zaidi S Mishra A et al 2022 Survey on machine learning in speech emotion recognition and vision systems using a recurrent neural network (RNN) Archives of Computational Methods in Engineering 29(3): 1753-1770
[3]. Prabha M I 2019 Srikanth G U. Survey of sentiment analysis using deep learning techniques 2019 1st international conference on innovations in information and communication technology (ICIICT). IEEE: 1-9
[4]. Von Oswald J Niklasson E Randazzo E et al 2023 Transformers learn in-context by gradient descent International Conference on Machine Learning PMLR: 35151-35174
[5]. Alaparthi S Mishra M 2020 Bidirectional Encoder Representations from Transformers (BERT): A sentiment analysis odyssey arXiv preprint arXiv:2007.01127
[6]. Devlin J Chang M W Lee K et al 2018 Bert: Pre-training of deep bidirectional transformers for language understanding arXiv preprint arXiv:1810.04805
[7]. Lin T Wang Y Liu X et al 2022 A survey of transformers AI Open
[8]. Huang Y Cheng Y Bapna A et al 2019 Gpipe: Efficient training of giant neural networks using pipeline parallelism Advances in neural information processing systems 32
[9]. Go A Bhayani R Huang L 2009 Twitter sentiment classification using distant supervision CS224N project report Stanford 1(12): 2009
[10]. Kaggle 2017 Sentiment140 dataset with 1.6 million tweets https://www.kaggle.com/datasets/kazanova/sentiment140
Cite this article
Zhou,C. (2024). Sentiment analysis of Twitter user text based on the BERT model. Applied and Computational Engineering,52,102-108.
Data availability
The datasets used and/or analyzed during the current study will be available from the authors upon reasonable request.
Disclaimer/Publisher's Note
The statements, opinions and data contained in all publications are solely those of the individual author(s) and contributor(s) and not of EWA Publishing and/or the editor(s). EWA Publishing and/or the editor(s) disclaim responsibility for any injury to people or property resulting from any ideas, methods, instructions or products referred to in the content.
About volume
Volume title: Proceedings of the 4th International Conference on Signal Processing and Machine Learning
© 2024 by the author(s). Licensee EWA Publishing, Oxford, UK. This article is an open access article distributed under the terms and
conditions of the Creative Commons Attribution (CC BY) license. Authors who
publish this series agree to the following terms:
1. Authors retain copyright and grant the series right of first publication with the work simultaneously licensed under a Creative Commons
Attribution License that allows others to share the work with an acknowledgment of the work's authorship and initial publication in this
series.
2. Authors are able to enter into separate, additional contractual arrangements for the non-exclusive distribution of the series's published
version of the work (e.g., post it to an institutional repository or publish it in a book), with an acknowledgment of its initial
publication in this series.
3. Authors are permitted and encouraged to post their work online (e.g., in institutional repositories or on their website) prior to and
during the submission process, as it can lead to productive exchanges, as well as earlier and greater citation of published work (See
Open access policy for details).