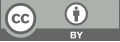
Predict Amazon stock by SVM and Random Forest
- 1 University of Southampton
* Author to whom correspondence should be addressed.
Abstract
The inherent uncertainties of market dynamics, such as economic data, geopolitics, and natural calamities, make stock market prediction extremely difficult. One increasingly effective method for handling this complexity is machine learning. Using data from the world's largest e-commerce and technology company, Amazon, this study concentrated on supervised machine learning models for stock market prediction. The most successful model was Support Vector Machine (SVM), which achieved an amazing prediction accuracy of 89.11%. Furthermore, Principal Component Analysis (PCA) significantly improved Random Forest's accuracy, enhancing it from 75.25% to 87.13%. In addition, the results show that the SVM outperforms the random forest no matter the PCA is considered. These results underscore SVM's importance in stock price prediction and PCA's value in enhancing Random Forest's performance. This research provides valuable insights into machine learning's role in financial forecasting, empowering investors and decision-makers to make informed choices in the ever-evolving stock market landscape.
Keywords
Amazon, SVM, Random Forest
[1]. Patel, R., Choudhary, V., Saxena, D., & Singh, A. K. (2021, June). Review of stock prediction using machine learning techniques. In 2021 5th International Conference on Trends in Electronics and Informatics (ICOEI) (pp. 840-846). IEEE.
[2]. Lawal, Z. K., Yassin, H., & Zakari, R. Y. (2020, December). Stock market prediction using supervised machine learning techniques: An overview. In 2020 IEEE Asia-Pacific Conference on Computer Science and Data Engineering (CSDE) (pp. 1-6). IEEE.
[3]. Yahoo Finance. Available at: https://finance.yahoo.com (Accessed on 05 November 2023).
[4]. Kumbure, M. M., Lohrmann, C., Luukka, P., & Porras, J. (2022). Machine learning techniques and data for stock market forecasting: A literature review. Expert Systems with Applications, 197, 116659.
[5]. Zhong, X., & Enke, D. (2019). Predicting the daily return direction of the stock market using hybrid machine learning algorithms. Financial Innovation, 5(1), 1-20.
[6]. Kumar, L., Pandey, A., Srivastava, S., & Darbari, M. (2011). A hybrid machine learning system for stock market forecasting. Journal of International Technology and Information Management, 20(1), 3.
[7]. Yu, H., Chen, R., & Zhang, G. (2014). A SVM stock selection model within PCA. Procedia computer science, 31, 406-412.
[8]. Khaidem, L., Saha, S., & Dey, S. R. (2016). Predicting the direction of stock market prices using random forest. arXiv preprint arXiv:1605.00003.
[9]. Polamuri, S. R., Srinivas, K., & Mohan, A. K. (2019). Stock market prices prediction using random forest and extra tree regression. Int. J. Recent Technol. Eng, 8(1), 1224-1228.
[10]. Wang, H., & Hu, D. (2005, October). Comparison of SVM and LS-SVM for regression. In 2005 International conference on neural networks and brain (Vol. 1, pp. 279-283). IEEE.
Cite this article
Li,J. (2024). Predict Amazon stock by SVM and Random Forest. Applied and Computational Engineering,46,283-289.
Data availability
The datasets used and/or analyzed during the current study will be available from the authors upon reasonable request.
Disclaimer/Publisher's Note
The statements, opinions and data contained in all publications are solely those of the individual author(s) and contributor(s) and not of EWA Publishing and/or the editor(s). EWA Publishing and/or the editor(s) disclaim responsibility for any injury to people or property resulting from any ideas, methods, instructions or products referred to in the content.
About volume
Volume title: Proceedings of the 4th International Conference on Signal Processing and Machine Learning
© 2024 by the author(s). Licensee EWA Publishing, Oxford, UK. This article is an open access article distributed under the terms and
conditions of the Creative Commons Attribution (CC BY) license. Authors who
publish this series agree to the following terms:
1. Authors retain copyright and grant the series right of first publication with the work simultaneously licensed under a Creative Commons
Attribution License that allows others to share the work with an acknowledgment of the work's authorship and initial publication in this
series.
2. Authors are able to enter into separate, additional contractual arrangements for the non-exclusive distribution of the series's published
version of the work (e.g., post it to an institutional repository or publish it in a book), with an acknowledgment of its initial
publication in this series.
3. Authors are permitted and encouraged to post their work online (e.g., in institutional repositories or on their website) prior to and
during the submission process, as it can lead to productive exchanges, as well as earlier and greater citation of published work (See
Open access policy for details).