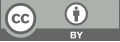
Continuous trading strategy based on deep reinforcement learning
- 1 Guangdong University of Foreign Studies
* Author to whom correspondence should be addressed.
Abstract
Automatic trading policy has been researched with reinforcement learning (RL). Designing a profitable and applicable policy is of great significance for research in quantitative finance. Incoprating with deep learning, typical deep reinforcement learning (DRL) algorithms such as Proximal Policy Optimization (PPO) have shown their effectiveness. To improve the practical applicability, the manner in which we train our model should better simulate the dynamic of the stock market. A sliding window training strategy is a better solution, which employes training, validation and trading procedures on dataset with a sliding window. However, this empirical strategy can still be further investigated in algorithm evaluation and the experiment designation such as choice of sliding window. In this paper, we further investigated the continuous trading strategy (CTS). We evaluated the performance of a wider range of algorithms, including PPO, Deep Deterministic Policy Gradient (DDPG), Advantage Actor-Critic (A2C), Soft Actor-Critic (SAC). Moreover, we trained our models on a longer term period. We also provide detailed observations and suggestions on experiment settings. These discussions will facilitate researchers in their future work.
Keywords
Financial Technology, Quantitative Finance, Stock Trading, Reinforcement Learning, Deep Learning
[1]. Xiong Z, Liu X-Y, Zhong S, Yang H, Elwalid A. Practical Deep Reinforcement Learning Approach for Stock Trading. arXiv preprint arXiv:181107522. 2018:1-7.
[2]. Liu X-Y, Xia Z. Empirical Demonstration of Stock Paper Trading for Financial Reinforcement Learning. Available at SSRN 4253133. 2022.
[3]. Schulman J, Wolski F, Dhariwal P, Radford A, Klimov O. Proximal policy optimization algorithms. arXiv preprint arXiv:170706347. 2017.
[4]. Lillicrap TP, Hunt JJ, Pritzel A, Heess N, Erez T, Tassa Y, et al. Continuous control with deep reinforcement learning. arXiv preprint arXiv:150902971. 2015.
[5]. Mnih V, Badia AP, Mirza M, Graves A, Lillicrap T, Harley T, et al., editors. Asynchronous methods for deep reinforcement learning. International conference on machine learning; 2016: PMLR.
[6]. Schulman J, Moritz P, Levine S, Jordan M, Abbeel P. High-dimensional continuous control using generalized advantage estimation. arXiv preprint arXiv:150602438. 2015.
[7]. Schulman J, Levine S, Abbeel P, Jordan M, Moritz P, editors. Trust region policy optimization. International conference on machine learning; 2015: PMLR.
[8]. Jiang Z, Xu D, Liang J. A Deep Reinforcement Learning Framework for the Financial Portfolio Management Problem. 2017.
[9]. Liang Z, Chen H, Zhu J, Jiang K, Li Y. Adversarial Deep Reinforcement Learning in Portfolio Management. arXiv preprint arXiv:180809940. 2018.
[10]. Guan M, Liu X-Y. Explainable deep reinforcement learning for portfolio management. Proceedings of the Second ACM International Conference on AI in Finance2021. p. 1-9.
[11]. Zhang Z, Zohren S, Stephen R. Deep reinforcement learning for trading. The Journal of Financial Data Science. 2020.
[12]. Yang H, Liu X-Y, Zhong S, Walid A, editors. Deep reinforcement learning for automated stock trading: An ensemble strategy. Proceedings of the first ACM international conference on AI in finance; 2020.
Cite this article
Zhang,K. (2024). Continuous trading strategy based on deep reinforcement learning. Applied and Computational Engineering,57,233-240.
Data availability
The datasets used and/or analyzed during the current study will be available from the authors upon reasonable request.
Disclaimer/Publisher's Note
The statements, opinions and data contained in all publications are solely those of the individual author(s) and contributor(s) and not of EWA Publishing and/or the editor(s). EWA Publishing and/or the editor(s) disclaim responsibility for any injury to people or property resulting from any ideas, methods, instructions or products referred to in the content.
About volume
Volume title: Proceedings of the 6th International Conference on Computing and Data Science
© 2024 by the author(s). Licensee EWA Publishing, Oxford, UK. This article is an open access article distributed under the terms and
conditions of the Creative Commons Attribution (CC BY) license. Authors who
publish this series agree to the following terms:
1. Authors retain copyright and grant the series right of first publication with the work simultaneously licensed under a Creative Commons
Attribution License that allows others to share the work with an acknowledgment of the work's authorship and initial publication in this
series.
2. Authors are able to enter into separate, additional contractual arrangements for the non-exclusive distribution of the series's published
version of the work (e.g., post it to an institutional repository or publish it in a book), with an acknowledgment of its initial
publication in this series.
3. Authors are permitted and encouraged to post their work online (e.g., in institutional repositories or on their website) prior to and
during the submission process, as it can lead to productive exchanges, as well as earlier and greater citation of published work (See
Open access policy for details).