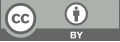
The investigation of symptom to disease chatbot based on LLAMA-2
- 1 Northwest University
* Author to whom correspondence should be addressed.
Abstract
In the swiftly advancing realm of Artificial Intelligence (AI), there has been a remarkable evolution in Natural Language Processing (NLP). Language models like ChatGPT have ushered in a new era of human-computer interaction, bestowing upon people an unparalleled capacity to comprehend and generate text that mimics human language. When these models are fine-tuned for specific domains, such as medicine, they become endowed with domain-specific knowledge, bridging the chasm between language comprehension and specialized expertise. However, a noteworthy research gap exists when it comes to harnessing AI-powered NLP for medical diagnosis and symptom analysis, particularly in the development of a Symptom to Disease Chatbot. This innovative approach aims to provide accurate and accessible healthcare information, enhancing the initial steps of medical consultation. This research proposes and develops a pioneering Symptom to Disease Chatbot, powered by the sophisticated Llama 2 13b pre-trained language model. By integrating extensive medical knowledge and AI capabilities, this chatbot seeks to empower individuals to make informed decisions about their health, potentially transforming personal health management. The research includes data preparation involving curated medical datasets, transforming them into a conversational format suitable for training. The base model, Llama 2, is fine-tuned for this medical context. While preliminary results are promising, further exploration with larger datasets is essential to enhance performance. Additionally, an open-source Gradio interface enhances user interaction. This research addresses the critical need for accessible healthcare information and demonstrates the potential of AI-powered language models in the healthcare sector.
Keywords
Artificial Intelligence, Natural Language Processing, Medical Diagnosis, Llama 2, Healthcare Accessibility
[1]. Floridi L and Chiriatti M 2020 GPT-3: Its nature, scope, limits, and consequences. Minds and Machines, 30, 681-694.
[2]. Touvron H et al 2023 Llama 2: Open foundation and fine-tuned chat models. arXiv preprint arXiv:2307.09288.
[3]. Ujjwal J 2023 diseases https://www.kaggle.com/datasets/jindalujjwal0720/diseases
[4]. Niyar R B 2023 Symptom2Disease, https://www.kaggle.com/datasets/niyarrbarman/symptom2disease
[5]. Dettmers T 2023 Qlora: Efficient finetuning of quantized llms. arXiv preprint arXiv:2305.14314.
[6]. UD 2023 Instruction fine-tuning Llama 2 with PEFT's QLoRa method, https://ukey.co/blog/finetune-llama-2-peft-qlora-huggingface/
[7]. philschmid 2023 Efficient Large Language Model training with LoRA and Hugging Face, https://www.philschmid.de/fine-tune-flan-t5-peft
[8]. baeldung 2023 Training and Validation Loss in Deep Learning, https://www.baeldung.com/cs/training-validation-loss-deep-learning
[9]. gradio 2023 Quickstart, https://www.gradio.app/guides/quickstart
[10]. Qiu Y et al 2022 Pose-guided matching based on deep learning for assessing quality of action on rehabilitation training Biomedical Signal Processing and Control 72 103323
Cite this article
Xu,N. (2024). The investigation of symptom to disease chatbot based on LLAMA-2. Applied and Computational Engineering,55,39-43.
Data availability
The datasets used and/or analyzed during the current study will be available from the authors upon reasonable request.
Disclaimer/Publisher's Note
The statements, opinions and data contained in all publications are solely those of the individual author(s) and contributor(s) and not of EWA Publishing and/or the editor(s). EWA Publishing and/or the editor(s) disclaim responsibility for any injury to people or property resulting from any ideas, methods, instructions or products referred to in the content.
About volume
Volume title: Proceedings of the 4th International Conference on Signal Processing and Machine Learning
© 2024 by the author(s). Licensee EWA Publishing, Oxford, UK. This article is an open access article distributed under the terms and
conditions of the Creative Commons Attribution (CC BY) license. Authors who
publish this series agree to the following terms:
1. Authors retain copyright and grant the series right of first publication with the work simultaneously licensed under a Creative Commons
Attribution License that allows others to share the work with an acknowledgment of the work's authorship and initial publication in this
series.
2. Authors are able to enter into separate, additional contractual arrangements for the non-exclusive distribution of the series's published
version of the work (e.g., post it to an institutional repository or publish it in a book), with an acknowledgment of its initial
publication in this series.
3. Authors are permitted and encouraged to post their work online (e.g., in institutional repositories or on their website) prior to and
during the submission process, as it can lead to productive exchanges, as well as earlier and greater citation of published work (See
Open access policy for details).