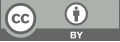
Stock price prediction for Google based on LSTM model with sentiment analysis
- 1 Heilongjiang University
* Author to whom correspondence should be addressed.
Abstract
Data analytics is increasingly widely used in economic and financial fields, with one of the more important applications being the prediction of stock price changes. However, the prediction of stock price changes is challenging because stock price changes are often uncertain and affected by multiple factors. This study is designed to use the LSTM model to predict stock price changes, and in the construction of the model to consider the psychological and emotional changes of investors, adding a sentiment analysis, combined with the sentiment index obtained from the sentiment analysis and the original stock price data as the input data for the prediction model. During the experiment, a comparison experiment was set up, i.e., only using the basic LSTM prediction model to predict stock price changes and the improved LSTM prediction model with the sentiment index obtained from the added sentiment analysis to predict stock price changes. After the comparison, the prediction results obtained by the LSTM model with the addition of sentiment analysis are more accurate, which on the one hand indicates that the change of investors' psychological sentiment will have an impact on the stock price change, and indicates that the prediction results obtained by the prediction model that considers the change of investors' sentiment are more accurate. The improved LSTM prediction model can help investors to effectively avoid possible risks when investing in stocks and thus gain more profit.
Keywords
Stock price prediction, LSTM, sentiment analysis
[1]. Fama E F 1998 Long-term returns, and Behavioral finance. J Financ Econ vol 49(3) pp 283-306
[2]. De Gooijer J G, Hyndman R J 2005 Selective Scrutiny. Soc Sci Electron Publ vol 22(3) pp 443-473.
[3]. Neild S 2003 Methods of time-frequency for structural quiver analysis. Eng Struct vol 25(6) pp 713-728.
[4]. Krunz M M and Makowski A M 2002 A compromise between Markovian and LRD models in modeling video traffic. IEEE J Sel Areas Commun vol 16(5) pp 733-748.
[5]. Farina L and Rinaldi S 2000 Theory and application of positive linear systems. J Vet Med Sci vol 63(9) pp 945-948 .
[6]. Contreras J, Espinola R, Nogales F J, et al. 2002 Predict electricity prices in the following day with ARIMA model. IEEE Power Eng Rev vol 22(9) pp 57-57.
[7]. Smola A J and SchÖlkopf B 2004 A supporting properties tutorial on vector regression. Stat Comput vol 14(3)pp 199-222.
[8]. Judith E and Deleo J M 2001 Artificial neural networks. Cancer vol 91(S8) pp 1615-1635.
[9]. Prasaddas S and Padhy S 2012 Future prices trends of Indian stock market based on support vector machine. Int J Comput Appl vol 41(3) pp 22-26.
[10]. Cavalcante R C, Brasileiro R C, Souza V L F, Nobrega J P and Oliveira A L I 2016 Future directions in the fields of computational intelligence and financial markets. Expert Syst Appl vol 55 pp 194-211.
[11]. Antweiler W and Frank M Z 2004 Is the information on the internet stock information review board just noise? J Finance vol 59(3) p 1259.
[12]. Baker M and Wurgler J 2006 The connection between investors sentiment towards investing in stocks and the returns they generate from investing in stocks. J Finance vol 61 pp 1645-1680.
[13]. Hochreiter S and Schmidhuber J 1997 Long short-term memory. Neural Comput vol 9(8) pp 1735-1780.
[14]. Xing S H, Chen Z, Wang H, Yeung D Y, Wong W K and Woo W C 2015 Machine learning method for predicting precipitation based on LSTM model. Advances in neural information processing systems pp 802-810.
[15]. Fischer T and Krauss C 2017 Predicting the trend of financial markets using deep learning method with LSTM model. Eur J Oper Res. vol S037 p 7221717310652.
[16]. Mao Y, Wang B, Wei W and Liu B 2012 The interaction between twitter data and S&P 500 stocks. Proceedings of the first ACM international workshop on hot topics on interdisciplinary social networks research vol 12(16) pp 69-72.
[17]. Zhou Z, Zhao J and Xu K 2016 Can the Chinese stock trading market be influenced by the investment sentiment of online investors? WISE lecture notes in computer Science vol 10041.
[18]. Antweiler W and Frank M Z 2004 Is the information on the internet stock information review board just noise? J Finance vol 59 pp 1259-1294.
Cite this article
Liu,Y. (2024). Stock price prediction for Google based on LSTM model with sentiment analysis. Applied and Computational Engineering,54,90-97.
Data availability
The datasets used and/or analyzed during the current study will be available from the authors upon reasonable request.
Disclaimer/Publisher's Note
The statements, opinions and data contained in all publications are solely those of the individual author(s) and contributor(s) and not of EWA Publishing and/or the editor(s). EWA Publishing and/or the editor(s) disclaim responsibility for any injury to people or property resulting from any ideas, methods, instructions or products referred to in the content.
About volume
Volume title: Proceedings of the 4th International Conference on Signal Processing and Machine Learning
© 2024 by the author(s). Licensee EWA Publishing, Oxford, UK. This article is an open access article distributed under the terms and
conditions of the Creative Commons Attribution (CC BY) license. Authors who
publish this series agree to the following terms:
1. Authors retain copyright and grant the series right of first publication with the work simultaneously licensed under a Creative Commons
Attribution License that allows others to share the work with an acknowledgment of the work's authorship and initial publication in this
series.
2. Authors are able to enter into separate, additional contractual arrangements for the non-exclusive distribution of the series's published
version of the work (e.g., post it to an institutional repository or publish it in a book), with an acknowledgment of its initial
publication in this series.
3. Authors are permitted and encouraged to post their work online (e.g., in institutional repositories or on their website) prior to and
during the submission process, as it can lead to productive exchanges, as well as earlier and greater citation of published work (See
Open access policy for details).