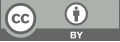
Real-time face recognition method based on MTCNN-Inception-ResNet-v2-SVM model
- 1 Southern University of Science and Technology
* Author to whom correspondence should be addressed.
Abstract
With the widespread use of smart devices such as smartphones, facial recognition applications have experienced rapid growth. Additionally, breakthroughs in deep learning algorithms have led to the development of facial recognition technology based on deep convolutional networks, greatly improving recognition speed and accuracy. Therefore, this study proposes a real-time face recognition method based on the MTCNN-Inception-ResNet-v2-SVM model. In the facial detection phase, the MTCNN algorithm, which offers better overall performance compared to face detection algorithms such as OpenCV and Dlib, is utilized. Data augmentation and other methods are employed in the image preprocessing phase to enhance data diversity. The Inception-ResNet-v2 deep convolutional neural network is used as the backbone network for feature extraction in the facial recognition backbone network section. In the final classification stage, an SVM classifier is employed for the ultimate facial classification. Comparative analyses with models such as Inception-ResNet-v1, Inception-v3, and Inception-v4 are conducted in the backbone network section, determining that the Inception-ResNet-v2 model exhibits the best overall performance. The final result is a real-time face recognition model, MTCNN-Inception-ResNet-v2-SVM, with a high accuracy of 98.79% and fast processing speed.
Keywords
Deep Learning, Facial Recognition, Convolutional Neural Network, MTCNN, Inception-ResNet-v2
[1]. Turk, M., & Pentland, A. (1991). Eigenfaces for recognition. Journal of cognitive neuroscience, 3(1), 71-86.
[2]. Ojala, T., Pietikainen, M., & Maenpaa, T. (2002). Multiresolution gray-scale and rotation invariant texture classification with local binary patterns. IEEE Transactions on pattern analysis and machine intelligence, 24(7), 971-987.
[3]. Turk, M. A., & Pentland, A. P. (1991, January). Face recognition using eigenfaces. In Proceedings. 1991 IEEE computer society conference on computer vision and pattern recognition (pp. 586-587). IEEE Computer Society.
[4]. Schroff, F., Kalenichenko, D., & Philbin, J. (2015). Facenet: A unified embedding for face recognition and clustering. In Proceedings of the IEEE conference on computer vision and pattern recognition (pp. 815-823).
[5]. Szegedy, C., Ioffe, S., Vanhoucke, V., & Alemi, A. (2017, February). Inception-v4, inception-resnet and the impact of residual connections on learning. In Proceedings of the AAAI conference on artificial intelligence (Vol. 31, No. 1).
[6]. He, K., Zhang, X., Ren, S., & Sun, J. (2016). Identity mappings in deep residual networks. In Computer Vision–ECCV 2016: 14th European Conference, Amsterdam, The Netherlands, October 11–14, 2016, Proceedings, Part IV 14 (pp. 630-645). Springer International Publishing.
[7]. Wen, Y., Zhang, K., Li, Z., & Qiao, Y. (2016). A discriminative feature learning approach for deep face recognition. In Computer Vision–ECCV 2016: 14th European Conference, Amsterdam, The Netherlands, October 11–14, 2016, Proceedings, Part VII 14 (pp. 499-515). Springer International Publishing.
[8]. Szegedy, C., Liu, W., Jia, Y., Sermanet, P., Reed, S., Anguelov, D., ... & Rabinovich, A. (2015). Going deeper with convolutions. In Proceedings of the IEEE conference on computer vision and pattern recognition (pp. 1-9).
[9]. Zeiler, M. D., & Fergus, R. (2014). Visualizing and understanding convolutional networks. In Computer Vision–ECCV 2014: 13th European Conference, Zurich, Switzerland, September 6-12, 2014, Proceedings, Part I 13 (pp. 818-833). Springer International Publishing.
[10]. Zhang, K., Zhang, Z., Li, Z., & Qiao, Y. (2016). Joint face detection and alignment using multitask cascaded convolutional networks. IEEE signal processing letters, 23(10), 1499-1503.
[11]. Viola, P., & Jones, M. J. (2004). Robust real-time face detection. International journal of computer vision, 57, 137-154.
[12]. Dalal, N., & Triggs, B. (2005, June). Histograms of oriented gradients for human detection. In 2005 IEEE computer society conference on computer vision and pattern recognition (CVPR'05) (Vol. 1, pp. 886-893). IEEE.
[13]. Neubeck, A., & Van Gool, L. (2006, August). Efficient non-maximum suppression. In 18th international conference on pattern recognition (ICPR'06) (Vol. 3, pp. 850-855). IEEE.
[14]. He, K., Zhang, X., Ren, S., & Sun, J. (2016). Deep residual learning for image recognition. In Proceedings of the IEEE conference on computer vision and pattern recognition (pp. 770-778).
[15]. Yi, D., Lei, Z., Liao, S., & Li, S. Z. (2014). Learning face representation from scratch. arXiv preprint arXiv:1411.7923.
[16]. Huang, G. B., Mattar, M., Berg, T., & Learned-Miller, E. (2008, October). Labeled faces in the wild: A database forstudying face recognition in unconstrained environments. In Workshop on faces in'Real-Life'Images: detection, alignment, and recognition.
Cite this article
Zhang,H. (2024). Real-time face recognition method based on MTCNN-Inception-ResNet-v2-SVM model. Applied and Computational Engineering,45,179-189.
Data availability
The datasets used and/or analyzed during the current study will be available from the authors upon reasonable request.
Disclaimer/Publisher's Note
The statements, opinions and data contained in all publications are solely those of the individual author(s) and contributor(s) and not of EWA Publishing and/or the editor(s). EWA Publishing and/or the editor(s) disclaim responsibility for any injury to people or property resulting from any ideas, methods, instructions or products referred to in the content.
About volume
Volume title: Proceedings of the 4th International Conference on Signal Processing and Machine Learning
© 2024 by the author(s). Licensee EWA Publishing, Oxford, UK. This article is an open access article distributed under the terms and
conditions of the Creative Commons Attribution (CC BY) license. Authors who
publish this series agree to the following terms:
1. Authors retain copyright and grant the series right of first publication with the work simultaneously licensed under a Creative Commons
Attribution License that allows others to share the work with an acknowledgment of the work's authorship and initial publication in this
series.
2. Authors are able to enter into separate, additional contractual arrangements for the non-exclusive distribution of the series's published
version of the work (e.g., post it to an institutional repository or publish it in a book), with an acknowledgment of its initial
publication in this series.
3. Authors are permitted and encouraged to post their work online (e.g., in institutional repositories or on their website) prior to and
during the submission process, as it can lead to productive exchanges, as well as earlier and greater citation of published work (See
Open access policy for details).